Chapter1 PART 1 - Basics & Fundamentals
1.1 Programming and Remote Sensing Basics
1.1.1 Remote Sensing Language
A) Definition
The term Remote sensing has been variously defined. Some of its early definitions include:
The art or science of telling something about an object without touching it. (Fischer et al., 1976)
Remote sensing is the acquisition of physical data of an object without touch or contact. (Lintz and Simonett, 1976)
Remote sensing is the observation of a target by a device separated from it by some distance. (Barrett and Curtis, 1976)
The term remote sensing in its broadest sense means “reconnaissance at a distance.” (Colwell, 1966)
Thus, in the context of this training, we can define Remote Sensing as the science of acquiring information about a given target, object or phenomenon on the surface of the Earth by sensors on-board various platforms orbiting our planet.
B) Building blocks of remote sensing
Although the many different methods for collection, processing and interpretation of remotely sense data can vary widely, they will always have the following essential components:
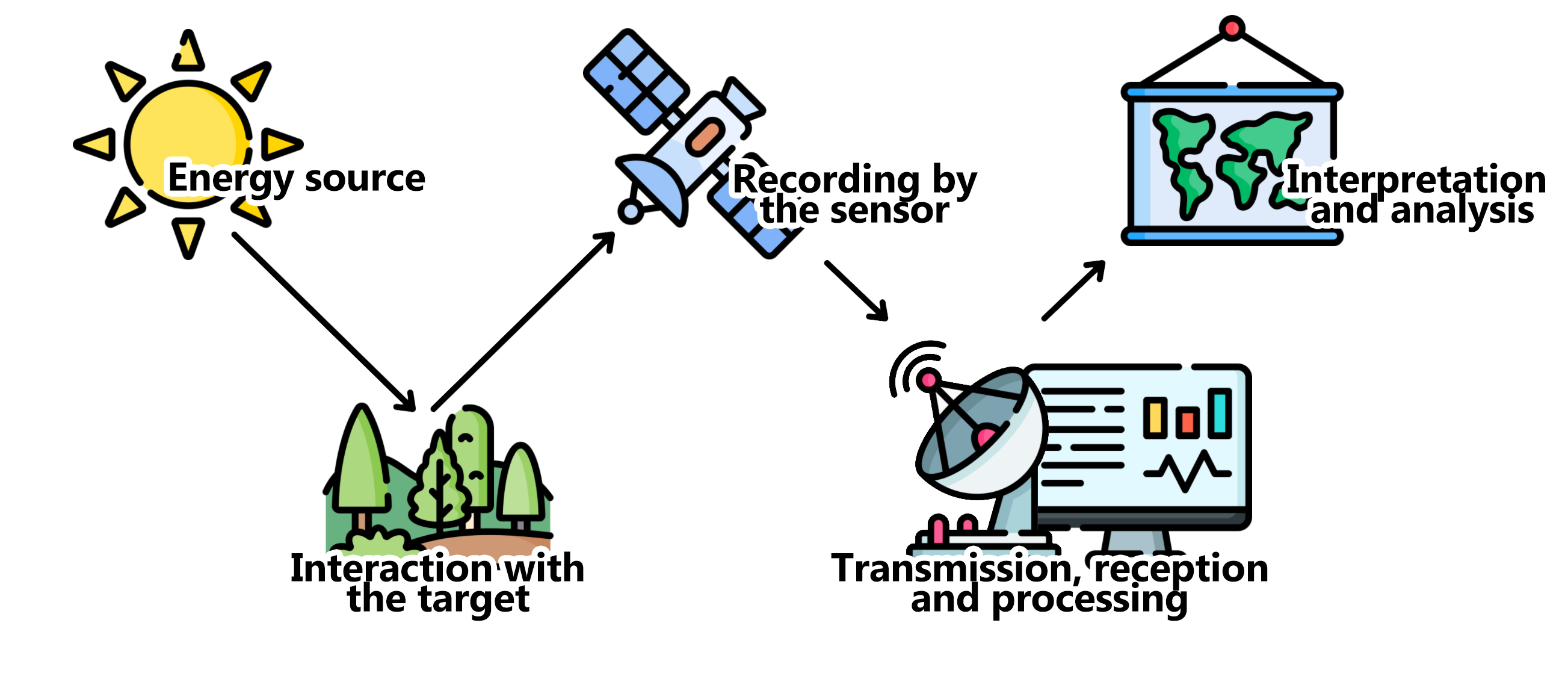
Figure 1.1: Basic components of a remote sensing system.
- Energy Source
The source of the electromagnetic radiation/energy (EMR) is the first requirement of any remote sensing process. The electromagnetic spectrum is used to “classify” the EMR according to its wavelength:
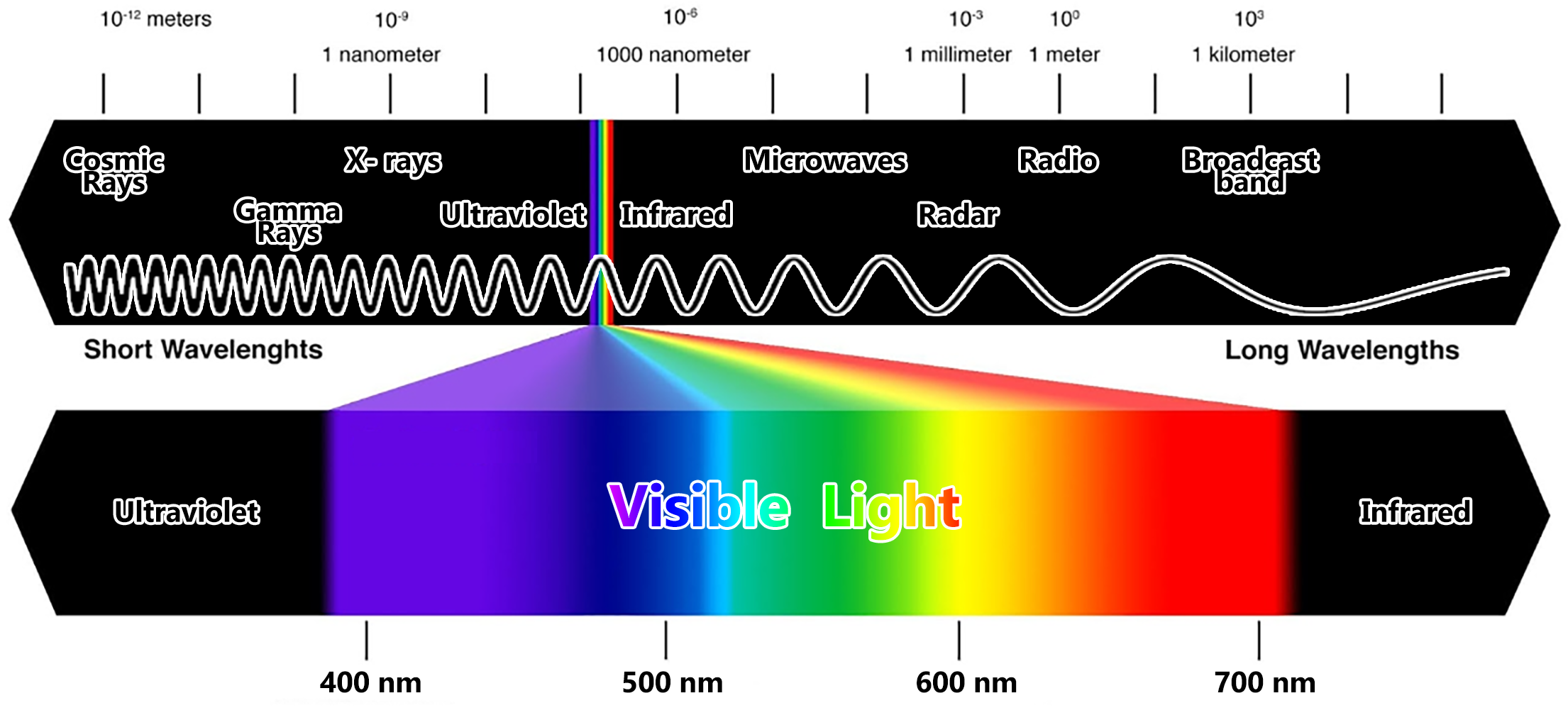
Figure 1.2: The Electromagnetic Spectrum
Depending on the source of energy they are using, the different remote sensing systems can be classified as active or passive. Active sensors will produce its own source of energy for the illumination of the target. They will emit the energy toward the target being investigated and the energy reflected by this target is detected and measured by the sensor. Usually, these sensors operate in the microwave range of the electromagnetic spectrum. On the other hand, passive sensors only measures the energy that is naturally available, usually from the sun. These sensors can only be used to detect the energy being reflected during the time when the sun is illuminating the Earth. These sensors usually measure energy from the optical range (visible, near infrared, short-wave infrared and thermal infrared).
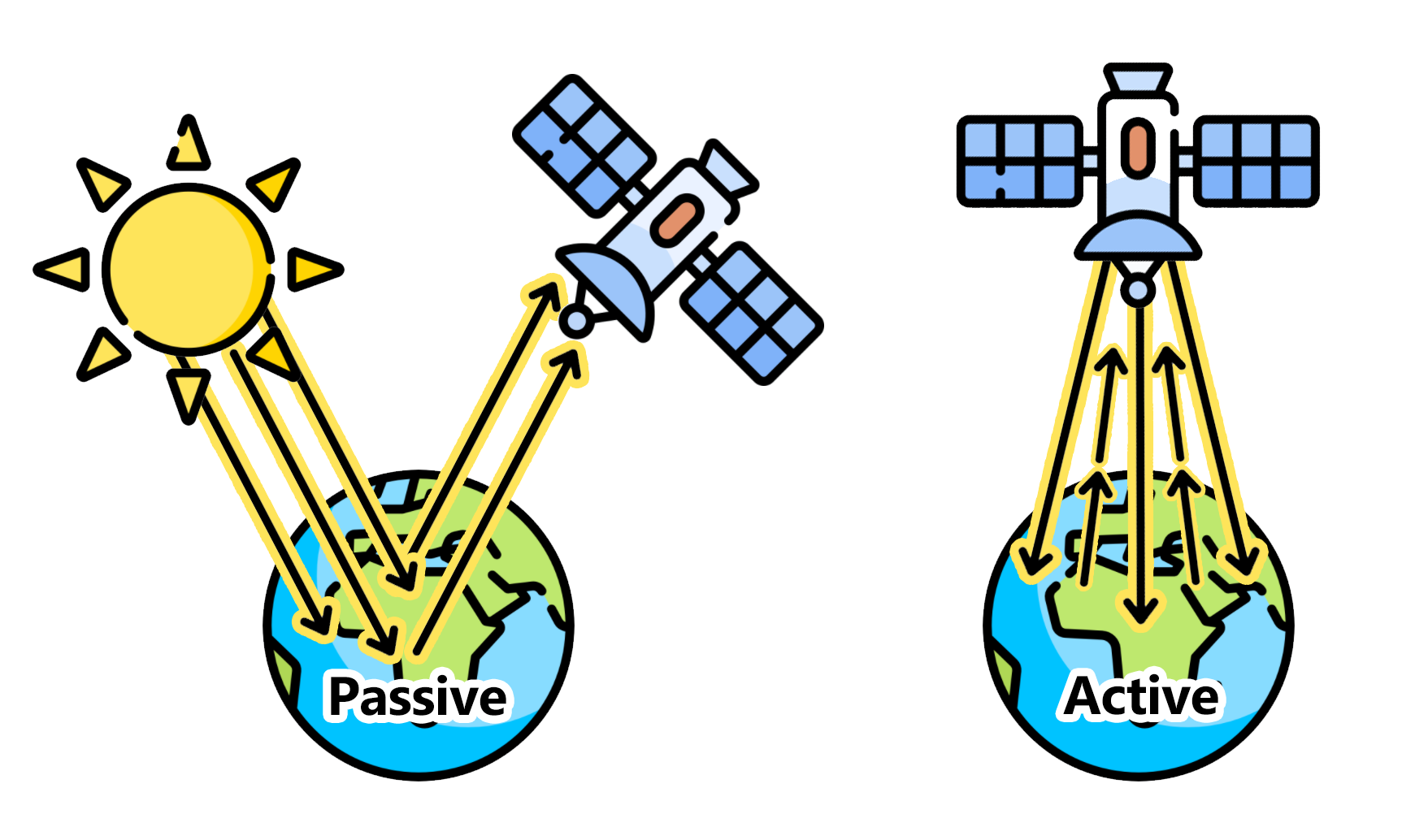
Figure 1.3: Remote sensing can be classified as Passive or Active based on the source of energy.
You can also think about these concepts of active and passive using a handheld photographic camera as an example: When photographing a target in the dark, the camera flash will provide the energy necessary to illuminate the target. Therefore, in that case, the camera is an active sensor. On the other hand, this same camera will be a passive sensor when you are photographing a target or object during the day, when the target being illuminated by sun light and no flash is necessary.
- Interaction with the target/object
The most common medium in between the source and target is the atmosphere. This is where the first interaction occurs. As the EMR travel from its source to the target, it will come in contact with and interact different atmosphere constituents: aerosols, water vapor, solid particles, etc. Secondly, once the EMR makes its way through the atmospherethe to the target, it will interact with it depending on the target properties and energy wavelength. The EMR can have different types of interaction when it encounters matter; whether it is gas, solid or liquid: it can be transmitted (that is, it passes through the target), absorbed (that is, the target absorbs the energy usually increasing its temperature as a result), emitted (that is, energy is emitted from all matter at temperatures above the absolute zero of 0 Kelvins), scattered (that is, deflected in every direction) and reflected (that is, energy bounces off the target’s surface and its direction is usually a function of target structure and texture).
Keep in mind: All targets can show different proportions of each of these interactions.
- Recording of the energy by the sensor
The sensor - often onboard of airplanes or satellites in space - will measure the returning EMR after it has interacted with the target and the atmosphere. This measurement is converted into a digital image with discrete values in units of digital number (DN) for each image pixel. Depending on the sensor, these resulting images will have different characteristics (or resolutions). They are:
Spatial Resolution: usually known as pixel size. It refers to the sensor’s ability to discriminate different objects/targets. A higher spatial resolution means a smaller pixel size which, in turn, means that smaller objects can be distinguishable as separate targets.
Spectral Resolution: Different sensors will measure the EMR at specific ranges (or wavelengths), usually called bands. Thus, the spectral resolution of a sensor usually refers to the number and bandwith of these bands.
Radiometric Resolution: Usually measured in bits, it refers to the sensor’s ability to detect the smallest change in the spectral reflectance among different targets. For example, a 8-bit image will have 256 levels of brightness while a 16-bit image has 65,536 levels of brightness.
Temporal resolution (sensors onboard satellites): is the time required for the satellite to collect two images at the same geographic location on Earth. Higher temporal resolution means less time for revisiting the same location. However, temporal resolution is usually inverselly proportional to spatial resolution: The larger the pixel size, the larger area the sensor will cover which means less time until the next revisit.
- Transmission, Reception, and Processing
The EMR recorded by the sensor is transmitted in an electronic form to a receiving station on Earth where the data is processed and stored.
- Analysis and Interpretation (we are here!)
This is where this training is focused on! The EMR was transformed into a digital dataset where we can use specialized instruments/hardware/software to extract information about the target observed. This extraction is often done through image processing (or digital image processing), which is the process which makes an image interpretable for a given use. There are many methods of image processing, but these are the most common ones:
Image correction: The digital image recorded by the sensor on a satellite (or aircraft) may contain errors related to the geometry and brightness values of the pixels. For example, a geometrical correction, also called geo-referencing, is a procedure where the content of image will be assigned a spatial coordinate system (for example, geographical latitude and longitude).
Image enhancement: This is related to modification of an image, by changing the pixel brightness values, to improve its visual aspects so that the actual analysis of images will be easier, faster and more reliable.
Image classification: The overall goal of this method is to categorize all pixels in an image into themes (or land cover classes). This resulting map with its limited number of classes can be more readily and sucessfully interpreted compared to the raw image and it is often use for planning purposes. There are supervised and unsupervised methods for classification of an image: A supervised classification (human-guided) is based on the idea that a user can select sample pixels in an image that are representative of specific classes and then direct the image processing software to use these training sites as references for the classification of all other pixels in the image. These samples are selected based on the knowledge of the user. On the other hand, an unsupervised classification (computer/software-guided) is where the output classes are based on the software’s ability to determine which pixels are related, using several different models and techniques.
This final component of Remote Sensing (V) is achieved when we apply the extracted information to solve a particular problem.
C) Spectral Signatures: A target’s spectral fingerprint
As mentioned before, remote sensing is based on the measurement of reflected (or emitted) radiation from different targets. Objects having different surface features reflect or absorb the sun’s radiation in different ways. In order to understand and interpret the information extracted from remotely sensed data, you have to first understand the behavior of the target in respect to the electromagnetic spectrum. Each target will show a distinct reflectance pattern as a function of the wavelength, known as spectral signature (or a spectral fingerprint). This signature will directly (or indirectly) lead to the identification of a target based on its set of values for its reflectance in different spectral ranges:
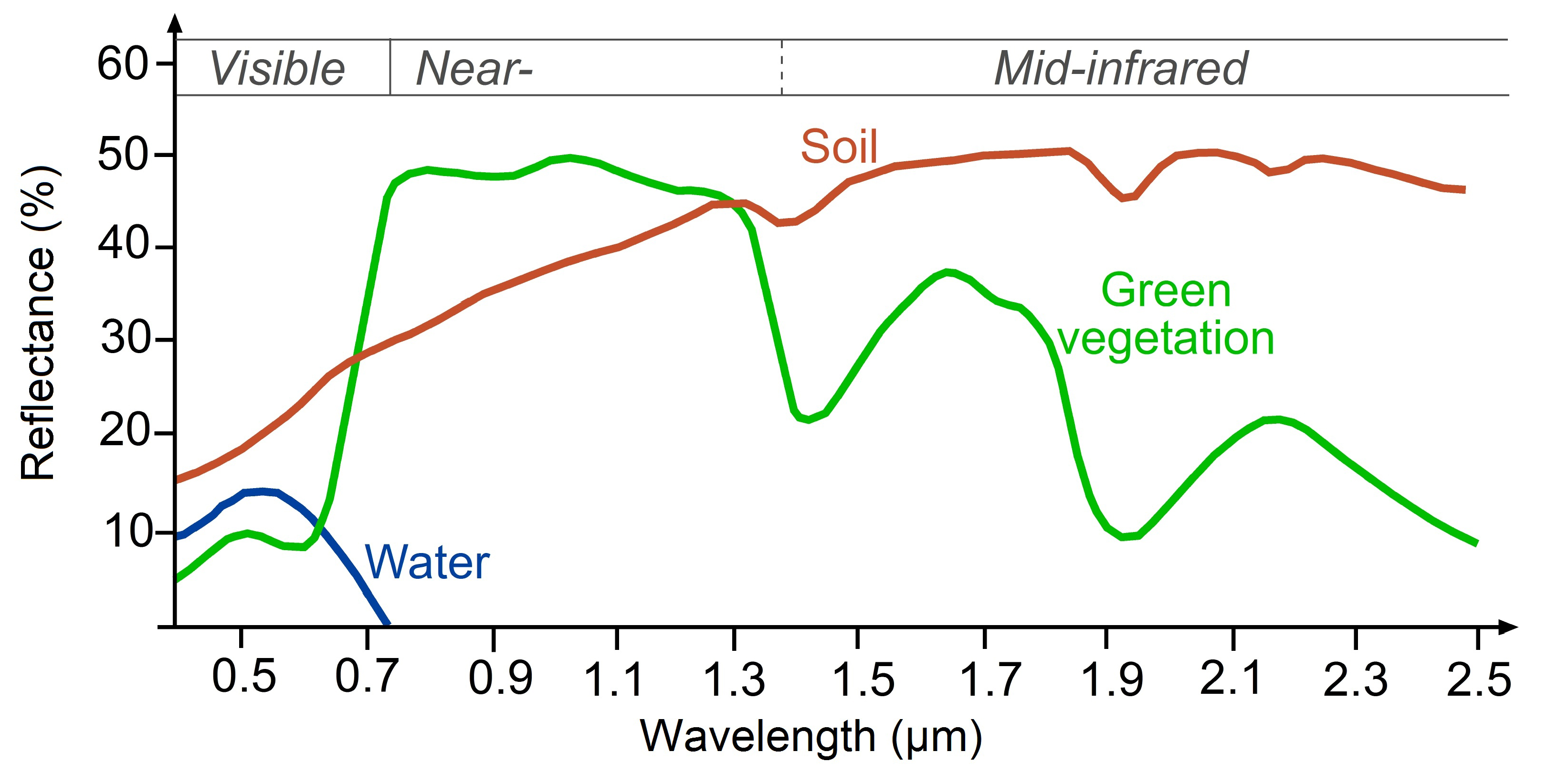
Figure 1.4: Typical spectral signatures of specific land cover types in the visible and infrared region of the electromagnetic spectrum (Source: http://www.seos-project.eu/)
The spectral signature of healthy green vegetation has a small reflectance in the visible portion of the electromagnetic spectrum resulting from the pigments in plant leaves. Most of the light is being used in the photosynthesis process. However, the reflectance increases dramatically in the near infrared. The spectral signature of soil is much less variable. Its behavior is affected by soil moisture, texture, surface roughness and they are less dominant than the absorbance features present in vegetation. The water’s spectral signature is characterized by a high absorption at near infrared wavelengths range and beyond. Because of this absorption property, water bodies as well as features containing water can easily be detected, located and delineated with remote sensing data.
