Chapter2 PART 2 - Advanced Google Earth Engine
2.1 Advanced Image Manipulation: Pre-classification
In this section we will provide functions and lines of code to assist the user to prepare, process and analyze Landsat 8 surface reflectance data for land cover classification purposes.
2.1.1 Cloud and cloud shadow masking
This sub-section demonstrates one way of masking clouds and cloud shadow pixels from Landsat 8 Surface Reflectance Collection 2 data based on file metadata. You may have noticed when exploring Landsat images in the Console tab that these images have a band called QA_PIXEL
or Quality Assessment band. Briefly, this band contains values that represent bit-packed combinations of surface, atmospheric, and sensor conditions that can affect the overall usefulness of a given pixel. One of the many bits represented in this band is cloud (bit 3) and cloud shadow (bit 4):
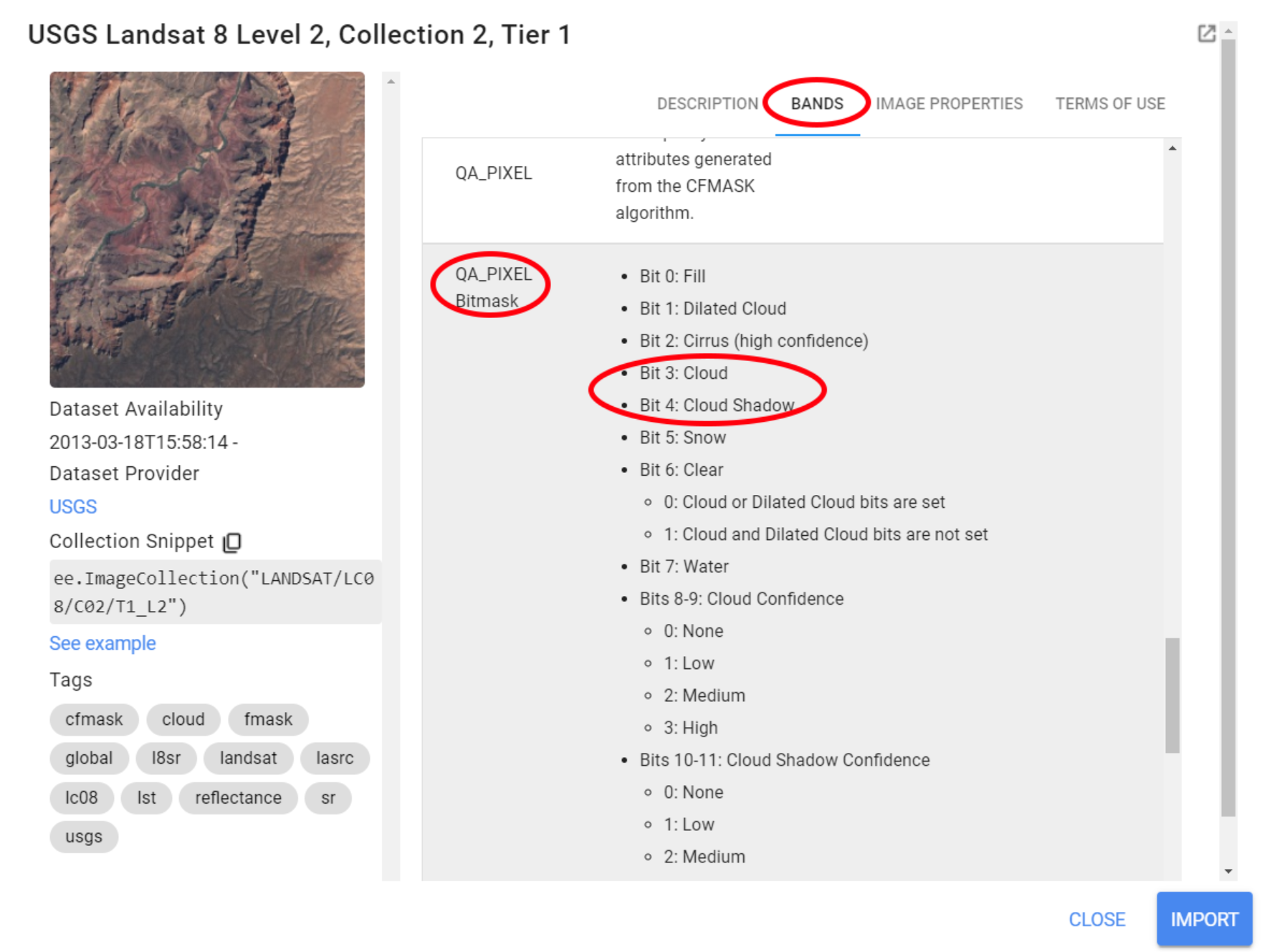
Figure 2.1: Landsat’s Quality Assessment (QA) band.