These differences make it possible to identify different Earth surface features or materials by analysing their spectral reflectance patterns or spectral signatures. [add more text]
References
Fischer, W. A., W.R. Hemphill and A. Kover. 1976. Progress in Remote Sensing. Photogrametria, Vol. 32, pp. 33-72
Lintz, J. and D. S. Simonett. 1976. Remote Sensing of Environment. Reading, MA: Addison-Wesley. 694 pp.
Barrett, E. C. and C. F. Curtis. 1976. Introduction to Environmental Remote Sensing. New York: Macmillian, 472 pp.
Colwell, R. N. 1966. Uses and Limitations of Multispectral Remote Sensing. In Proceedings of the Fourth Symposium on Remote Sensing of Environment. Ann Arbor: Institute of Science and Technology, University of Michigan, pp. 71-100.
1.1.2 Google Earth Engine’s Application Programming Interface (API) and Java Script
Google Earth Engine is a cloud-based platform for scientific data analysis and remote sensing data processing. It provides a large catalog of ready-to-use, cloud-hosted datasets. One of Earth Engine’s key features is the ability to handle computationally demading processing and analysis very fast by distributing them across a large number of servers. The ability to efficiently use the cloud-hosted datasets and computation is enabled by the Earth Engine API.
An API is a way to communicate with Google Earth Engine servers. It allows you to specify what computation or command you would like to do, and then to receive the results back from the servers. The API is designed so that users do not need to worry about how the computation is distributed across a cluster of machines and the results are assembled. Users of the API simply specify what needs to be done. This greatly simplifies the code by hiding the implementation detail from the users. It also makes Earth Engine simpler for users who are not too familiar with writing code.
JavaScript API and Introduction to the Code Editor
The Earth Engine platform comes with a web-based Code Editor that allows you to start using the Earth Engine JavaScript API without any installation. It also provides additional functionality to display your results on a map, save your scripts, access documentation, manage tasks, and more. It has a one-click mechanism to share your code with other users—allowing for easy reproducibility and collaboration. In addition, the JavaScript API comes with a user interface library, which allows you to create charts and web-based applications with little effort.
The Code Editor is an integrated development environment for the Earth Engine JavaScript API. It offers an easy way to type, debug, run, and manage code. Once you have successfully registered for a Google Earth Engine account, you can visit https://code.earthengine.google.com/ to open the Code Editor. When you first visit the Code Editor, you will see a screen such as the one shown below:
Figure 1.5: Earth Engine Code Editor
The Code Editor allows you to type JavaScript code and execute it. When you are first learning a new language and getting used to a new programming environment, it is customary to make a program to display your name or the words “Hello World.” This is a fun way to start coding that shows you how to give input to the program and how to execute it. It also shows where the program displays the output. Doing this in JavaScript is quite simple. Copy the following code into the center panel:
The line of code above uses the JavaScript print()
function to print the text “Hello World” to the screen. Once you enter the code, click the Run button. The output will be displayed on the upper right-hand panel under the Console tab:
Figure 1.6: Running code with GEE
You now know where to type your code, how to run it, and where to look for the output. You just wrote your first Earth Engine script and may want to save it. Click the Save button to save a script:
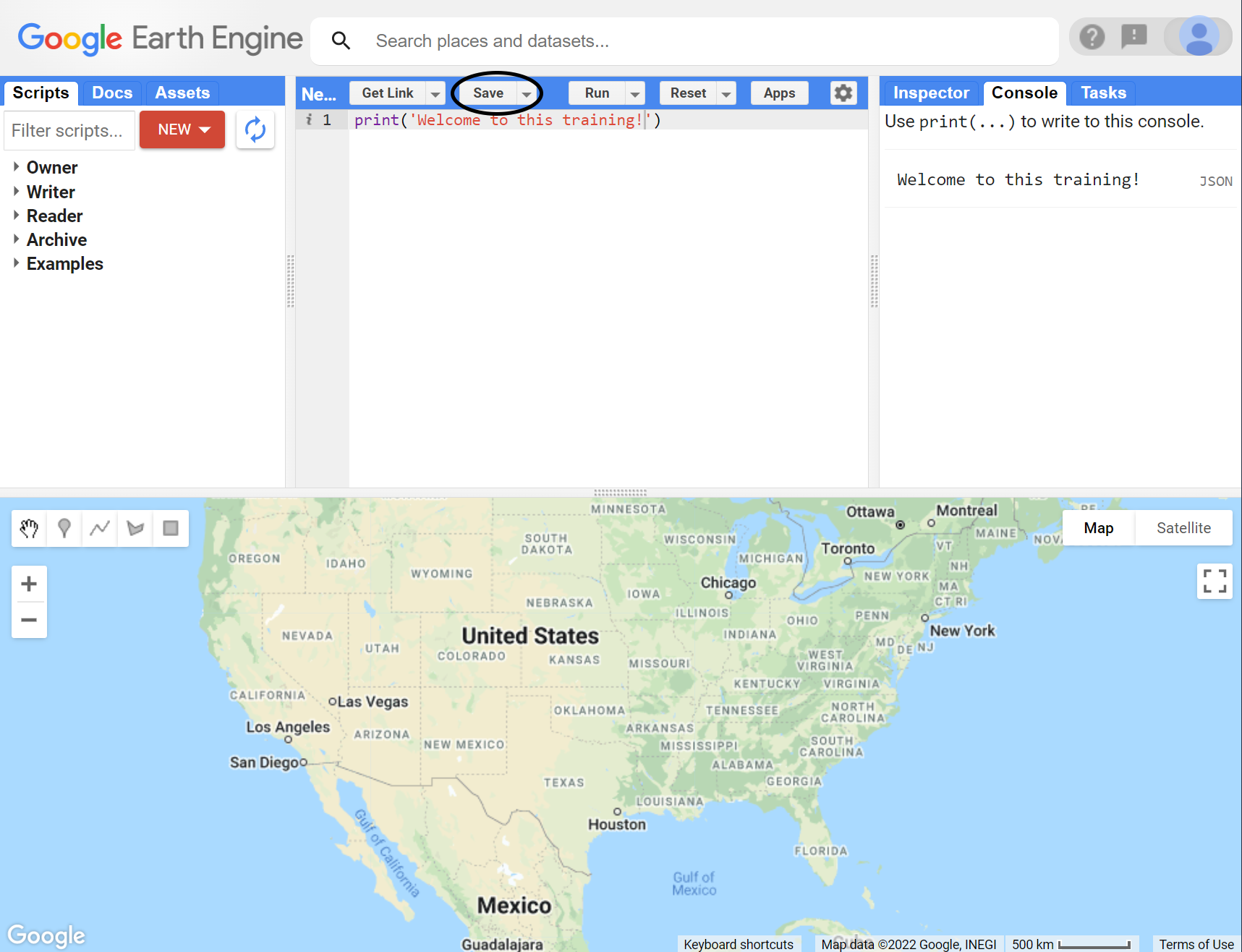
Figure 1.7: Saving a script
If this is your first time using the Code Editor, you will be prompted to create a home folder. This is a folder in the cloud where all your code will be saved:
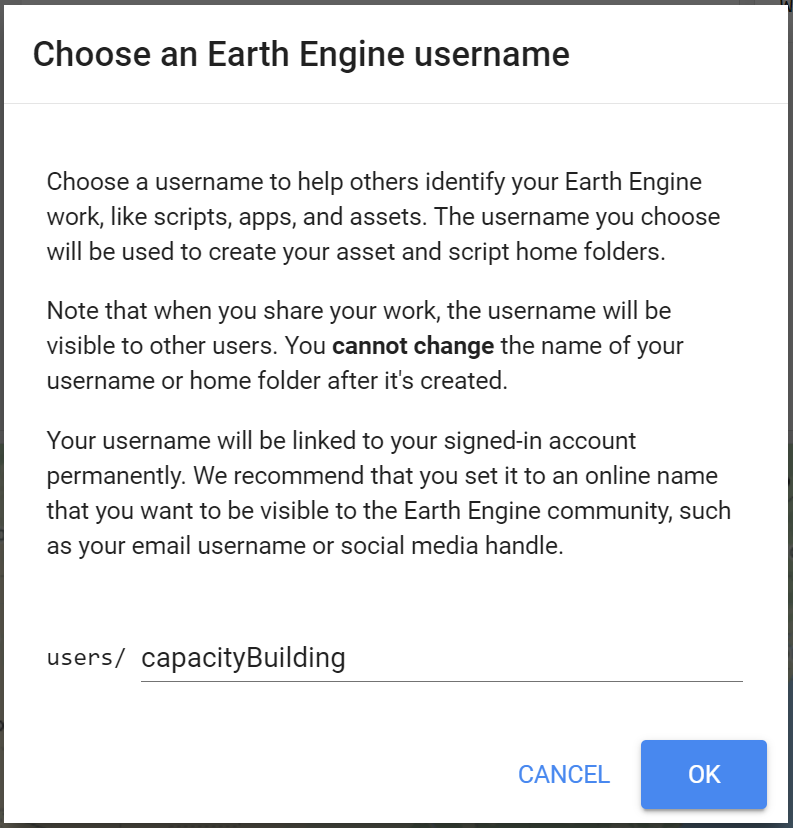
Figure 1.8: Your first home folder
You can pick a name of your choice, but remember that it cannot be changed and will permanently be associated with your account.
Once youre home folder is created, you will be prompted to create a new repository. A repository is a like a folder where you can save your scripts. You can also share entire repositories with other users. Your account can have multiple repositories and each one can hold multiple code scripts. Start by creating a repository:
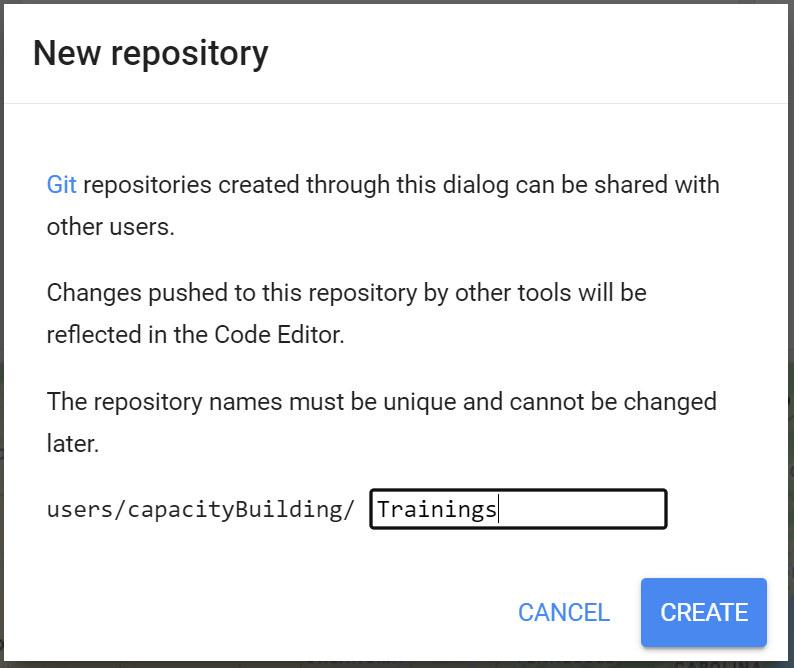
Figure 1.9: Your first repository
Finally, you will be able to save your script inside the newly created repository. Enter a name of your choice and click OK:
Spaces are not allowed when naming scripts!
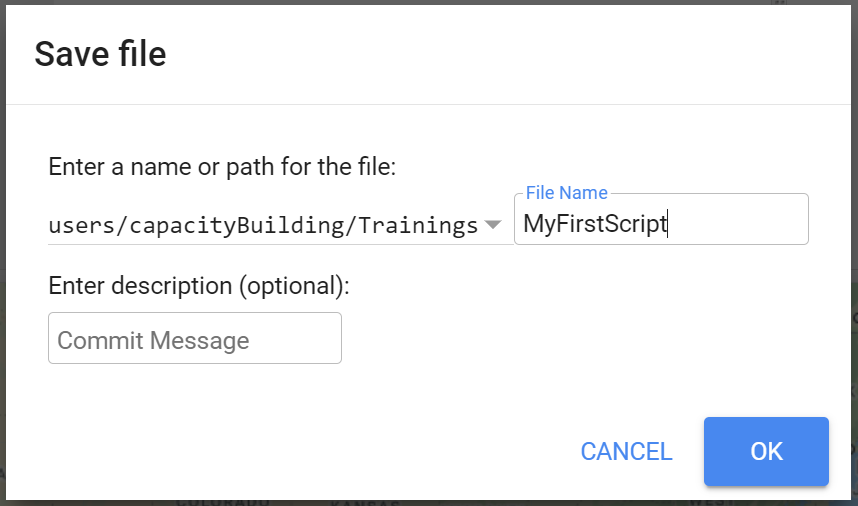
Figure 1.10: Saving a script file
Once the script is saved, it will appear in the script manager panel. The scripts are saved in the cloud and will always be available to you when you open the Code Editor.
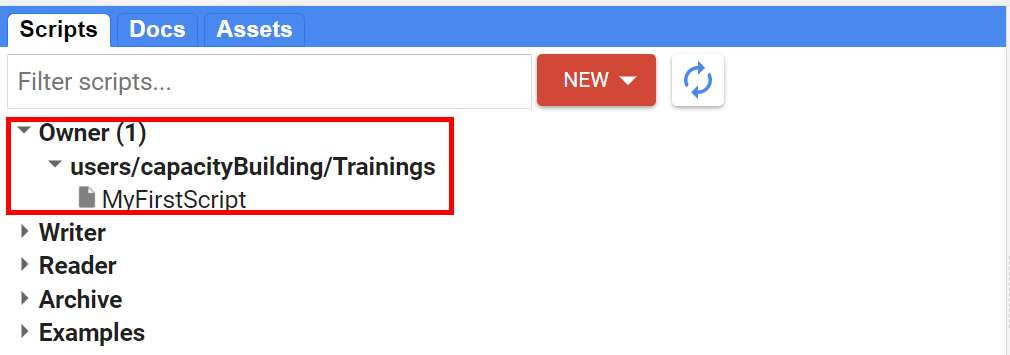
Figure 1.11: Your first script in your repository
JavaScript Basics: Data types
Javascript is the language you will use to construct and set up your commands and analysis. This section covers the basics of the Java Script sintax and some basic data structures. In the following sections, more JavaScript code will be presented. Throughout this document, code will be presented with a distinct colored font and with shaded background. As you encounter code, copy and paste it into the Code Editor and click Run.
A) Variables
In a programming language, variables are used to store data values. In JavaScript, a variable is defined using the var
keyword followed by the name of the variable. For instance, create a var
named city
that contain the text string 'Monrovia'
. Text strings in the code must be always in quotes. Google Earth Engine allows you to use single '
or double "
quotes, as long as they match in the beginning and end of the text string. We also usually end each statement on scripts with a semicolon ;
, although Earth Engine’s code editor does not require it. A ‘i’ will be shown in the line of the code where a semicolon is missing:

Figure 1.12: Even though they are not a requirement, Google Earth Engine indicates that a semicolon is missing in the statement. You can hover the mouse cursor over the icon to reveal its meaning.
If you print
the variable city
, you will get the string stored in the variable (Monrovia) printed in the Console.
When you use quotations, the variable is automatically assigned the type string. You can also assign numbers to variables. For example, create the variables population
and area
and assing a number as their value. When assigning numbers, you do not use commas ,
for thousand separators. You do, however, use .
for decimals:
Print those variables to the Console. You can also add text to describe the variables printed in the Console. Simply add a text string within the print
function along with the variable being printed separated with ,
. Use the code below as example:
B) Lists
In the previous examples, we created variables holding a single value (text or number). JavaScript provides a data structure called a list that can be used When you want to store multiple values in a single variable. You can create lists using square brackets []
and adding multiple values separated by ,
. Create a variable called listofcities
, add values to it and print it to the Console:
var listofcities = ['Monrovia', 'Gbarnga','Kakata', 'Bensonville'];
print('Largest cities in Liberia', listofcities);
Looking at the output in the Console, you will see listofcities
with an expander arrow (▹) next to it. Expand the list by clicking on the arrow to show its content. You will notice that along with the items on the list, there will be a number next to each value you added. This is the index of each item. It allows you to refer to each item in the list using a numeric value that indicates its position in the list. This is useful when you want to extract a particular item from a list object.
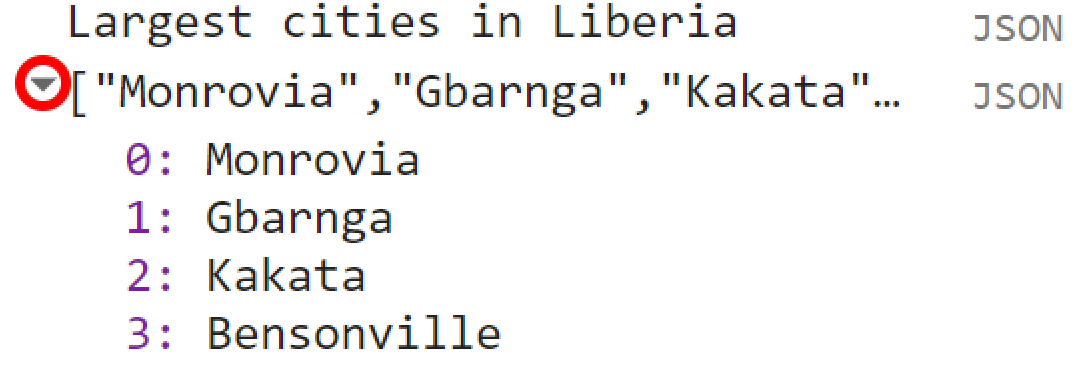
Figure 1.13: A JavaScript List.