In essence, these values indicate which pixels might be affected by surface conditions such as cloud contamination. Therefore, this band can be used to construct filters to mask (or remove) pixels flagged as ‘affected’ by that condition. Here, we will provide a function for masking clouds and cloud shadow from Landsat 8 images based on the information stored in the Quality Assessment band. This function was created based on the documentation available for Landsat 8. Values for pixel bit and pixel band were found here.
The cloud masking function is as follows:
function maskClouds(image) {
var cloudShadowBitMask = ee.Number(2).pow(3).int();
var cloudsBitMask = ee.Number(2).pow(4).int();
var QA = image.select('QA_PIXEL');
var mask = QA.bitwiseAnd(cloudShadowBitMask).eq(0)
.and(QA.bitwiseAnd(cloudsBitMask).eq(0));
return image.updateMask(mask).divide(100000).select("SR_B[0-9]*").copyProperties(image, ["system:time_start"]);
}
Do not worry about the new methods within this function. What you need to know is that this function was constructed in a way that we are selecting the QA_PIXEL
band from the Landsat image and creating a mask where only pixels flagged to 0 (indicating clear conditions) for bits 3 and 4 will be included; The function will return image
- in this case the Landsat 8 image - masked for pixels that are not flagged 0 for bits 3 and 4. The .divide()
was used simply to scale the band values to [0,1] and .select()
to only select the spectral bands from Landsat (SR_B1-9). These steps are not necessary for the cloud masking to work! However, we can add these extra steps to further improve our image collection.
Now, to apply a function to every image in an image collection, we use the .map()
method from the ee.ImageCollection()
object. You can refer to the Docs tab for more information on the methods available for ee.ImageCollection()
. The only argument to map()
is a function which takes one single parameter: an ee.Image()
. Using .map(maskClouds)
over an image collection will result in a collection where every image is masked for clouds and cloud shadows.
To illustrate this, we will apply maskClouds
over collection