C) Objects and Dictionaries
While useful to hold multiple values, lists are not appropriate to hold more structured data. JavaScript allows you to store ‘key-value’ information in objects or dictionaries. In this type of data structure, you can refer to a value by its key rather than its position - like in lists. You can create objects using curly braces {}
. Use the code below as an example of an object:
var cityData = {'city': 'Monrovia', 'population': 939524, 'area': 194.25, 'coordinates': [-10.790, 6.315]};
There are a few important things about the syntax of the code above: As objects tend to hold several keys and values, it can be difficult to read the code if it is written on a continuous string. To improve readability you can use multiple lines instead:
var cityData = {
'city': 'Monrovia',
'population': 939524,
'area': 194.25,
'coordinates': [-10.790, 6.315]
};
Note how each key-value pair is on a different line. It is much easier to organize your key-value information this way. Additionally, the code can be more easily read. Second, note that the object above can holds multiple types of types of values (string and numbers) and structure (list)!
If you print cityData
to the Console, you can see that instead of a numeric index, each value will be identified by its key.
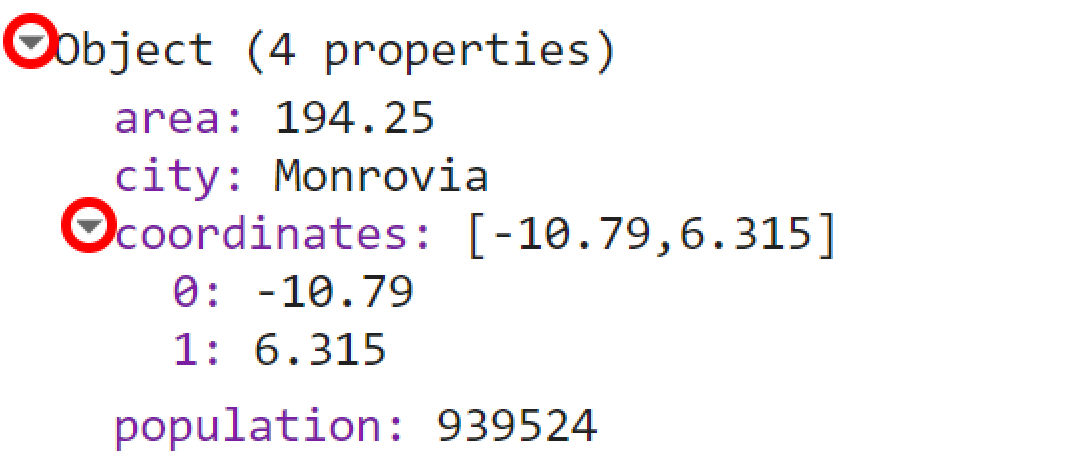
Figure 1.14: A JavaScript Object.
This key can also be used to retrieve the value of an item within an object or dictionary. If you want to retrieve a particular key from a dictionary, simply use ['key']
. For example, if you want to retrieve the population value from this object and print it individually to the Console, you can use a code like this:
The same logic can be applied to lists. Always remember that lists have numeric index. Therefore, when retrieving itens from a list, use the number of its position on the list:
D) Functions
Functions are often used to group a set of operations and used to repeat the same operation with different set of parameters without having to rewrite the code for every iteration. In other words, you can call a function with different parameters to generate different outputs without changing the code.
Functions are defined using function()
. They often take parameters which tell the function what to do. These parameters go inside the parentheses ()
.
Below is an example of a function named SumFunction
to calculate the sum of two numbers: fistValue
and secondValue
. The var sum
adds those two parameters and return
is used to generate the output of that operation
Note that if you run the code above, nothing happens. This is just a function and it needs to be given the parameters. For example, if you need to add 37 to 584 using this function and print the result into the Console, you can use the code below:
When you call SumFunction
, it will always perform the operation sum
with watever two parameters you define in ()
.
As mentioned, you can perform several operations at once using functions. For example, you can add other operations in the function above and return
a list or a dictionary with the results:
function MathFunction (firstValue, secondValue) {
var sum = firstValue + secondValue;
var sub = firstValue - secondValue;
var fraction = firstValue / secondValue;
return {'Result Sum':sum, 'Result Substraction':sub, 'Result Division': fraction};
}
Using MathFunction
with a pair of parameters (firstValue
and SecondValue
) will return an object with the results of each operation:
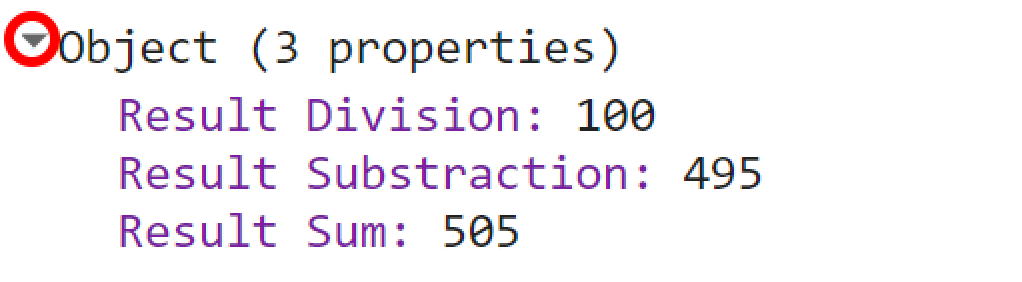
Figure 1.15: Printed results of MathFunction.
Earth Engine Containers and Objects
So far, you learned the different data structure/types you can use within Google Earth Engine. However, if you want to do any computation with the data stored in these different types of structure, you will have to use an Earth Engine container. The ee
package is used for formulating requests to Earth Engine. In other words, ee
(prefix for Earth Engine) allows you to request Earth Engine servers to perform a certain computation to an variable or object. Each ee
object has many different methods. You can think of methods as the many different things and computations that can be done for an specific object. In the Code Editor, you can switch to the Docs tab to see the API functions grouped by object types.
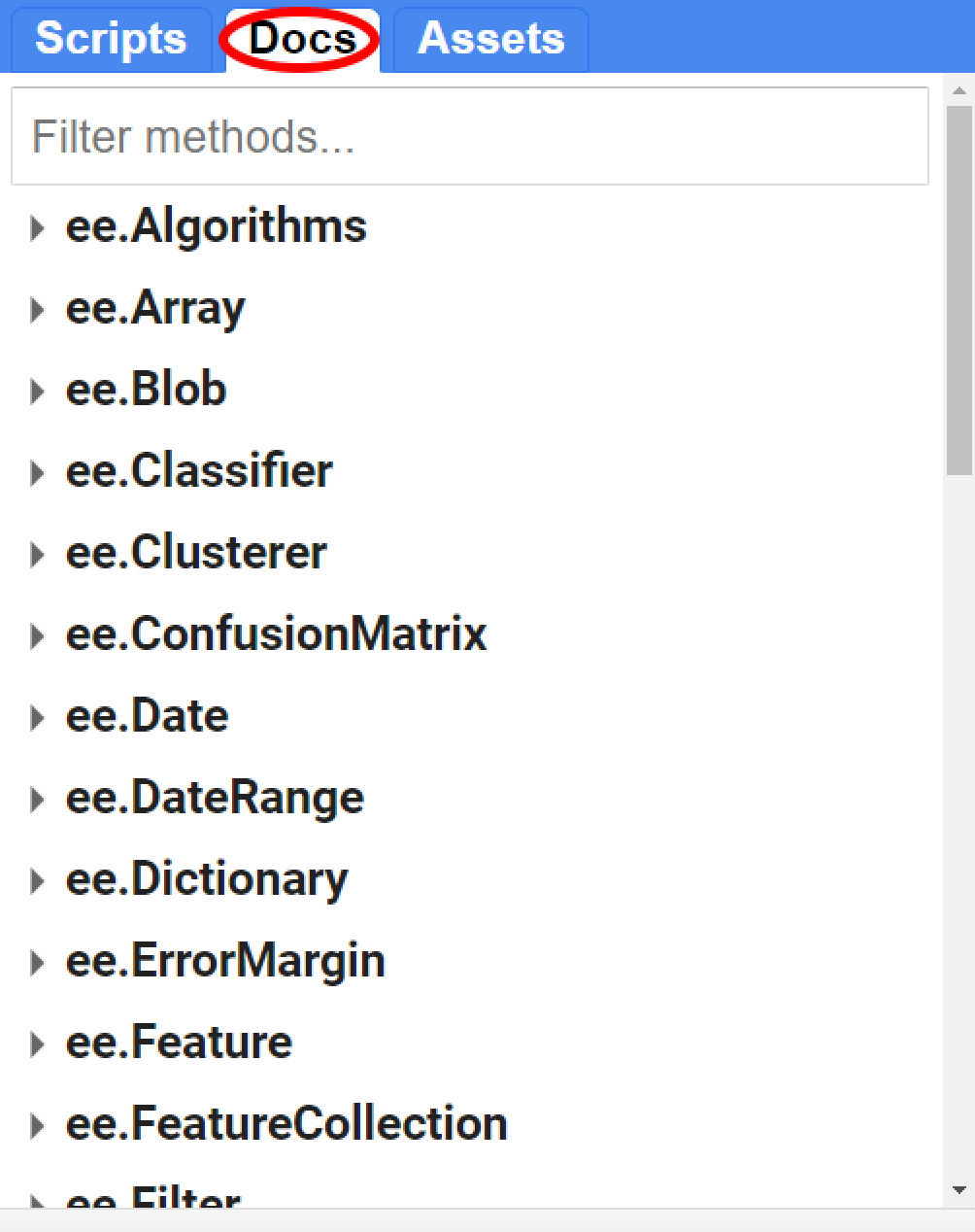
Figure 1.16: List of available objects in the Docs tab of GEE API.
Below are some examples to ilustrate the concept behind an Earth Engine object:
A) Strings
You can put strings into a ee.String
object to be sent to Earth Engine. Using an object/container allows you to do manipulate them in many different ways. For example, ee.String
has the:
* toLowerCase()
method. This method will convert any string in a ee.String
object to lower case;
* length()
method. This method will count the characters in your string;
* cat()
method. This method will concatenate two strings into one;
* and many others! Make sure to check the Docs tab for all the available methods for a given object.
The code below uses some of the methods of ee.String
as an example:
var string = ee.String('HELLO EVERYONE, ');
print(string.toLowerCase());
print(string.length());
var string2 = ee.String('nice to meet you');
print(string.cat(string2));
In your console tab you will see that the ‘HELLO EVERYONE,’ string is now all in lower case. You will also see that .length()
returned 16 as the number of characters for that string.
Consider the code below:
If you try to use a method on a non ee
object, you will get an error.
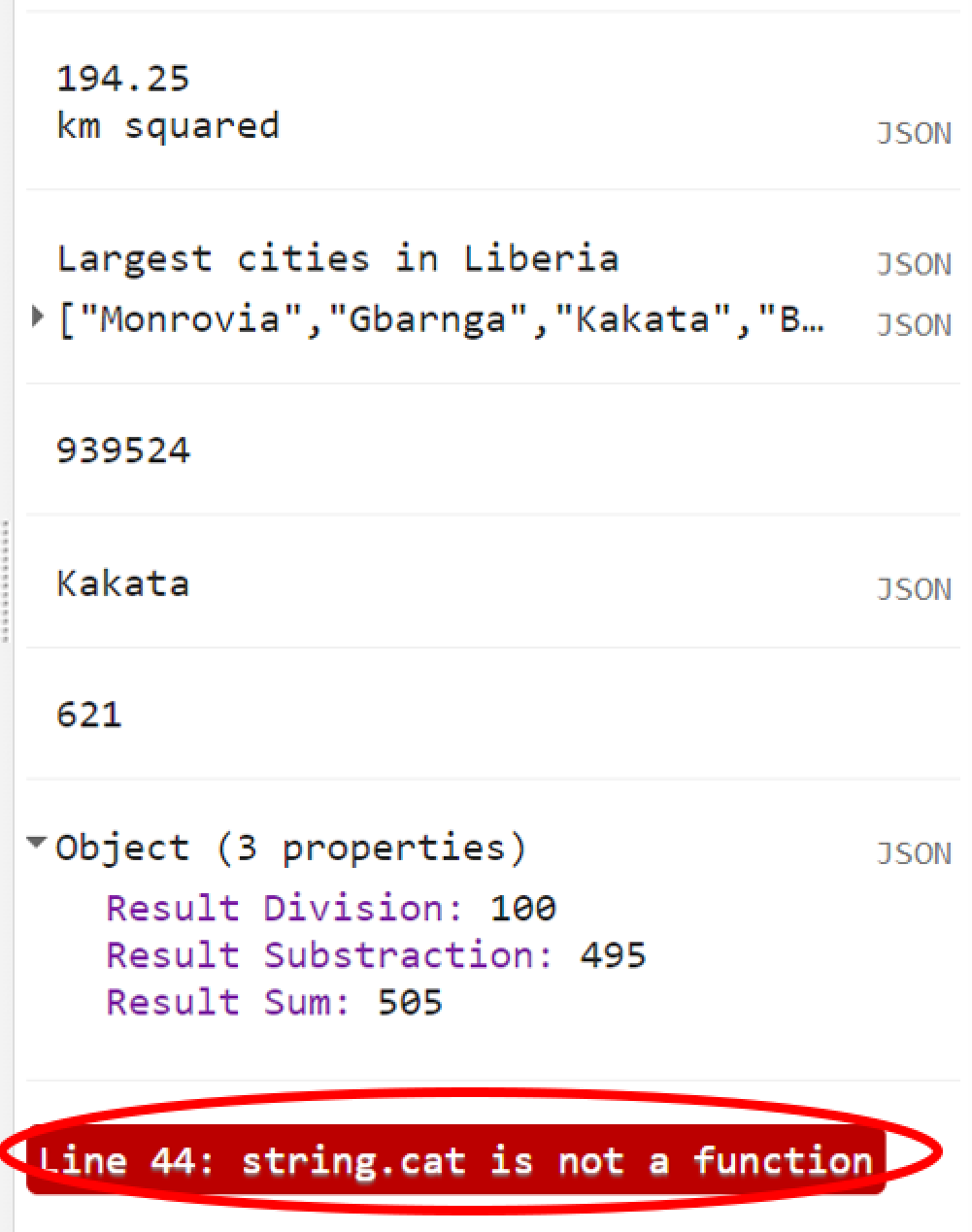
Figure 1.17: Trying to use a method on non-ee.Object will return an error
B) Numbers
ee.Number
is just another example of an object from that list. Similarly to ee.String
, it will have many methods to be used with. For example, .add()
, .subtract()
, .divide()
and .multiply ()
will perform these operations on ee.Number
objects. Make sure to consult the Docs tab for all the different methods for each object.
var value1 = ee.Number(100);
var value2 = ee.Number(2);
print(value1.add(value2));
print(value1.subtract(value2));
print(value1.divide(value2));
print(value1.multiply(value2));
print(value1.log10());
B) Lists
You can also make a JavaScript list into an ee.List
on Earth Engine server by simply casting your list into the container. There are many other useful methods to use when you have an ee.List
. For instance, instead of making a JavaScript list by typing each value, you can use ee.List.sequence()
to construct a list. This method will take many arguments, such as the value of the start of the list, the value at the end of the list, steps (increment) and count. Consider the examples below:
- Making a list with numbers from 0 to 10:
- Making a list from 0 to 10 with increments of 2:
Remember: A var string = ‘text’
is a
JavaScript string that does not allow computations. A
var string = ee.String(‘text’)
is an Earth Engine object
that allows computations!
1.1.3 Exploring Image and Image Collection
Now that you learned the basics of JavaScript and Earth Engine objects, you have the tools at your disposal to start using the Earth Engine API to build scripts for remote sensing analysis. In this section, we explore satellite imagery, which are one of GEE’s core capabilities!