(created in the previous chapter) along with the same temporal and spatial filters to recreate collectionFiltered
. Feel free to use your previous chapter’s script and simply add .map()
to the collections using the code below as an example:
var collection = ee.ImageCollection('LANDSAT/LC08/C02/T1_L2');
var startDate = '2022-01-01';
var endDate = '2022-04-30';
var collectionFilteredMasked = collection.filterBounds(aoi)
.filterDate(startDate,endDate)
.map(maskClouds);
Now, we will create a max value composite and compare it to the previous composite created with an image collection that was not masked for clouds and cloud shadows:
var compositeMax = collectionFilteredMasked.max();
Map.addLayer(compositeMax, {bands: ['SR_B4', 'SR_B3', 'SR_B2'], min:0, max:0.25}, 'Composite Max Value');
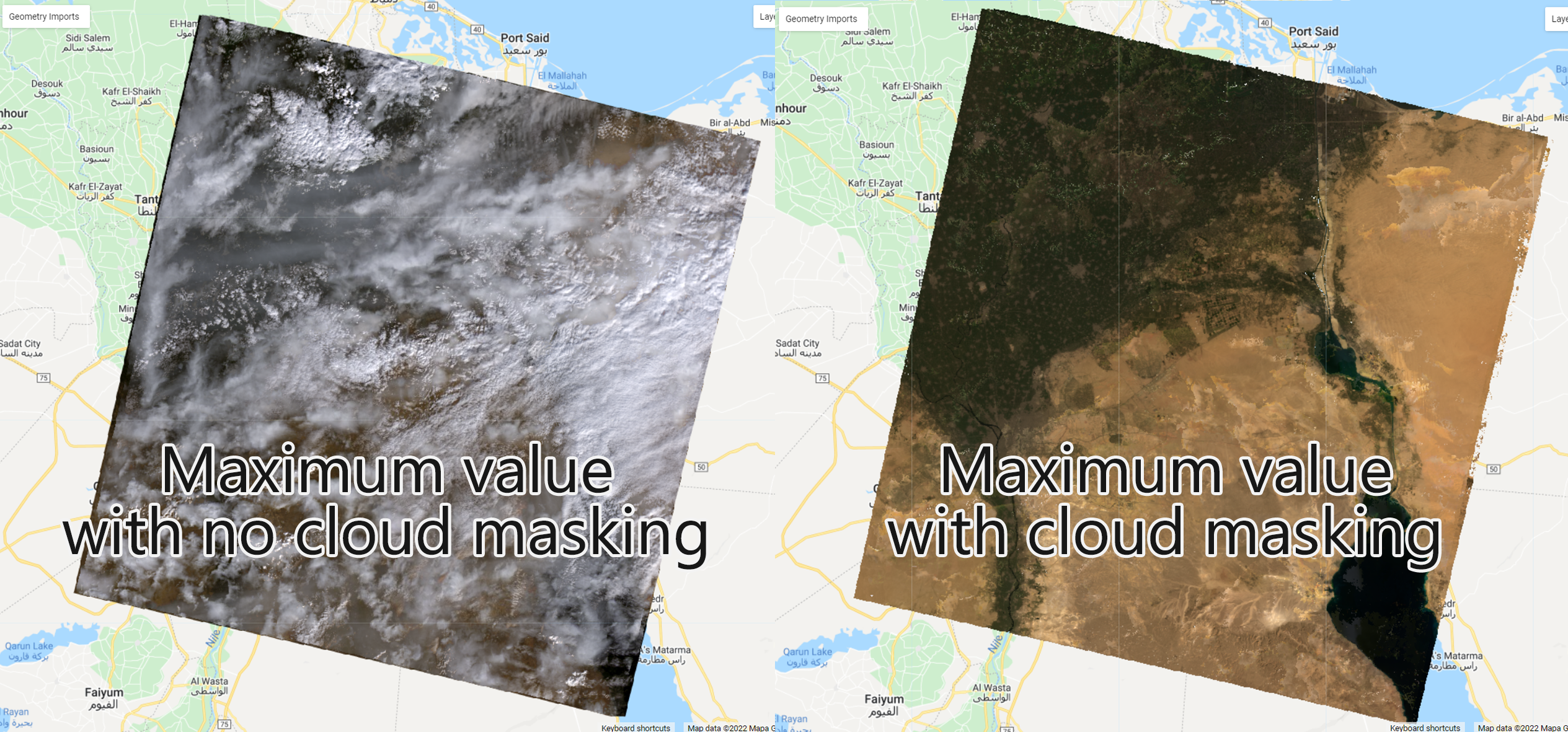
Figure 2.2: A maximum value composite created with an image collection masked by clouds compared to a composite created using an unmasked collection.
As mentioned in the previous chapter, a maximum value composite will likely highlight clouds as they are very reflective. However, by removing the cloud pixels from the images based on the Quality Assessment (QA) band information, the resulting maximum value composite greatly improves.
2.1.2 Spectral indices
In the previous sub-section, we presented a function to mask cloud and cloud shadows from Landsat image collections. A function can also be created to perform band math to every image in an image collection. Building on the concepts of vegetation indices presented on the previous chapter, we will create a function that will calculate several vegetation indices for Landsat 8 images. You will recognize the NDVI and EVI indices from the previous chapters. This function includes other commonly used spectral indices to highlight features like open water, moisture, vegetation and soil:
- NDVI - Normalized Difference Vegetation Index;
- NBR - Normalized Burn Ratio;
- NDMI - Normalized Difference Mangrove Index;
- MNDWI - Modified Normalized Difference Water Index;
- SR - Simple Ratio;
- BI - Bare soil Index;
- GCVI - Green Chlorophyll Vegetation Index;
- EVI - Enhanced Vegetation Index, and;
- MSAVI - Modified Soil-Adjusted Vegetation Index.