A) Single band image
The first thing you need to know is that when working with images in Earth Engine, you will have to use an ee.Image()
container. The argument provided to the constructor is the string ID of an image in the Earth Engine data catalog. (Remember to always see the Docs tab for a full list of arguments to this container). To retrieve an image ID, you can search in the Earth Engine catalog using the search tool at the top of the Code Editor:
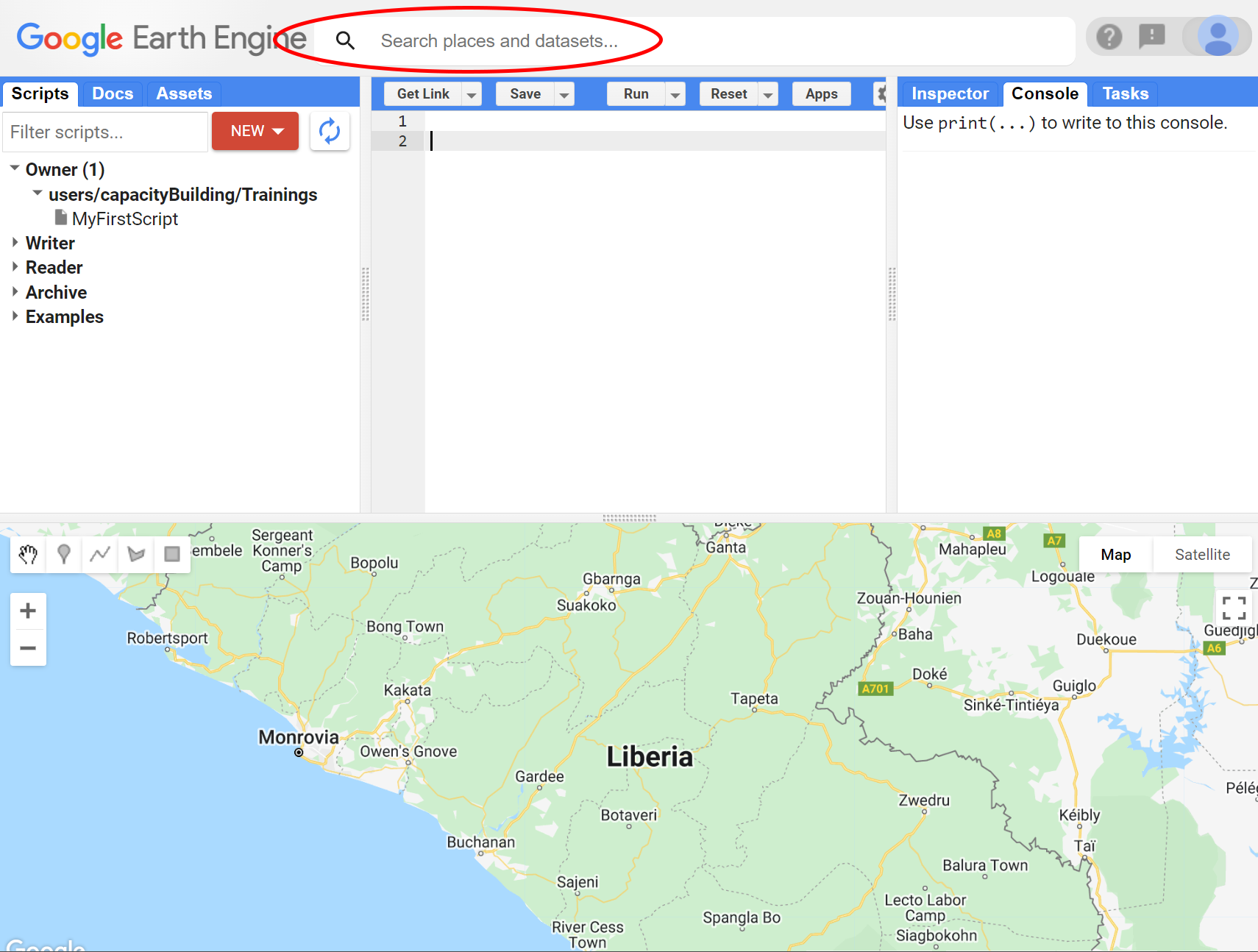
Figure 1.18: You can browse through Earth Engine’s data catalog with the search tool
For example, try typing ‘elevation’ into the search field and note that a list of rasters is returned:
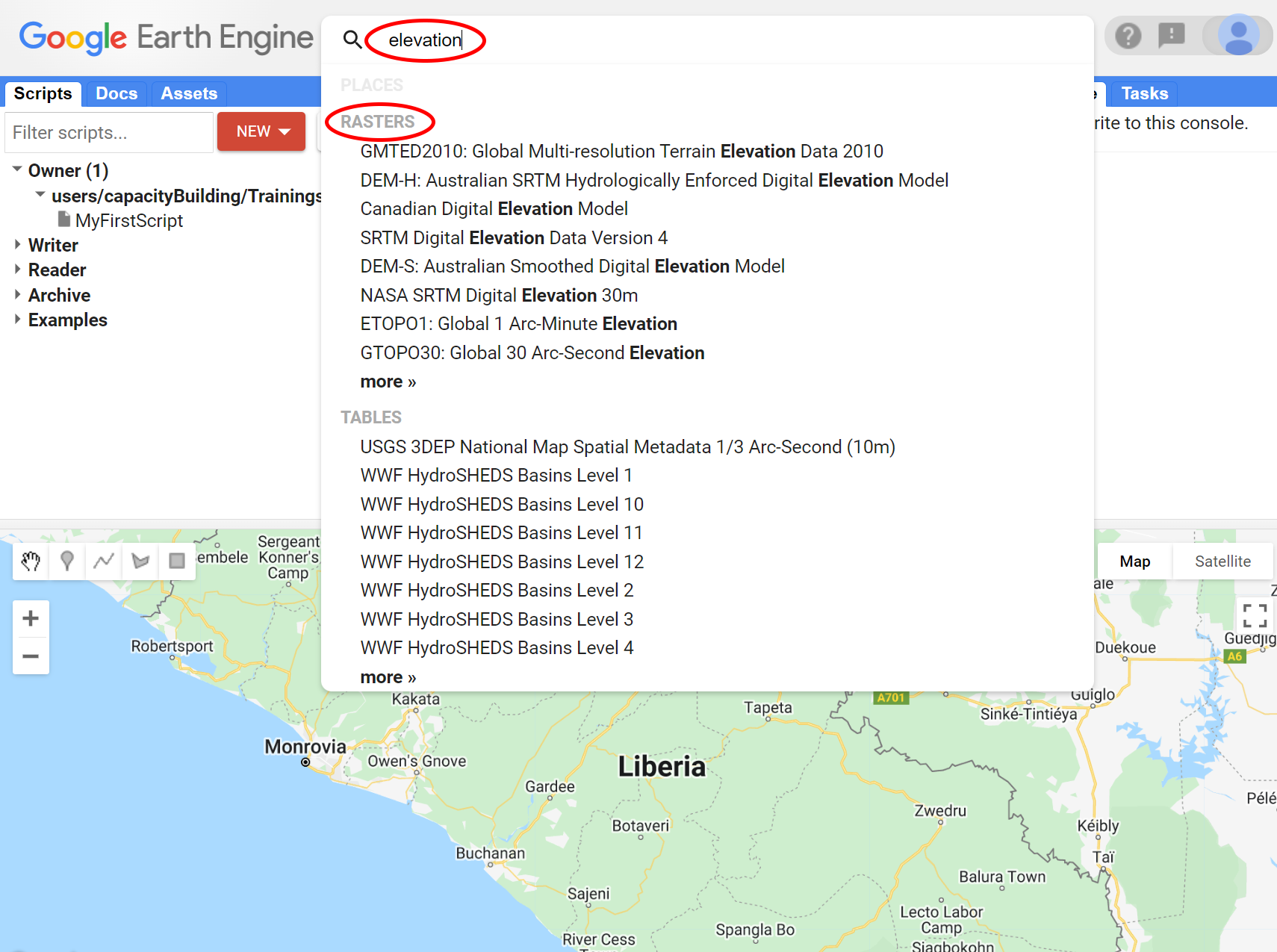
Figure 1.19: Typing in the search field. Google Earth Engine will show all datasets available with that criteria.
Click in any of the dataset entries to see more information about that dataset. From the list above, explore the dataset ‘SRTM Digital Elevation Data Version 4’. You can also find more information about this dataset on the tabs on the top right side of the dataset description screen. On the left side of the dataset description screen, you can find the Image ID, which is what we use with the ee.Image()
. Alternatively, you can use the Import button on the dataset description. Using the Import button, a variable is automatically created in a special section, named ‘Imports’, at the top of your script. You can rename the variable by clicking on its name in the imports section.
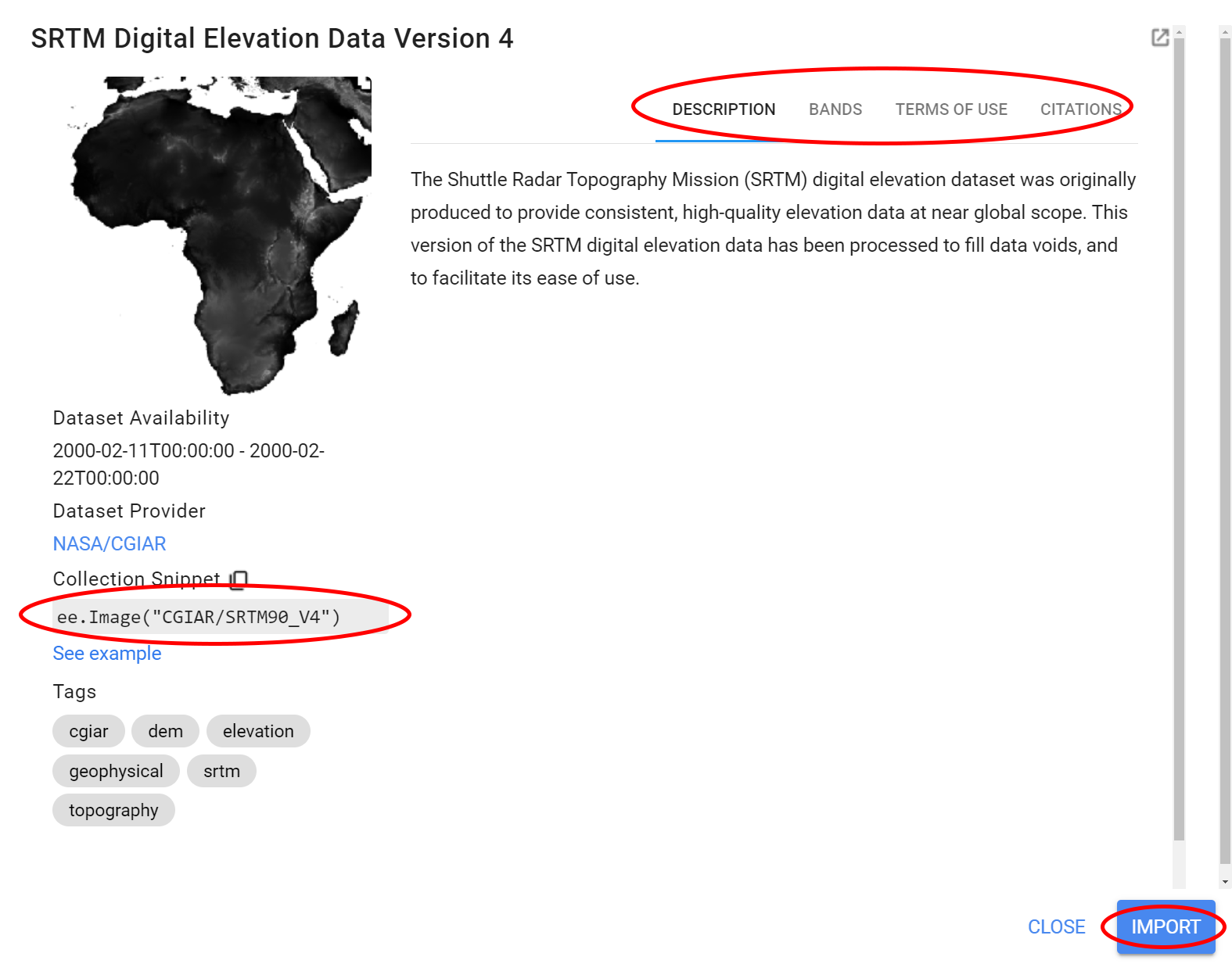
Figure 1.20: Data description
Copy the image ID from the screen above and add it to a var
elevationImage with the ee.Image()
container as shown below:
You can also retrieve the metadata about this image by using print()
. In the Console, click the expander arrows to show the information. You will discover that the SRTM image has one band called ‘elevation’.
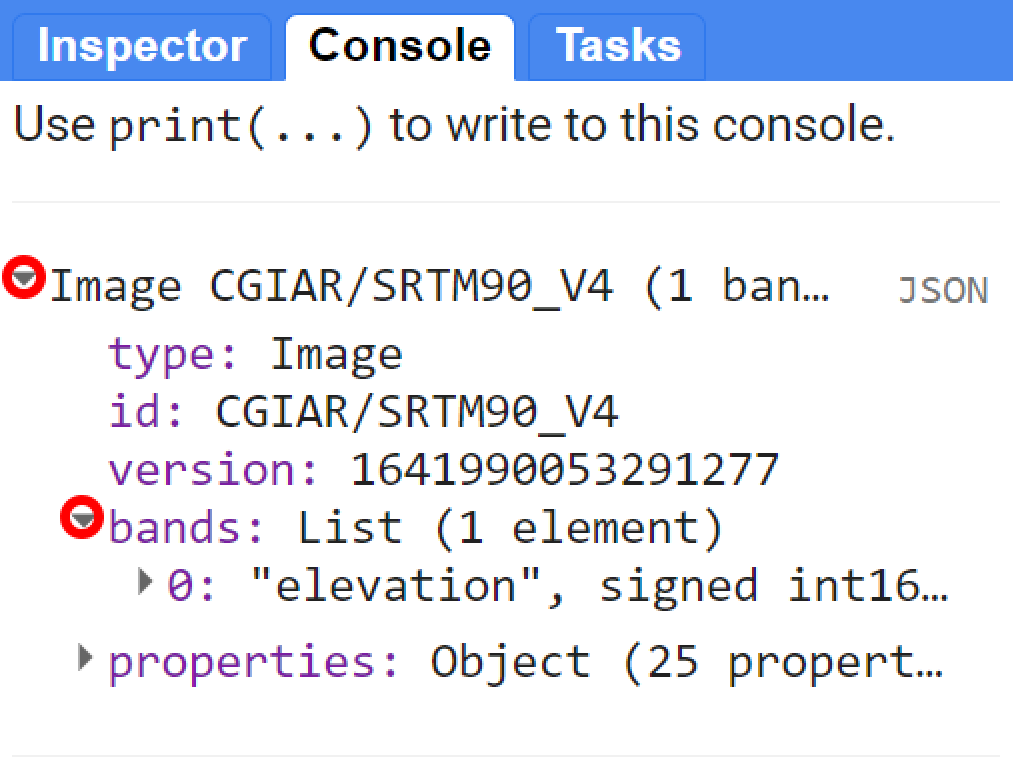
Figure 1.21: Image description using print()
To display the image in the Map Editor, use the Map
object’s .addLayer()
method. When you add an image to a map using Map.addLayer()
, Earth Engine needs to determine how to map the values in the image band(s) to colors on the display. If a single-band image is added to a map - which is our case- by default Earth Engine displays the band in grayscale, where the minimum value is assigned to black, and the maximum value is assigned to white. If you don’t specify what the minimum and maximum should be, Earth Engine will use default values.
Do not worry! You can make it look better with custom visualization parameters!
To change the way the data are stretched, you can provide another parameter to the Map.addLayer()
call. Specifically, the second parameter, visParams
, lets you specify the minimum and maximum values to display. One way of gauging the range of values of an image is by activating the Inspector tab and click around on the map. You will be able to see the value of that band for this dataset at that particular location:
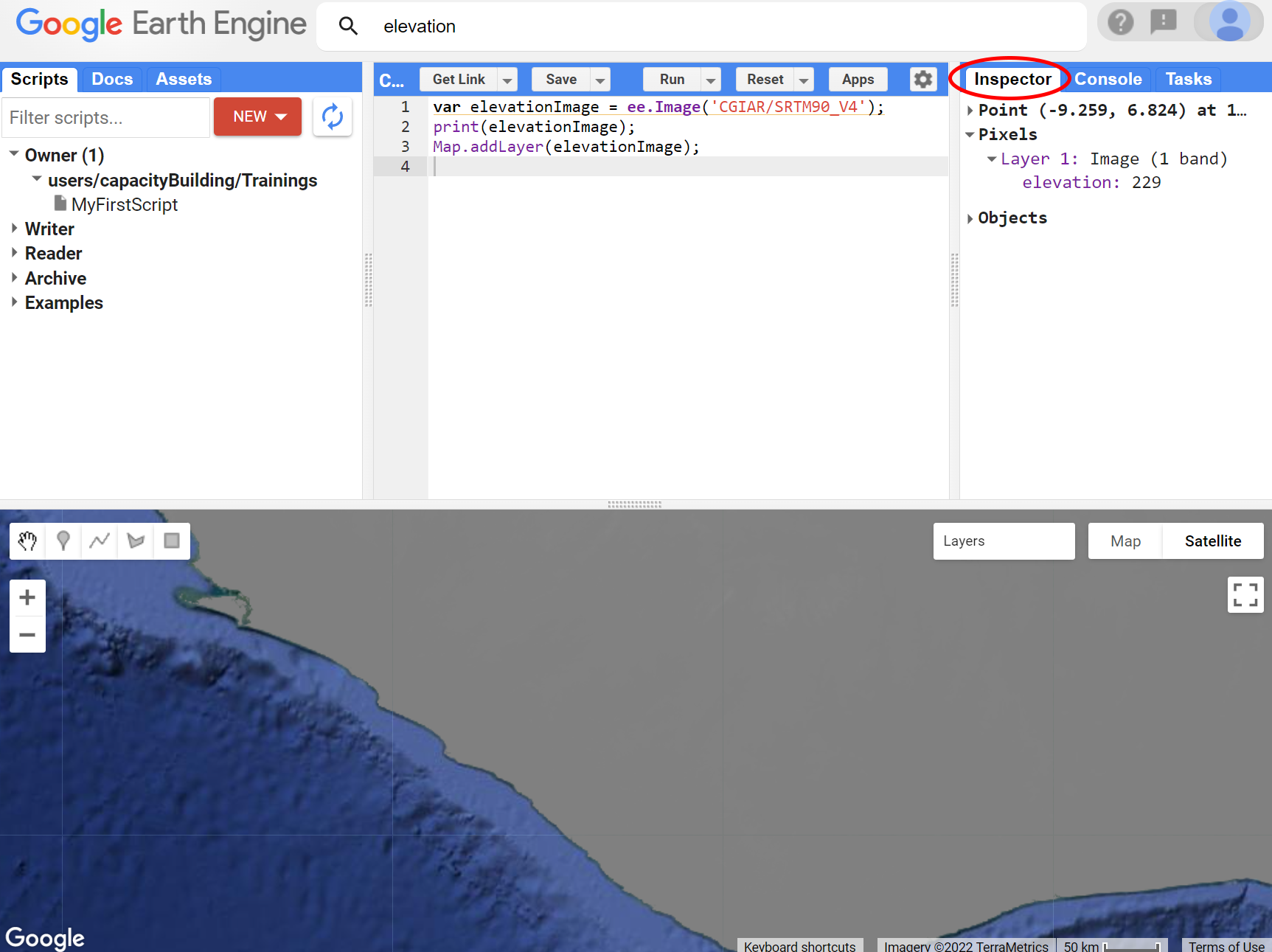
Figure 1.22: Inspector tab. Click in the image and the inspector tab will display the data for that point
Click around the area of Liberia to have a sense of the range of values for this dataset. Suppose that, through further investigation, you determine that the best range of values to display elevation data in Liberia is [0,1000]. To display the data using this range, you can use a dictionary containing two keys: min
and max
and their respective values 0
and 1000
. A third parameter for Map.addLayer()
is the name of the layer that is displayed in the Layer manager. Thus your code should be looking like the one below:
Run the code and you will see something like:
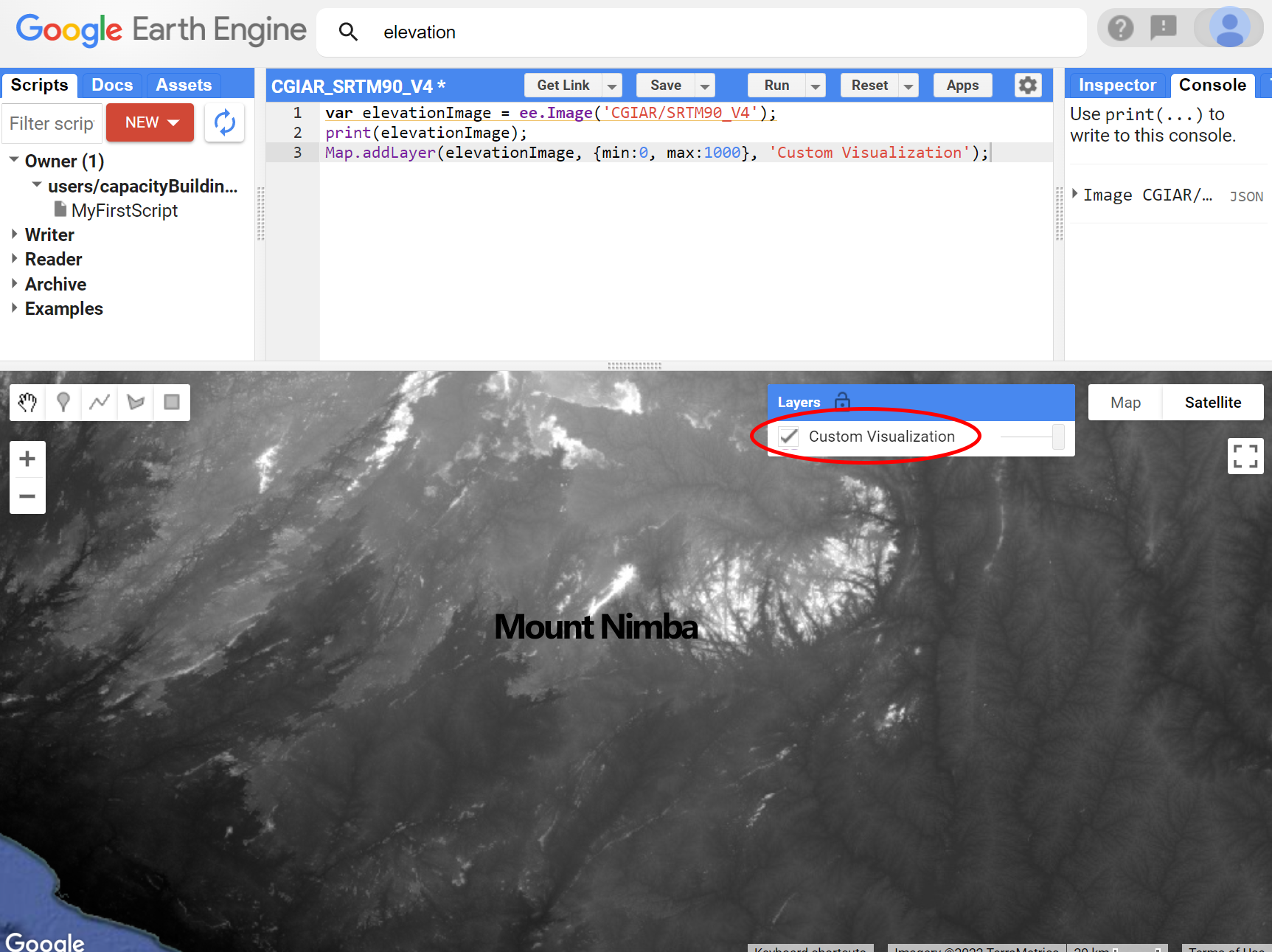
Figure 1.23: Custom Visualization for the SRTM data based on range of values.
You can further improve your image display by using a color palette. Palettes let you set the color scheme for single-band images. A palette is a comma delimited list of color strings which are linearly interpolated between the maximum and minimum values in the visualization parameters.
To display this elevation band using a color palette, add a palette
property to the visParams
dictionary:
Map.addLayer(elevationImage, {min:0, max:1000,palette: ['green', 'yellow', 'red']}, 'Custom Visualization - Color');
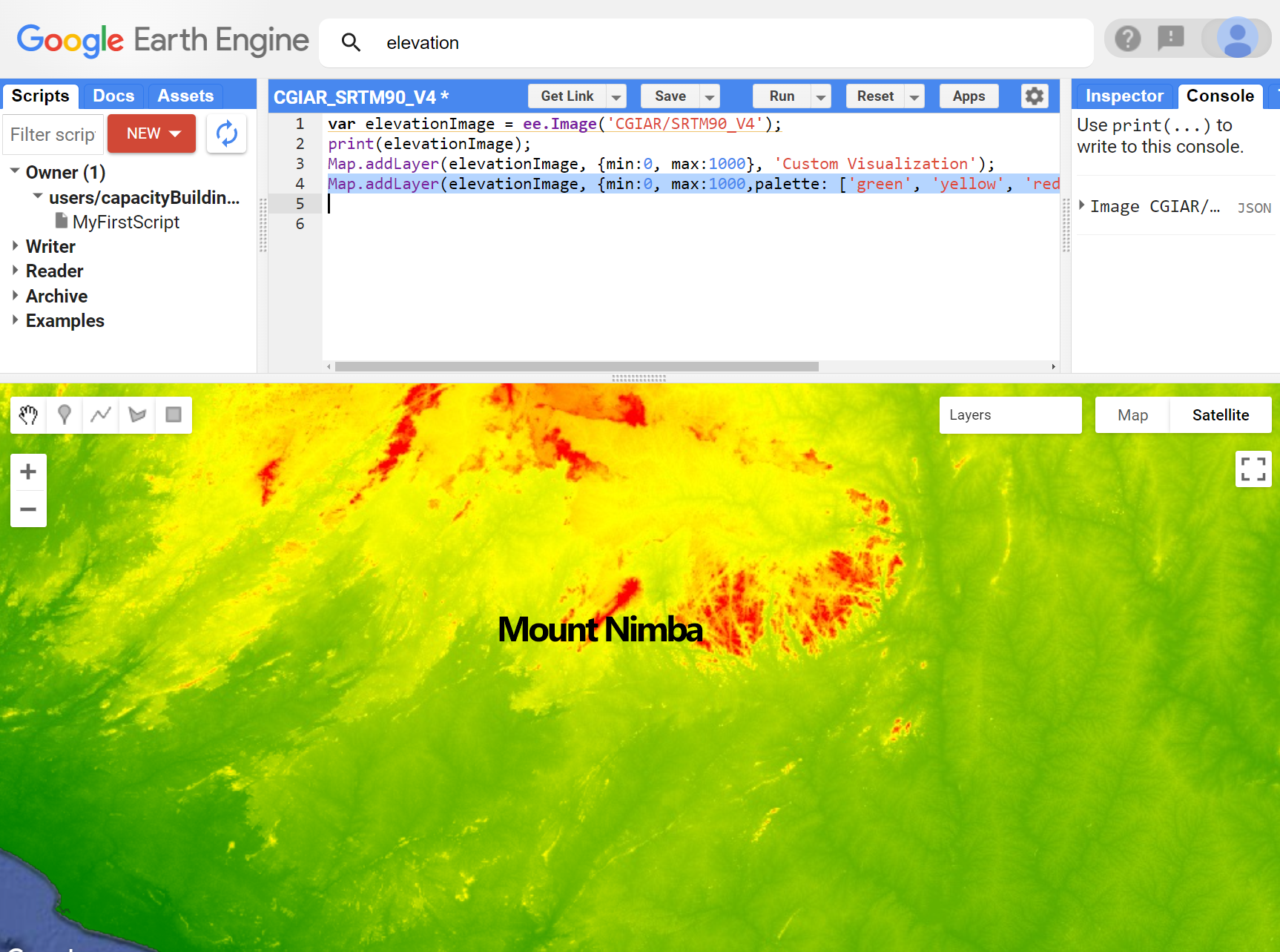
Figure 1.24: Custom Visualization for the SRTM data based on range of values and color palette.
In the next section, you’ll learn how to display multi-band imagery.
B) Multi band image
To ilustrate the concepts and codes in this section, we will use a Landsat 8 image from April 2022 over Cairo, Egypt. Landsat is a set of multispectral satellites developed by the NASA (National Aeronautics and Space Administration of USA), since the early 1970’s. Landsat images are very used for environmental research. These images have from 8 to 11 spectral bands (spectral resolution), with pixels of 30x30 meters (spatial resolution) and with a revisit time of 16 days (temporal resolution).
Copy and paste the code below into the Code Editor:
As learned before, the code above is casting a Landsat 8 image into an ee.Image
container using its ID into a var
called ‘ImageL8’. By clicking Run, Earth Engine will retrieve this image from its Landsat image catalog. You will not yet see any output.
As you learned, you can retrieve additional information about this image by using print()
.
In the Console panel, you may need to click the expander arrows to show the information. You should be able to read that this image consists of 19 different bands (index 0 to 18). Each band will have 4 properties: name, data type (as in if the value is an integer, float, ect.), projection and dimensions (in rows and columns of pixels). For this example, simply note the first property of the first band:
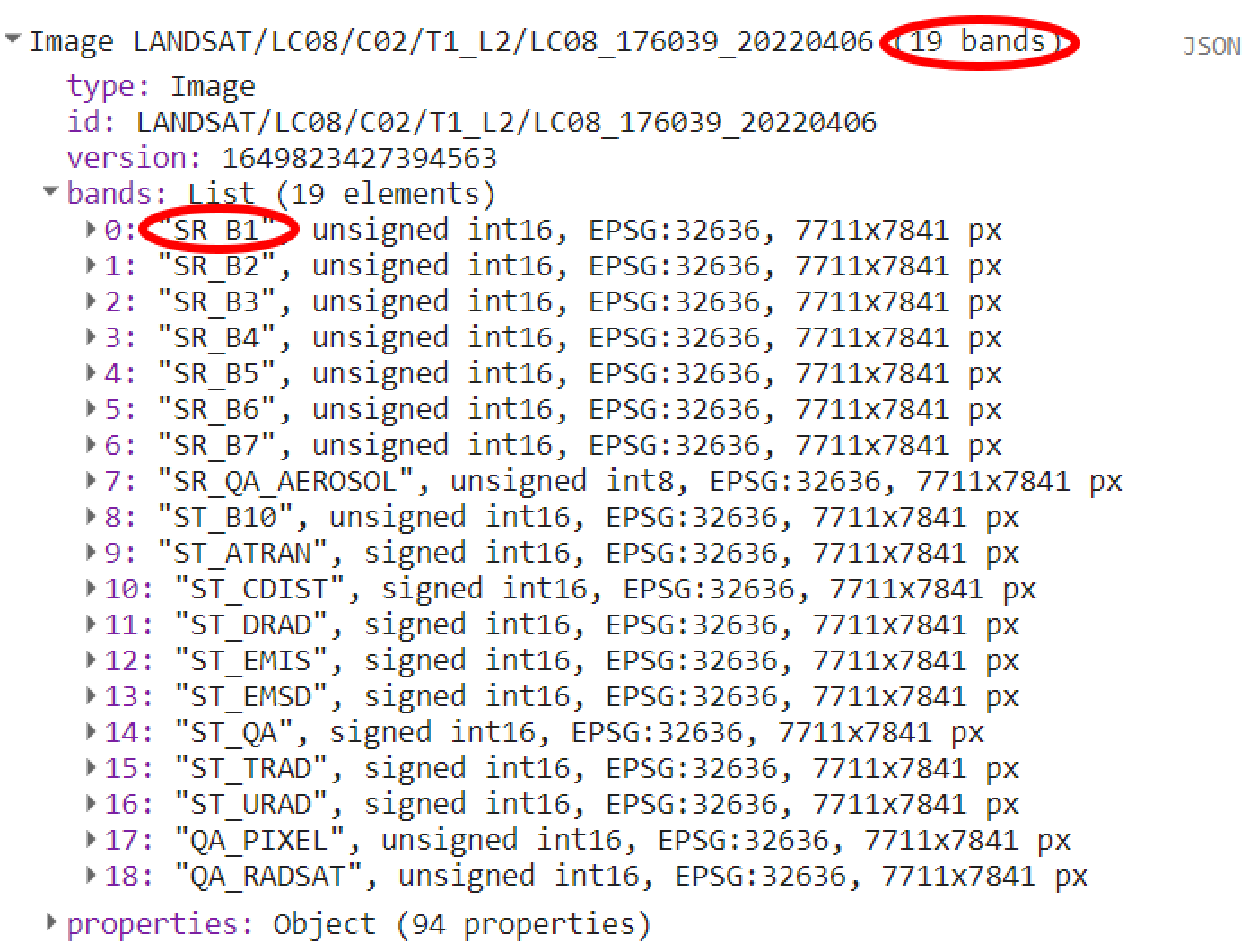
Figure 1.25: Landsat 8 image metadata.
A satellite sensor like Landsat 8 measures the EMR in different portions of the electromagnetic spectrum. Six out of seven first bands in our image (“SR_B2” through “SR_B7”) contain measurements for six different portions of the spectrum:
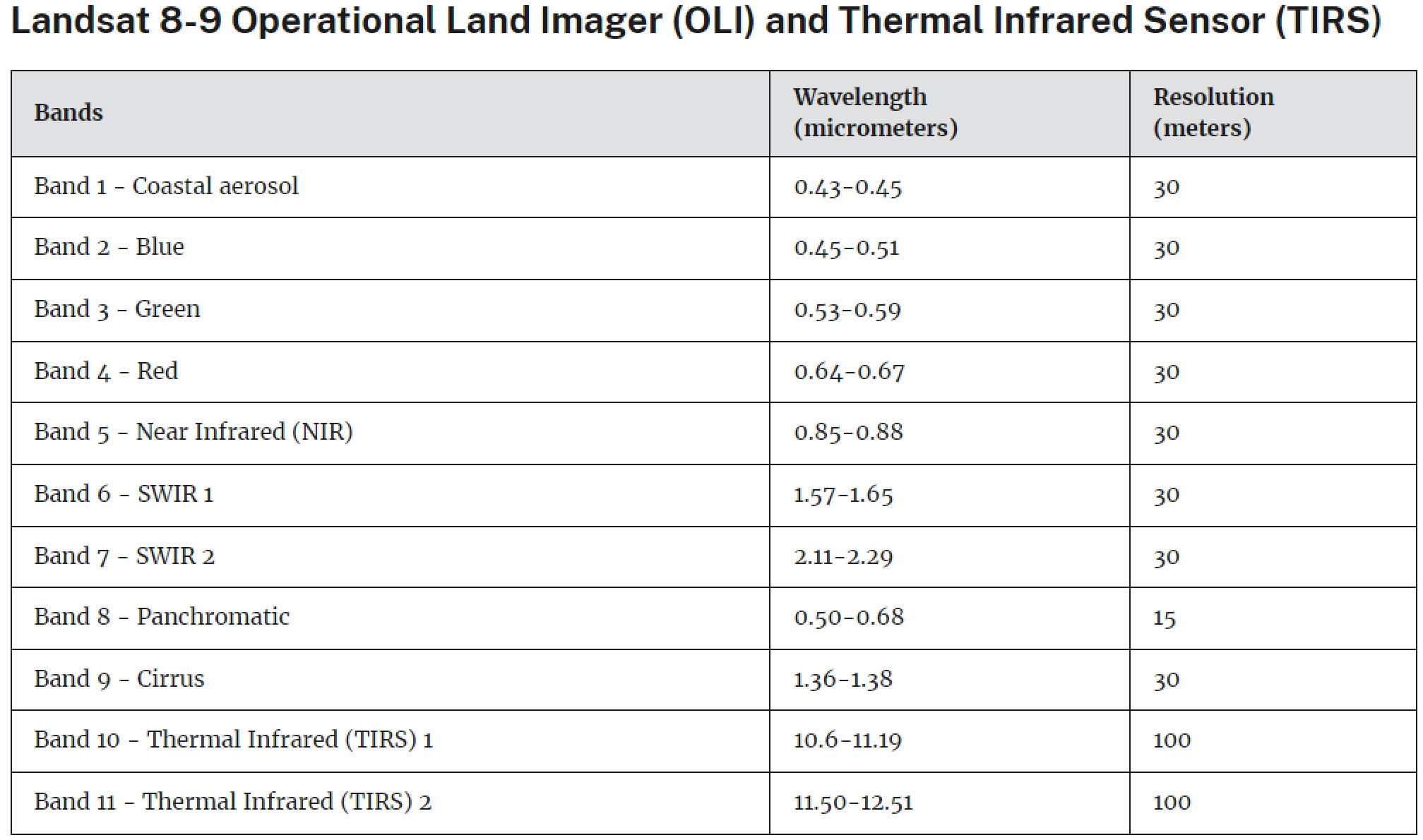
Figure 1.26: Landsat 8 bands. Source: https://www.usgs.gov/faqs/what-are-band-designations-landsat-satellites
Now, let’s visualize ImageL8
. First, make sure your map is somewhere near Cairo, Egypt. To do this, click and drag the map towards Cairo, Egypt. (You can also jump there by typing “Cairo” into the Search panel at the top of the Code Editor). Add ImageL8
to the Map as a layer using the code below:
You probably see a gray image with not a lot of details. From the previous section you learned that Map.addLayer
take many parameters. One of them is the visParams
, which lets you specify the minimum and maximum values to display. Similarly to the previous section, you can use the Inspector tab to investigate the range of values for each location for each of ImageL8
’s band. Now, specify a range of values to be displayed. Follow the code example below:
You should see something like this:
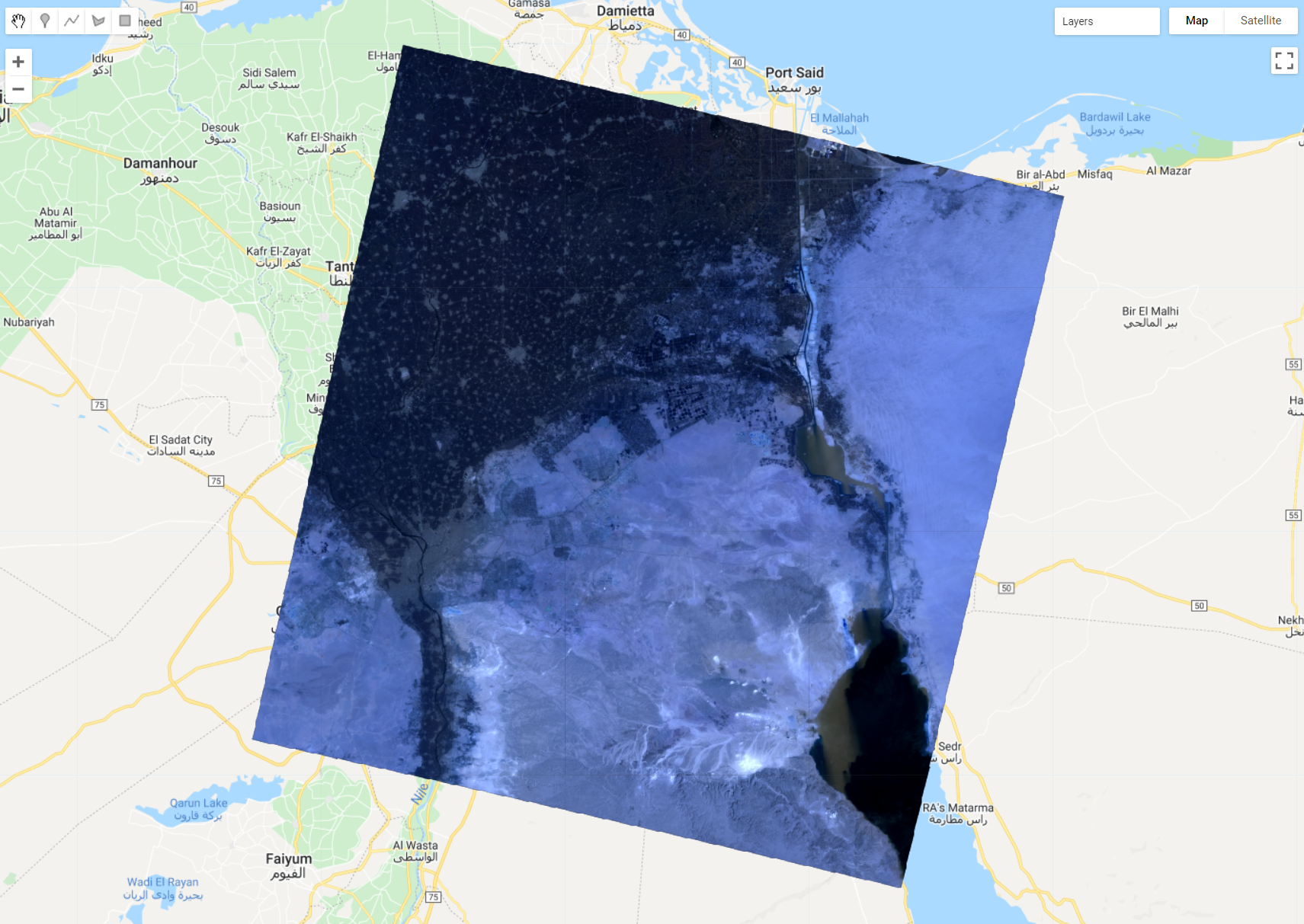
Figure 1.27: Landsat 8 image in false color .
By default, if you do not specify the bands to be displayed within the visParams
, Earth Engine will display the first three bands, each band on each RGB (red, green, blue) channel of your monitor screen. In other words, Earth Engine is displaying ‘SR_B1’ (Landsat 8’s coastal aerosol band) in the red channel, ‘SR_B2’ (Landsat 8’s blue band) in the green channel and ’SR_B3 (Landsat 8’s green band). This is a case of a false color display, when spectral bands do not match the RGB channels in your screen. False color displays have many advantages and they will be explored later in the tutorial.