function addIndices(image) {
var ndvi = image.normalizedDifference(['SR_B5','SR_B4']).rename('NDVI');
var nbr = image.normalizedDifference(['SR_B5','SR_B7']).rename('NBR');
var ndmi = image.normalizedDifference(['SR_B7','SR_B3']).rename('NDMI');
var mndwi = image.normalizedDifference(['SR_B3','SR_B6']).rename('MNDWI');
var sr = image.select('SR_B5').divide(image.select('SR_B4')).rename('SR');
var bare = image.normalizedDifference(['SR_B6','SR_B7']).rename('BI');
var gcvi = image.expression('(NIR/GREEN)-1',{
'NIR':image.select('SR_B5'),
'GREEN':image.select('SR_B3')
}).rename('GCVI');
var evi = image.expression(
'2.5 * ((NIR-RED) / (NIR + 6 * RED - 7.5* SR_BLUE +1))', {
'NIR':image.select('SR_B5'),
'RED':image.select('SR_B4'),
'SR_BLUE':image.select('SR_B2')
}).rename('EVI');
var msavi = image.expression(
'(2 * NIR + 1 - sqrt(pow((2 * NIR + 1), 2) - 8 * (NIR - RED)) ) / 2', {
'NIR': image.select('SR_B5'),
'RED': image.select('SR_B4')}
).rename('MSAVI');
return image
.addBands(ndvi)
.addBands(nbr)
.addBands(ndmi)
.addBands(mndwi)
.addBands(sr)
.addBands(evi)
.addBands(msavi)
.addBands(gcvi)
.addBands(bare);
}
The .addBands()
method is used to include an ee.Image()
as a band to an existing image. Therefore, this function will calculate each index and include them as extra bands to every image in the image collection.
As in the previous sub-section, we can use .map()
to map this function over collection
:
var collectionFilteredwithIndex = collection.filterBounds(aoi)
.filterDate(startDate,endDate)
.map(maskClouds)
.map (addIndices);
Then we will create median composite based on this new collection. We will add it to the Map Editor and will use the Inspector tab to explore its band values at a given location:
var compositeMedian = collectionFilteredwithIndex.median();