For a real color display, you will need to define the bands within visParams
to match the RGB channels:
In the code above, visParams
contains a list (bands
) with the names of the ImageL8
’s bands to be displayed in the RGB channels of your screen. This band set up tells Earth Engine to display the red band in the red channel, the green band in the green channel and the blue band in blue channel. We call this composition a RGB 432 or Real color composition. You should see an image like this when running the code above:
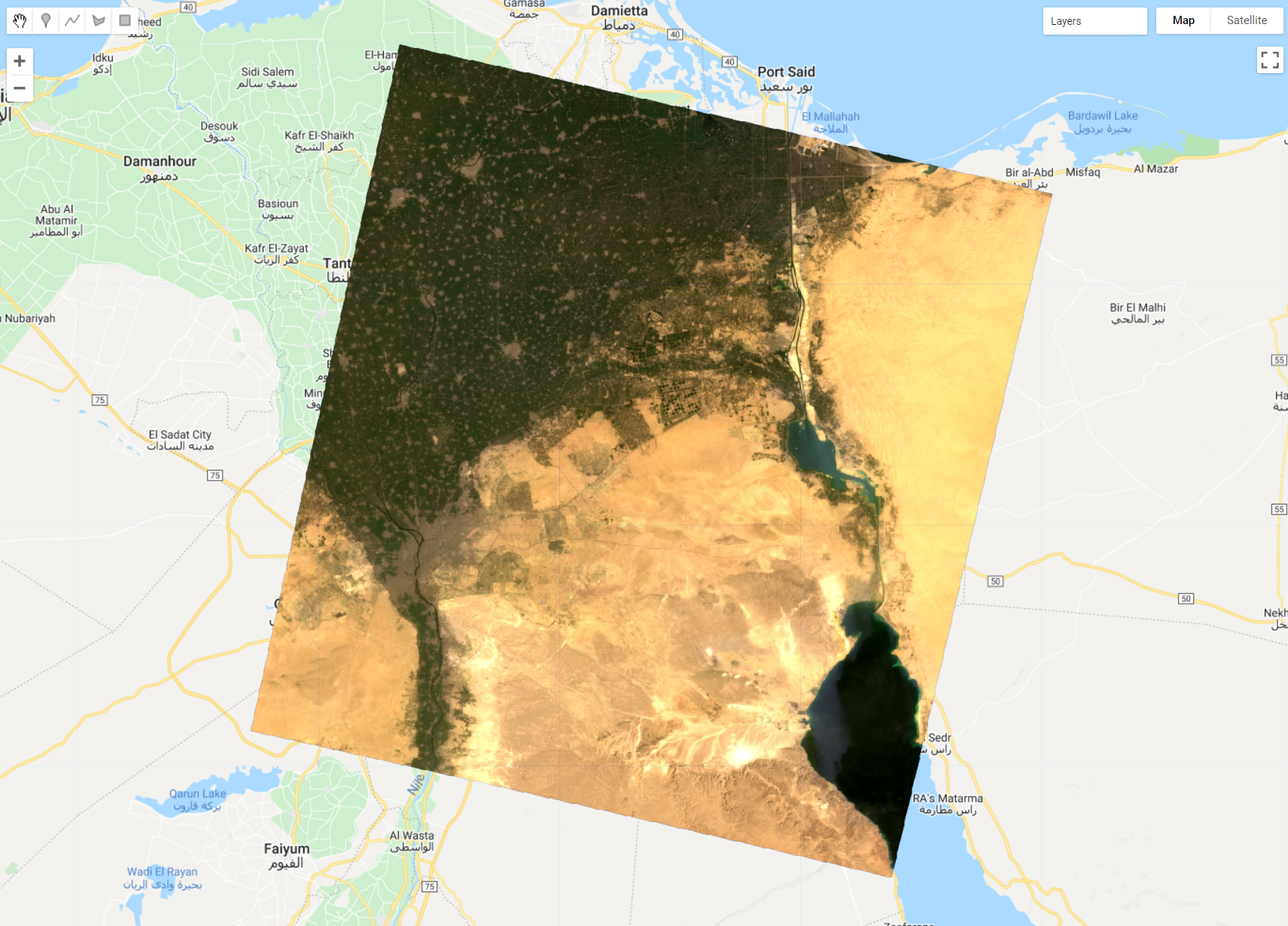
Figure 1.28: Landsat 8 image displayed with a real color composition.
You can easily change these values and settings within visParams
by using the Layer setting option in the Map editor. You can find it by hovering your cursor over the Layer List (upper right corner of the Map editor, next to the Map and Satellite buttons) and clicking the gear icon of the layer:
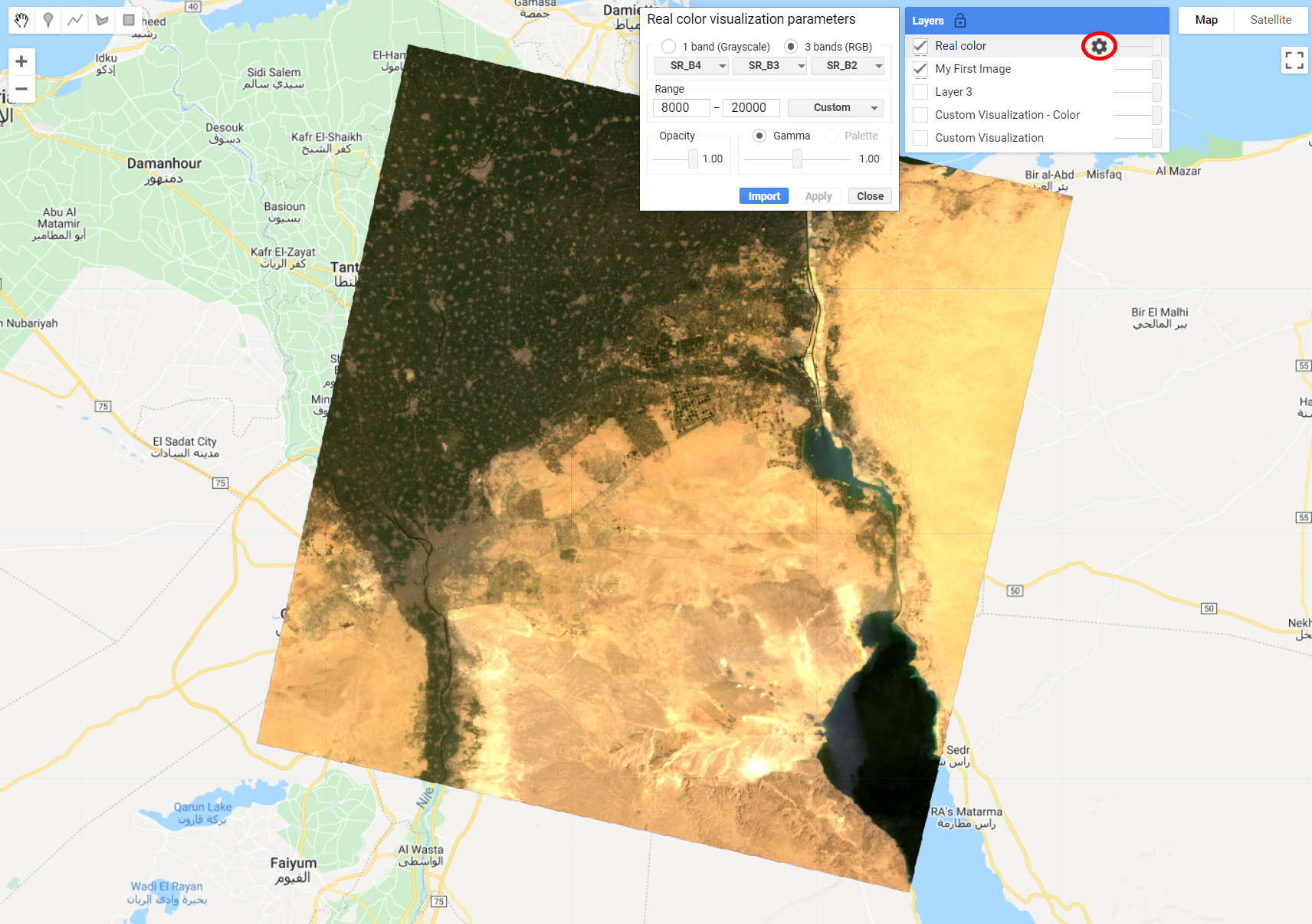
Figure 1.29: Layer settings window.
Once you chose your settings, click Apply. Earth Engine will automatically apply the new settings and display the layer. Try using different band combinations and range values to see how the image changes. Different color compositions will highlight different features in the image based on their spectral signatures!
C) Image collections
An image collection refers to a set of Earth Engine images. For example, the collection of all Landsat 8 images! In this case, you will use ee.ImageCollection()
instead of ee.Image()
to retrieve a particular image collection . Like the SRTM image or the Landsat image you have been working with, image collections also have an ID. Similarly to the single images, you can discover the ID of an image collection by searching the Earth Engine data catalog from the Code Editor.
Start by loading the Landsat 8 image collection into a var
called ’collection using the ee.ImageCollection
container. Then try to print this collection to the Console:
You will notice that you will get an error when trying to print collection
to the Console:

Figure 1.30: Trying to print an image collection without any filter.
It’s worth noting that this collection represents every Landsat 8 scene collected, all over the Earth. Thus, Earth Engine is not able to print the information for every scene all over the globe since 2013 (when Landsat 8 started collecting data). In this case we need to filter this collection.
Exploring the Docs tab of the Code Editor to learn more about ee.ImageCollection
, you will notice the methods filterBounds()
and filterDate()
. These are shortcut methods on a bigger filter()
method. In this case, filterBounds()
filters a collection by intersection with geometry (or a location) while filterDate()
filters a collection by a date range, expressed as strings.
Location Filter: To filter collection
to images that cover a particular location, first define your area of interest with the geometry drawing tools. To create geometries, use the geometry drawing tools in the upper left corner of the map display.
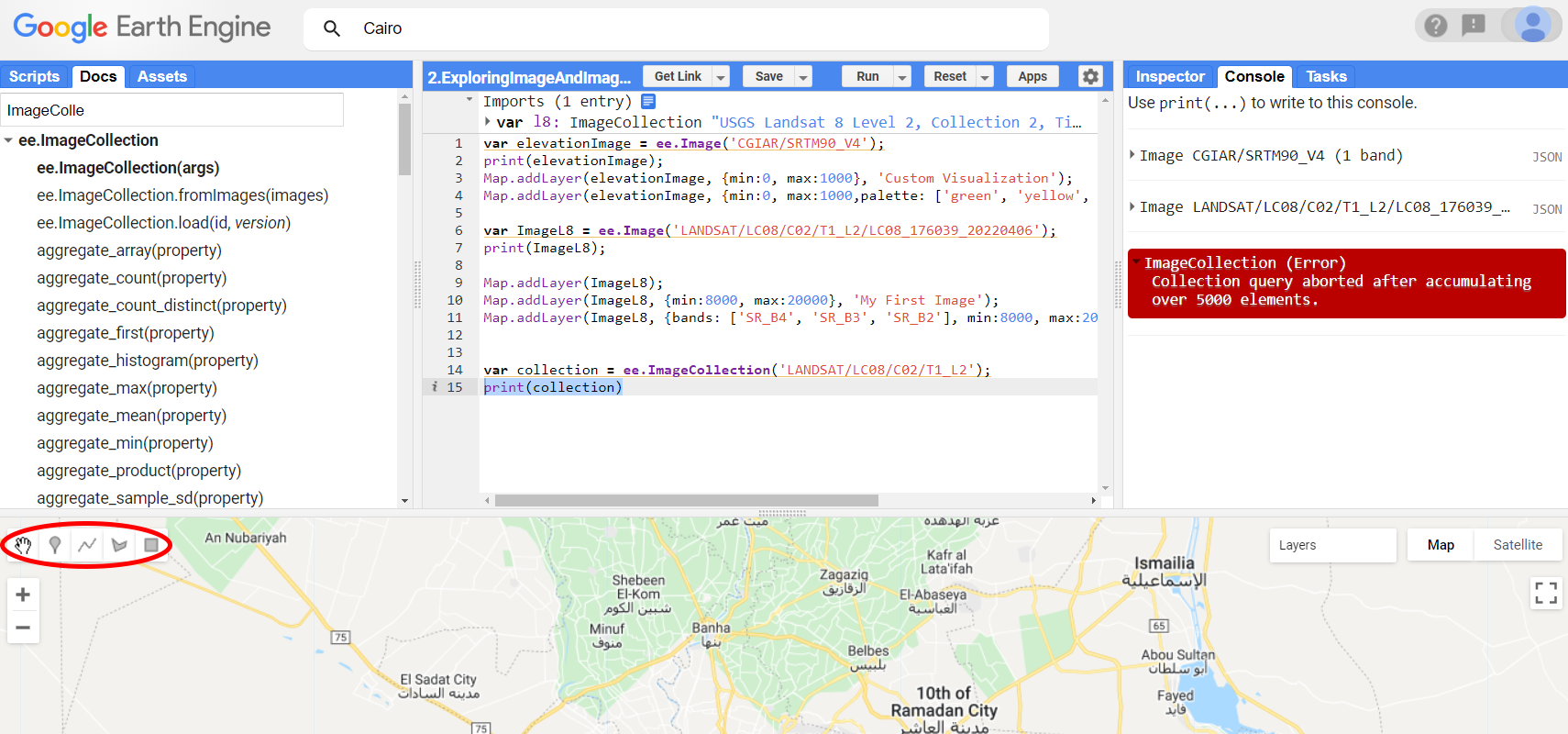
Figure 1.31: The Geometry tools.
For drawing points, use the place mark icon, for drawing lines, use the line icon, for drawing polygons, use the polygon icon, for drawing rectangles, use the rectangle icon. Using any of the drawing tools will automatically create a new geometry layer and add an import for that layer to the Imports section (top of the script). Once you finish drawing the geometry, click Exit. To rename the geometries that are imported to your script, click the settings icon next to it (or rename it directly in the Imports section).The geometry layer settings tool will be displayed in a dialog where you can change the geometry name.
Use the point mark and create a point geometry named ‘aoi’ (area of interest) in a location of your interest. For this example, we will use a point mark location over Cairo, Egypt. Use the figure below as a guide: 1) Click on the point mark geometry; 2) Place it in your location of interest, and; 3) rename it ‘aoi’.
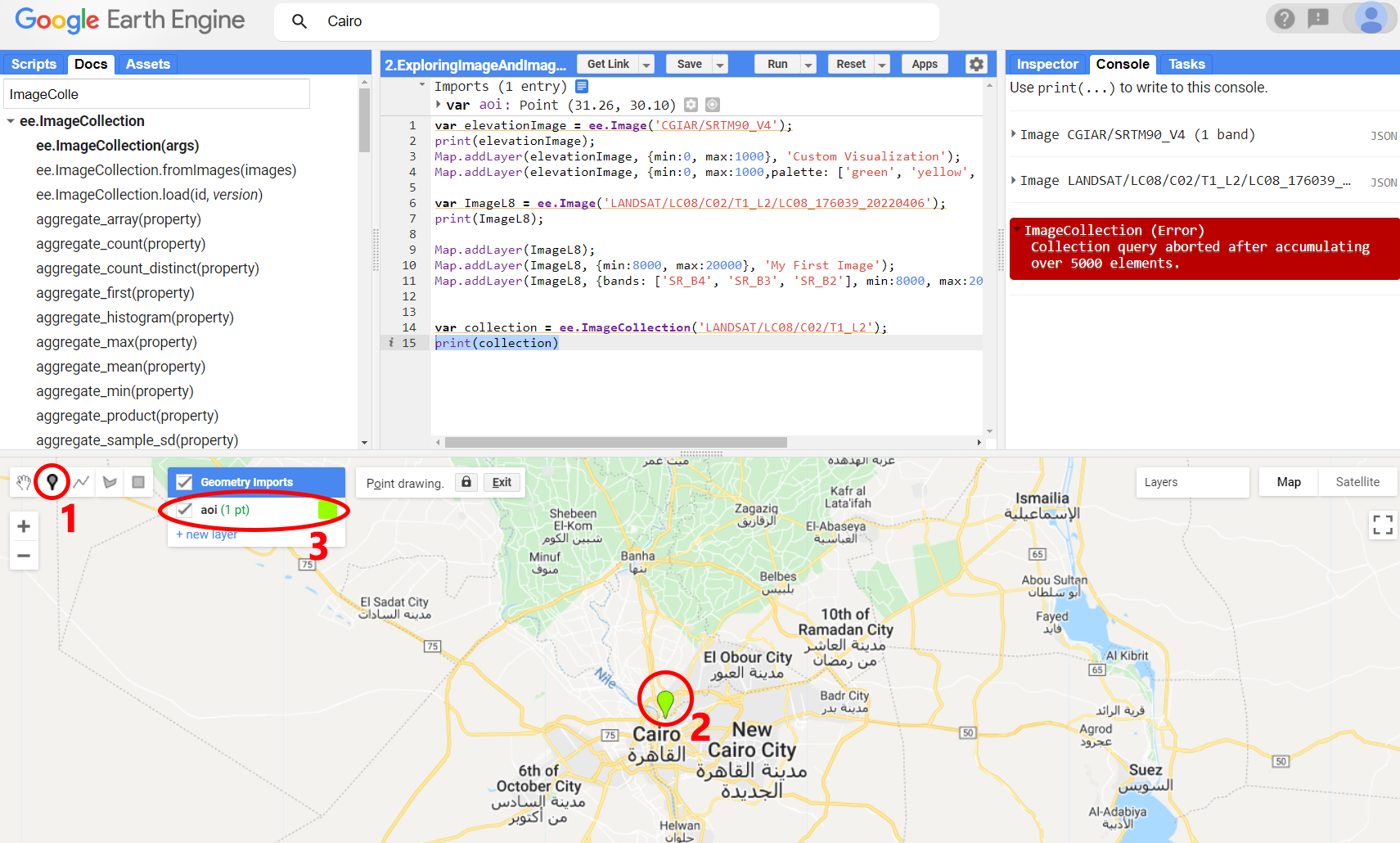
Figure 1.32: Creating a point geometry.
Date filter: Now that you have a location, create two variables startDate
and endDate
containing a data range expressed as strings.
Use a date range of your choice. Dates are expressed as ‘YYYY-MM-DD’ in Earth Engine. For this example, we will use the first four months of 2022:
Now that you have both filters for location and date range, you are ready to filter your image collection using filterBounds()
and filterDates()
:
var collectionFiltered = collection.filterBounds(aoi).filterDate(startDate,endDate);
print(collectionFiltered);
When printing collectionFiltered
to the Console you will notice that now Earth Engine was able to retrieve the information of every Landsat scene based on your filters. Using the date range and the location filters from this example, there are the 13 images in the collectionFiltered
for Cairo from January to April, 2022:
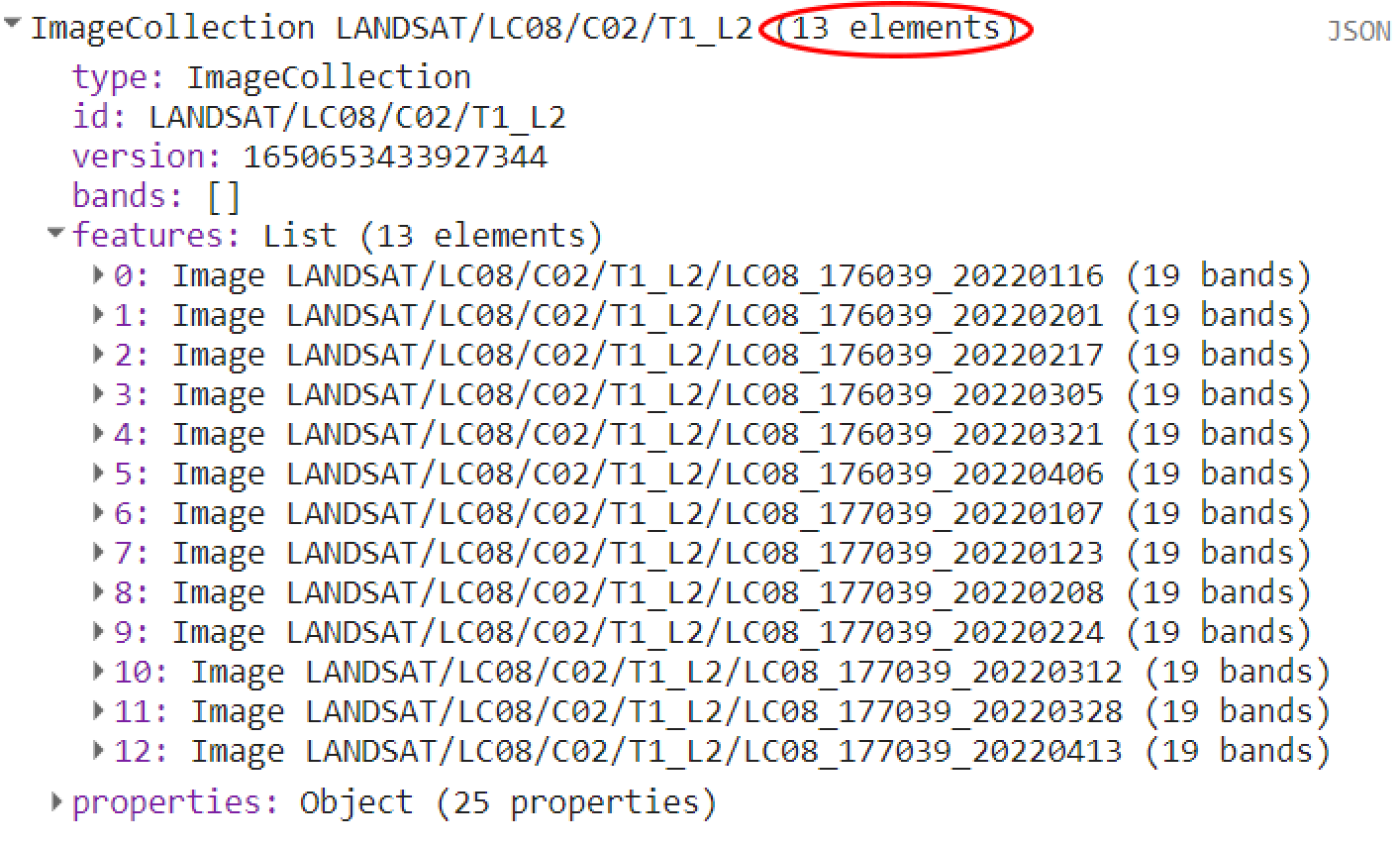
Figure 1.33: Filtered collection.
If you want to retrieve a particular image from this collection you can simply use ee.Image()
and the image ID printed in the Console tab!
1.2 Image and Image Collection Manipulation
Now that you know how to load and display an image, it’s time to apply a computation to it. In the following sections you will learn some examples of computations for a single-band image and multi-band image (band math and vegetation index calculation) and for an image collection.
1.2.1 Image Math
- Single-band image
Considering our previous example, we will use the SRTM single-band image elevationImage
and create a ‘slope’ image. Briefly, you calculate the slope by dividing the difference between the elevations of two points by the distance between them, then multiply the quotient by 100. The difference in elevation between points is called the rise. The distance between the points is called the run. Thus, percent slope equals (rise / run) x 100. Intuitively, you can think of doing this calculation manually by applying .divide()
and .multiply()
. However, as you will learn with this material, Google Earth Engine has specific methods within ee
objects to perform computations such as slope, for example.
Simply, you can create an the ‘slope’ image with the slope
method of the ee.Terrain
package:
Note that elevationImage
was provided as an argument to the slope method! Add slope
to the map and find Mount Nimba, in Liberia. Use a min
and max
values to reflect a resonable range of % slope.
Explore the slope image around Mount Nimba, in Liberia. It should look like the figure below:
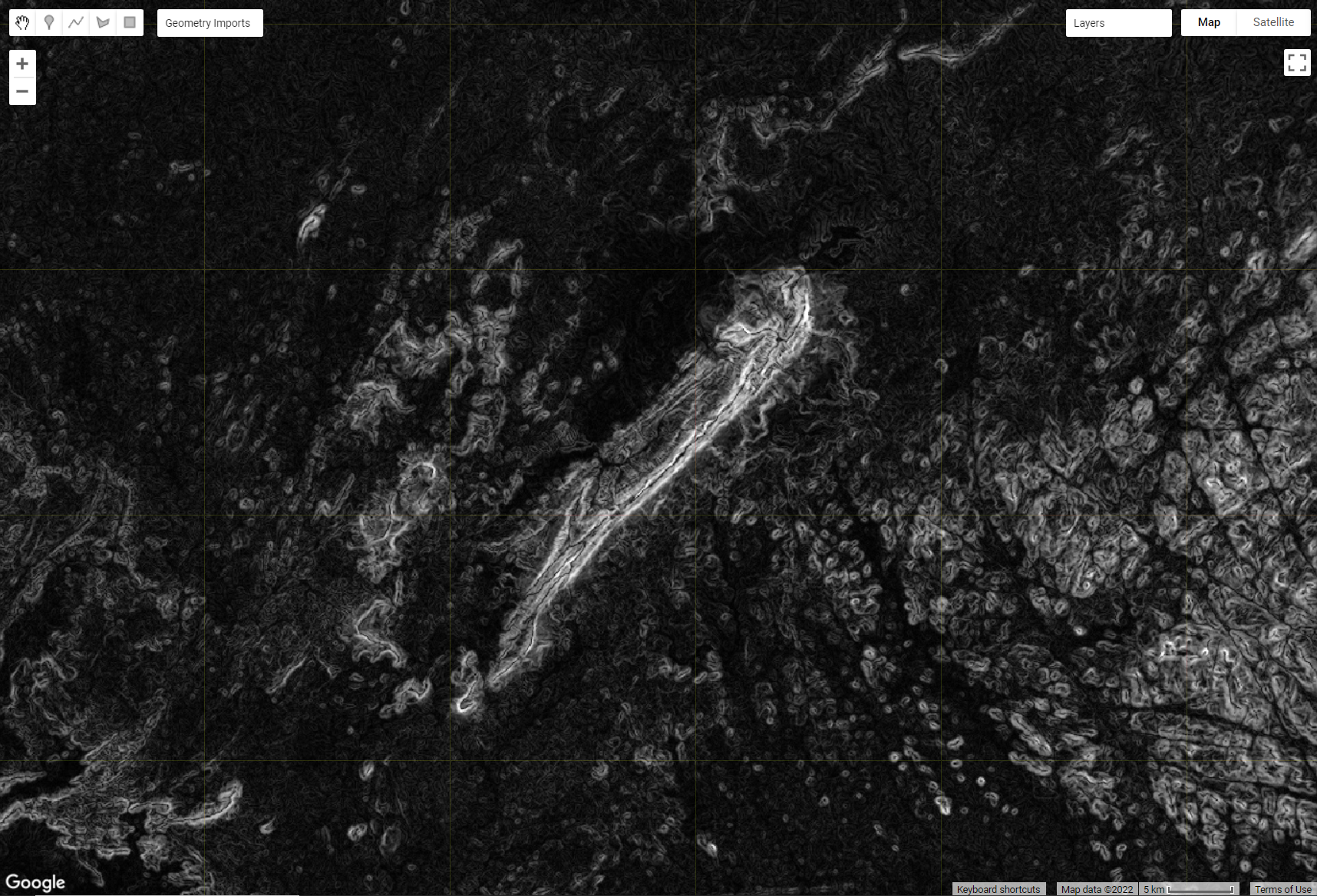
Figure 1.34: Slope image calculated with the .slope()
method of the ee.Terrain
package. Mount Nimba in Liberia is shown in white.
As mentioned before, there are also methods in the ee.Image
container that can be invoked on an image object. We call it band math when you do mathematical operations with image bands. Still considering the previous example, suppose you are interested in further processing the slope image into an aspect image and then perform some trigonometric operations on it. Aspect, in this case, refers to the orientation of a slope, measured clockwise in degrees, from 0 to 360. Similarly to .slope()
, .aspect()
is also an method from ee.Terrain
.
Now, convert the image aspect
into radians then calculate its sin:
It is worth noting that the code above chained multiple methods. This way, you can perform complex mathematical operations. In other words, the code above is simply dividing the aspect by 180 (using the .divide()
method), multiplying (with .multiply()
) the result of that by π (that can be retrieved using Math.PI
), and finally taking the sin (with .sin()
). The result should look something like the figure below:

Figure 1.35: Aspect image calculated with the .aspect()
method of the ee.Terrain
package. The resulting SIN image from aspect reveals Mount Nimba in greater detail.
- Band math with multi-band image
As mentioned previously, you can do mathematical operations with bands from a given image. One of the most common examples of band math with remote sensing imagery is the calculation of spectral indices. A spectral index is a mathematical equation that is applied on the various spectral bands of an image per pixel, with the objective of highlighting pixels showing the relative abundance or lack of the feature of interest. There are several categories of spectral indices that have been developed, using a variety of spectral bands to highlight different phenomena, such as water, snow, soil and vegetation. For example, Vegetation Indices (VIs) are combinations of surface reflectance at two or more wavelengths designed to highlight a particular property of vegetation.
A) NDVI
The most used index in this category is the Normalized Difference Vegetation Index (NDVI), which provides an indication of abundance of live green vegetation (or abundance of chlorophyll). The pigment in plant leaves, chlorophyll, strongly absorbs visible light (from 0.4 to 0.7 µm) for use in photosynthesis. The cell structure of the leaves, on the other hand, strongly reflects near-infrared light (from 0.7 to 1.1 µm) (See Figure 1.4). Thus, NDVI is calculated by comparing the different reflectance values of the red and near-infrared bands (normalized such that the minimum value is -1.0, and the maximum is +1.0).
Bringing this concept to the context of a Landsat 8 image, we can produce an NDVI image by computing the normalized difference between its bands 5 (near-infrared) and 4 (red) (See Figure 1.26).
For this example, we will use ImageL8
created in the previous sections to create a NDVI image. We can use the method .select()
on a ee.Image
object to select any given band. The .select()
method will take a string as an argument for the band name you want to select. Then we can use the mathematical operators to perform a normalized difference using these bands:
var nirBand = ImageL8.select('SR_B5');
var redBand = ImageL8.select('SR_B4');
var NDVI = nirBand.subtract(redBand).divide(nirBand.add(redBand));
There is another way of doing the same calculation from the code above. As we seen previously, Earth Engine usually have methods for widely used operations and computations with remote sensing data. In this case, the normalized difference operation is available as a shortcut method .normalizedDifference()
for a ee.Image()
object. It take as an argument a list with the names of the bands you wish to calculate the normalized difference with. Thus, NDVI
can be rewritten as:
You can then visualize this NDVI image by adding it to the Map Editor. Add it to the map and use the Inspector tab to investigate the range of values of NDVI around Cairo:
You should see something like this:
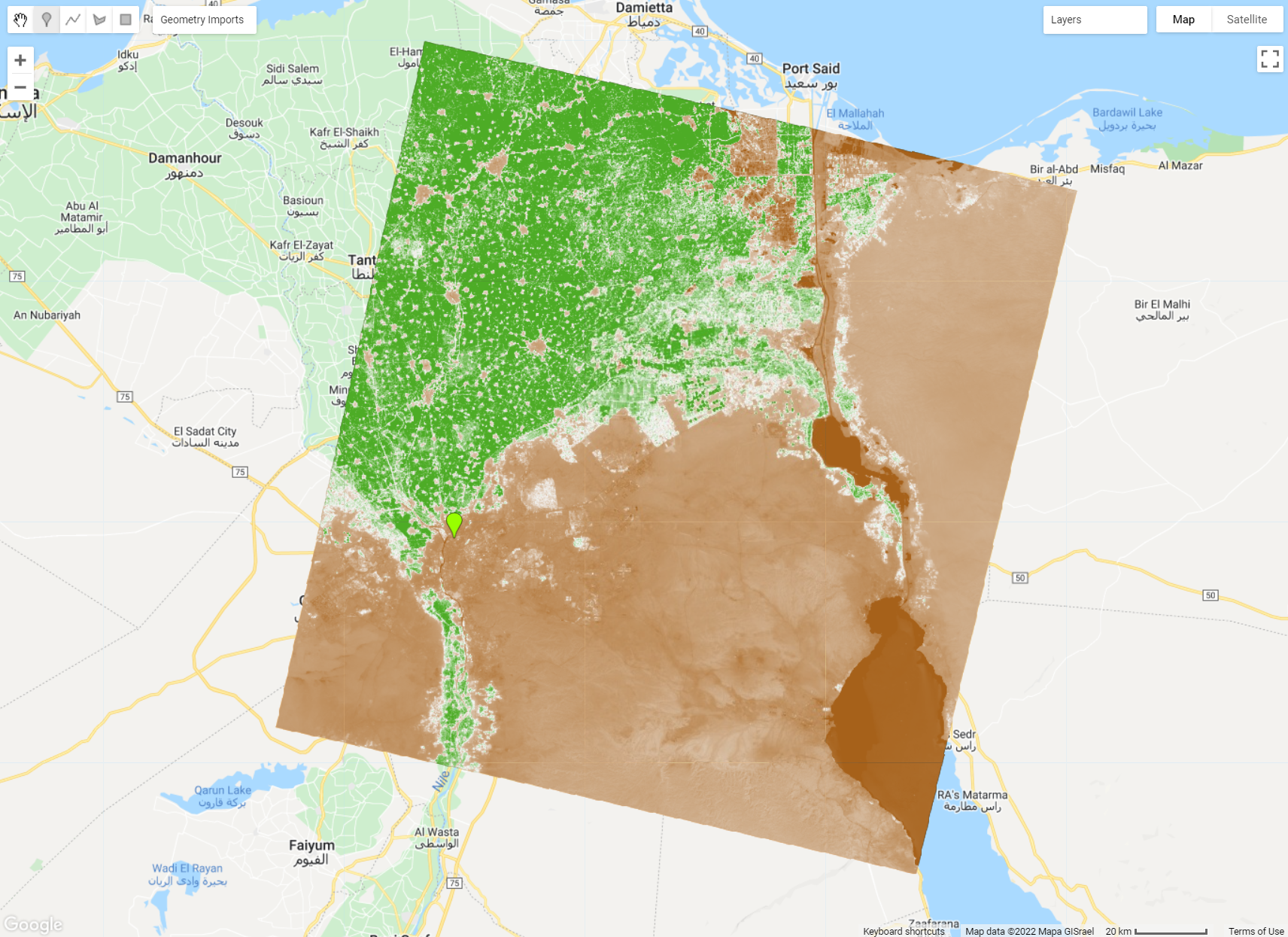
Figure 1.36: NDVI Image highlighting the agricultural area in the Nile Delta. With the visualization parameters provided above, higher values of NDVI (that is, higher content of chlorophyll in live green leaves) is shown in green.
A) EVI
EVI, or Enhanced Vegetation Index, is similar to NDVI and can be used to quantify vegetation greenness. However, EVI corrects for some atmospheric conditions and canopy background noise and is more sensitive in areas with dense vegetation where NDVI usually saturates. It incorporates an “L” value to adjust for canopy background, “C” values as coefficients for atmospheric resistance, and values from the blue band (B):
EVI = 2.5 * ((Near-infrared - Red) / (Near-infrared + C1 * Red – C2 * Blue + L)), where C1, C2 and L are usually 6, 7.5 and 1, respectively.
You can see that an expression like this can become hard to be expressed using mathematical operators such as the ones used to calculate a normalized difference. To implement more complex mathematical expressions, consider using the .expression()
method for a ee.Image
object. the first argument to .expression()
is the textual representation (string) of the math operation, the second argument is a dictionary where the keys are variable names used in the expression and the values are the image bands to be used in the operation. Using the same ImageL8
as an example, EVI would be defined as:
var EVI = ImageL8.expression(
'2.5 * ((NIR - RED) / (NIR + 6 * RED - 7.5 * BLUE + 1))', {
'NIR': ImageL8.select('SR_B5'),
'RED': ImageL8.select('SR_B4'),
'BLUE': ImageL8.select('SR_B2')
});
The code above is able to regonize operators (+, -, *, /, %, **: Add, Subtract, Multiply, Divide, Modulus, Exponent) and the numbers; everything else is defined as keys in the dictionary.
Can you think of how you can calculate the NDVI using the code structure above?
1.2.2 Compositing and Mosaicking
Compositing is an example of a computation that can be done to a image collection. In general, compositing refers to the process of combining spatially overlapping images into a single image based on an aggregation function while mosaicking usually refers to the assembly of these images to produce a spatially contiguous one. In Earth Engine, when you composite two scenes that do not overlap completely, the resulting composite will be a composited mosaic:
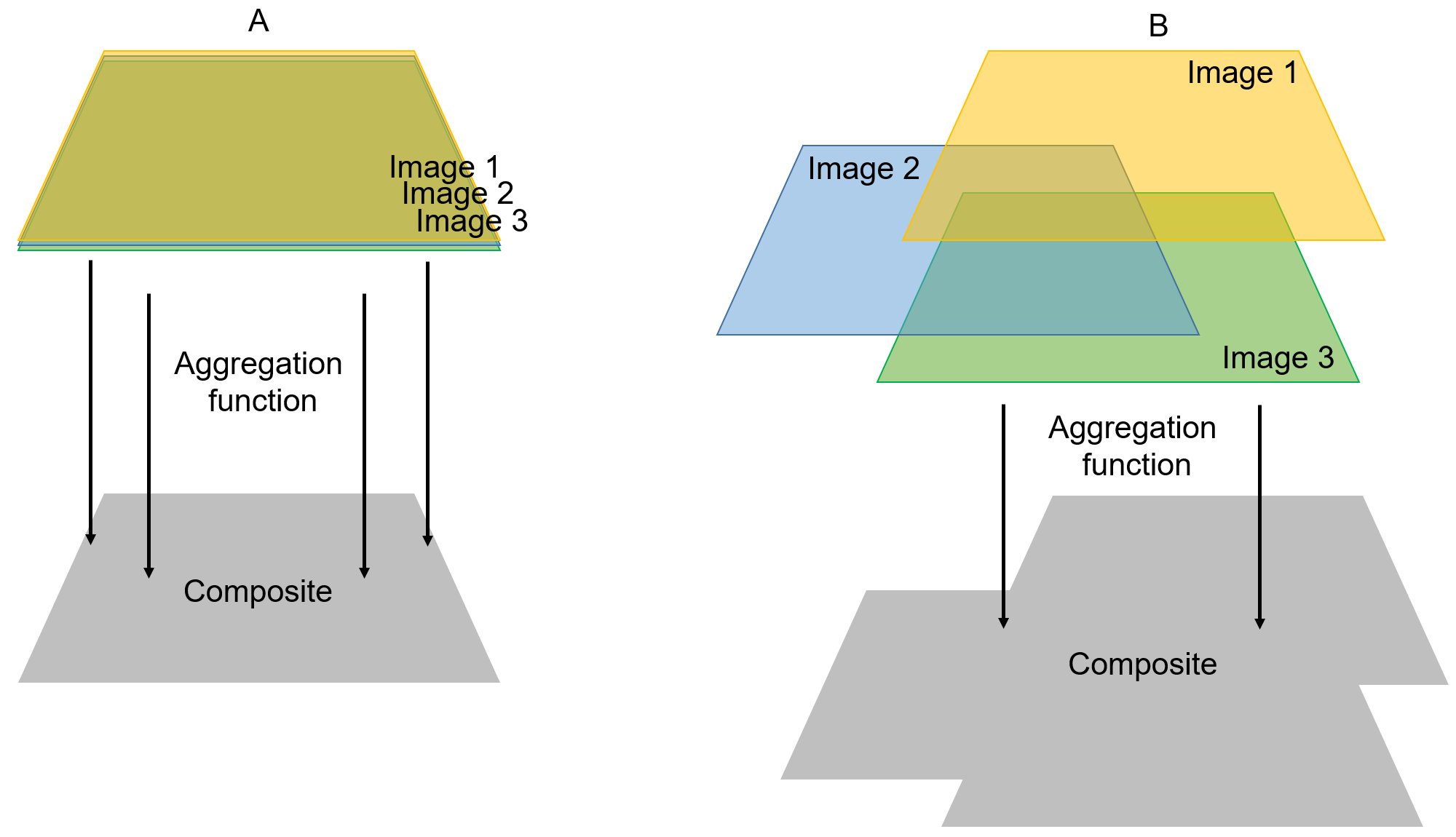
Figure 1.37: Compositing images in Earth Engine. When applying a compositing function to scenes that do not overlap completely (B), the resulting composite will be a spatially contiguous image.
Thefore, in the context of Earth Engine these terms are used interchangeably. We illustrate these concepts with the examples below.
- Example 1: Compositing the same scene (100% overlap)
In this example, we will use collectionFiltered
(a Landsat 8 image collection filtered for Cairo from January to April 2022. See 1.33). There are several aggregation functions that can be used to composite an image collection, most notably .max()
for a maximum value composite and .mendian()
for a median value composite. All these functions will aggregate the values on a per-pixel, per-band basis. In other words, .max()
will select the maximum value of a given pixel for each image band to create the final composite while .median()
will calculate the median value of each band for this pixel.
If you print these composites to the Console you will notice that they are a 19-band single image and no longer a collection with 13 images with 19 bands each!
Add these composites to the Map Editor to investigate their differences:
Map.addLayer(compositeMax, {bands: ['SR_B4', 'SR_B3', 'SR_B2'], min:8000, max:30000}, 'Composite Max');
Map.addLayer(compositeMedian, {bands: ['SR_B4', 'SR_B3', 'SR_B2'], min:8000, max:30000}, 'Composite Median');
Question: What is the most evident difference between these composites?
Answer: The max value composite is mostly covered by clouds. Clouds and snow are very reflective and will always have high reflectance values for the regions of the electromagnetic spectrum covered by the Landsat spectral bands. Therefore, a maximum value composite will likely highlight these features - which is not desireable in most cases.
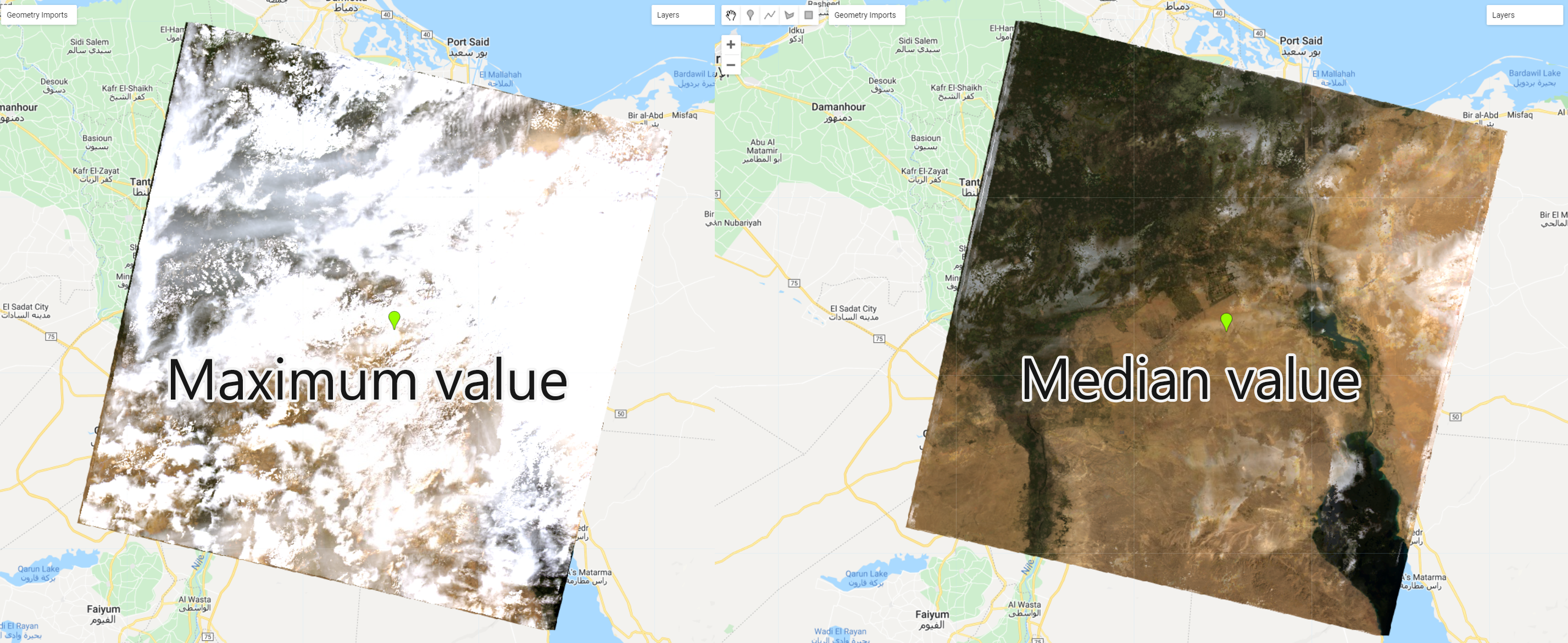
Figure 1.38: Maximum and median value composites. Clouds are evident in the maximum value composite due to their high reflectance values.
Although there are different methods for compositing, the median composite method is the state of the art in Google Earth Engine and has been applied to a multitude of studies using multitemporal remote sensing data.
- Example 2: Compositing (mosaicking) different scenes
Consider the need to composite (mosaic) four different Landsat scenes at different locations. For that we will edit the location parameter from .filterBounds()
to include extra Landsat scenes from different locations. Use the information from figures 1.31 and 1.32 to create a geometry covering a larger area. Remember that .filterBounds()
will filter an image collection to all the scenes that intercept the boundaries of a geometry, whether it is a line, point mark or polygon.
Rename this as ‘aoi2’. For this example, we will use the polygon tool to draw a large polygon over a large area in Egypt.
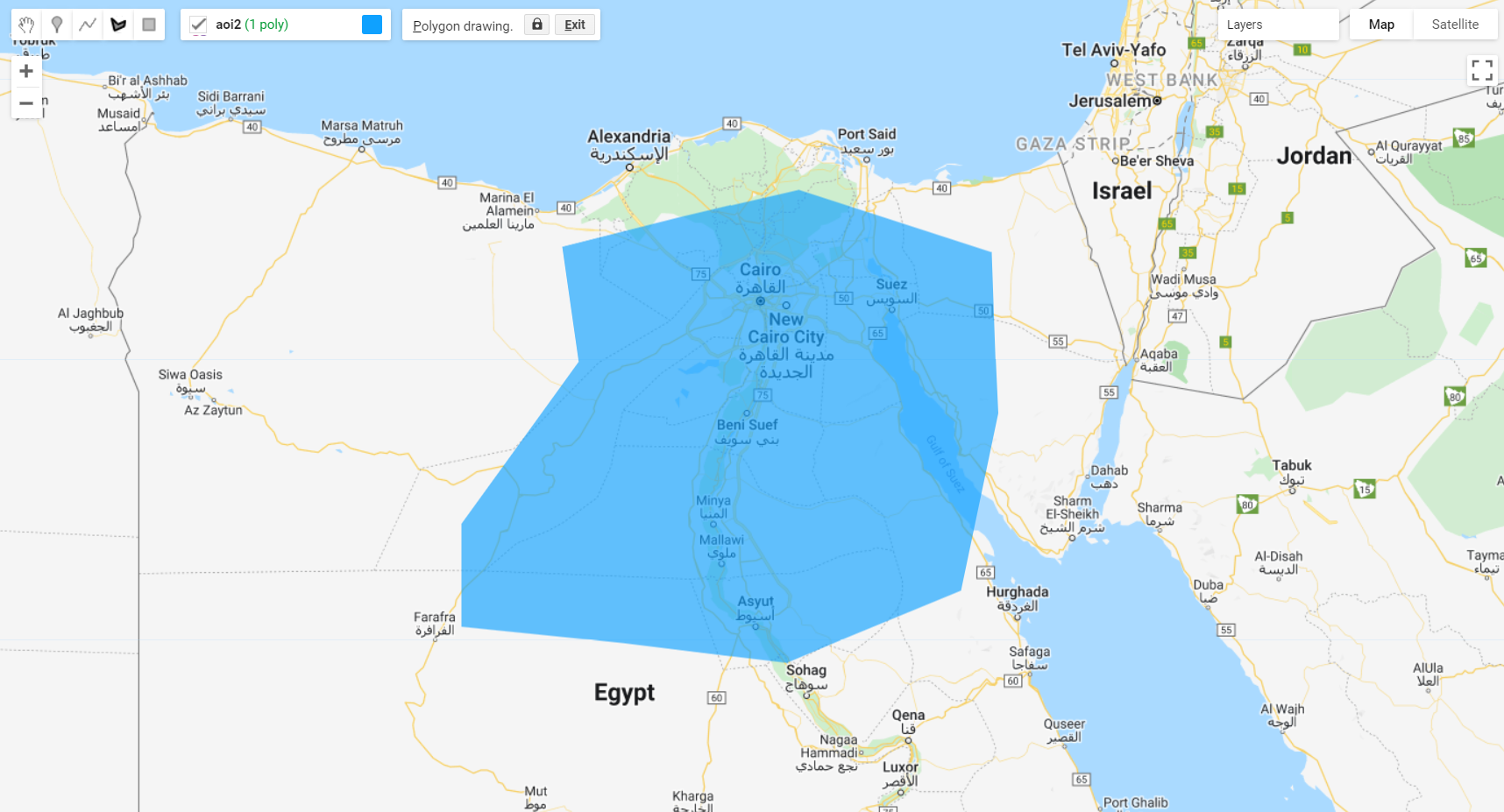
Figure 1.39: Area of interest (aoi) for filtering image collection.
We will use this geometry to filter collection
to this new spatial filter aoi2
:
var collectionFiltered2 = collection.filterBounds(aoi2).filterDate(startDate,endDate);
print(collectionFiltered2);
Note that if you print collectionFiltered2
to the
Console you will notice that it includes more images
than when we filtered for a single location using a point geometry.
Using the same compositing functions described earlier in this section, we will composite this new collection to two new composites. Also, we will use the .mosaic()
method as a comparison. This method composites overlapping images according to their order in the collection (last on top):
var compositeMax2 = collectionFiltered2.max();
var compositeMedian2 = collectionFiltered2.median();
var mosaic = collectionFiltered2.mosaic();
Map.addLayer(compositeMax2, {bands: ['SR_B4', 'SR_B3', 'SR_B2'], min:8000, max:30000}, 'Composite Max 2');
Map.addLayer(compositeMedian2, {bands: ['SR_B4', 'SR_B3', 'SR_B2'], min:8000, max:30000}, 'Composite Median 2');
Map.addLayer(mosaic, {bands: ['SR_B4', 'SR_B3', 'SR_B2'], min:8000, max:30000}, 'Mosaic');
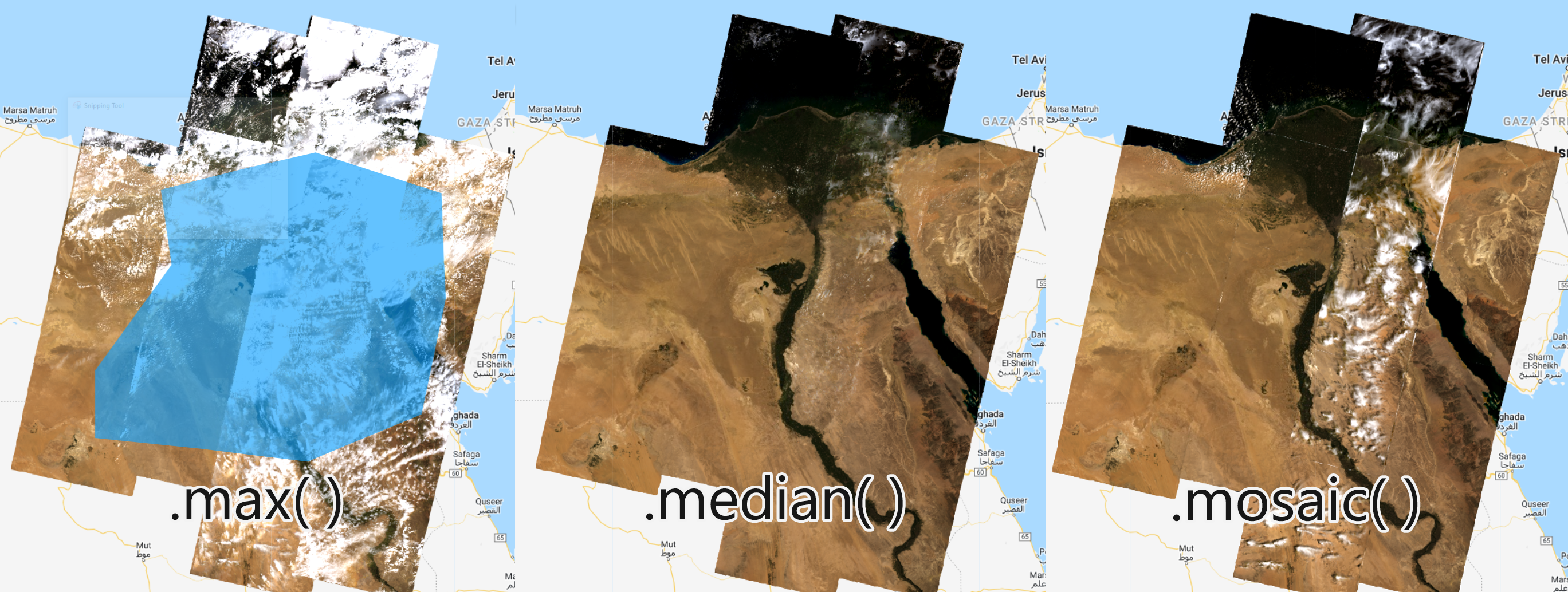
Figure 1.40: Composites of an image collection filtered by the bounds of aoi2
.
Whether you are compositing using an aggregation function or mosaicking with .mosaic()
the result will be similar in respect to the output type (i.e. an ee.Image object) and spatial extent. Thus, explaining why compositing and mosaicking are used interchangeably in Earth Engine.
Suppose you are only interested in the composite within the bounds of your area of interest. The method .clip()
of an ee.Image()
allows you to clip (or cut) any image to the shape of a geometry. We will use compositeMedian2
and aoi2
as an example:
var compositeClipped = compositeMedian2.clip(aoi2);
Map.addLayer(compositeClipped, {bands: ['SR_B4', 'SR_B3', 'SR_B2'], min:8000, max:30000}, 'Composite Median 2 clipped');
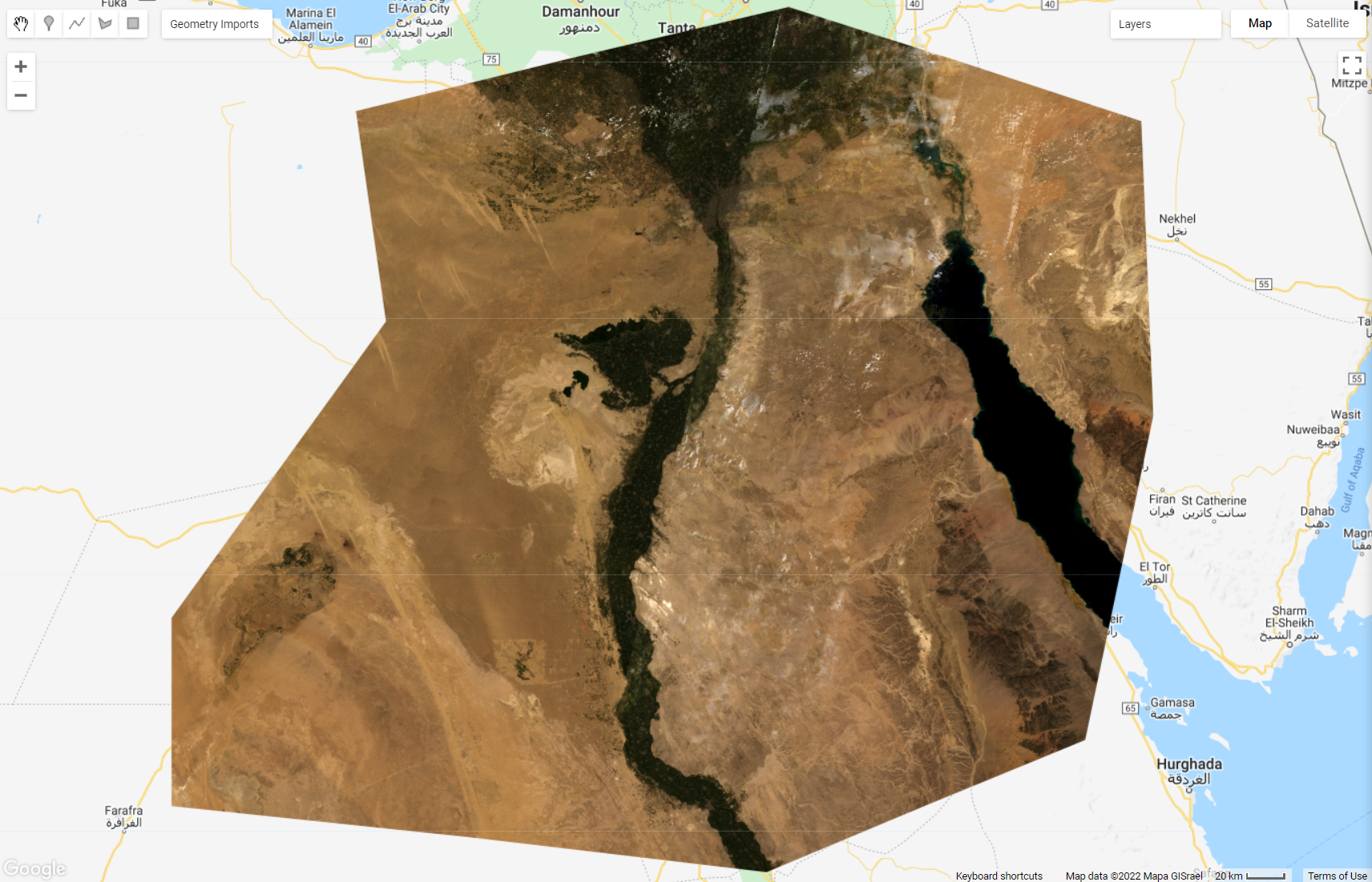
Figure 1.41: Median composite clipped by the extent of aoi2
.
You can only clip an ee.Image() object, never an image collection!
Reflectance is the ratio of the amount of light leaving a target to the amount of light striking the target. It has no units.