Map.addLayer(compositeMedian, {bands: ['SR_B4', 'SR_B3', 'SR_B2'], min:0, max:0.25}, 'Composite Median');
You can toggle the data view from chart to values with the chart/value view button:
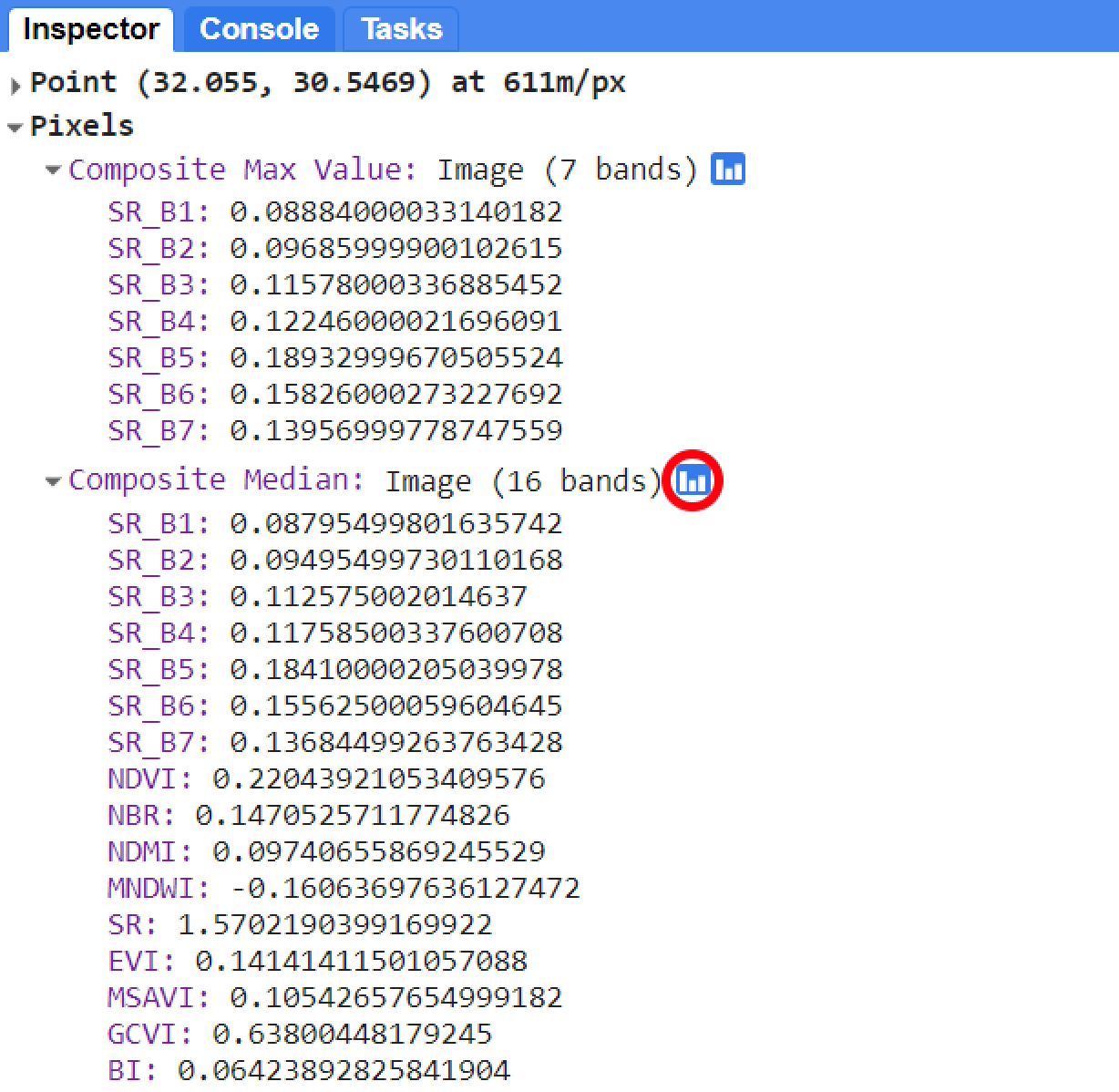
Figure 2.3: A median composite created from the collectionFilteredwithIndex
image collection. The function addIndices
was used to calculate each index and add them as separate bands to every image in the collection. In this example, the spectral bands and spectral index values for a pixel at an arbitrary location within compositeMedian
. You can toggle between the chart view and value view using the chart button (red circle).
2.2 Supervised Classification using Random Forest
As seen in Part 1, Interpretation and Analysis is one of the building blocks of any remote sensing system (see 1.1). Image classification is one of the many methods of image processing and it is the sole focus of this training material. So far, you have learned the basics of Java Script and Google Earth Engine and some pre-processing steps to perform an image classification using the Random Forest (RF) Classifier. Briefly, classifier is an ensemble of classification trees, where each tree contributes with a single vote for the assignment of the most frequent class to the input data. Different from Decision Trees, which use the best predictive variables at the split, RF uses a random subset of the predictive variables. To illustrate this concept, we will use a rather simplistic example:
Suppose you were given this list of attributes from three edible fruits:
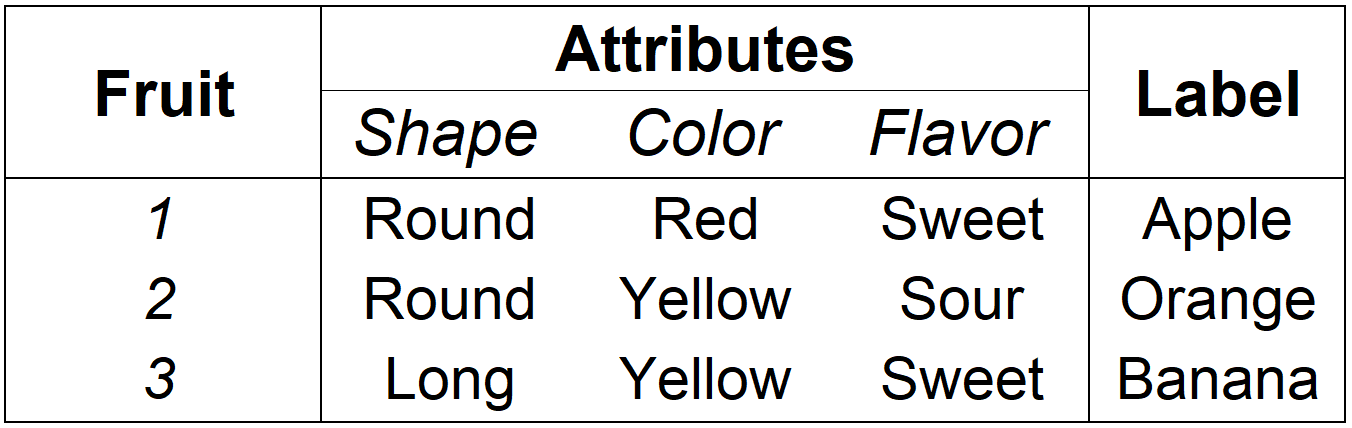
Figure 2.4: Simplistic list of predictive variables from three types of fruits.
A random forest classifier can be created (or trained) using the sample above as a training sample set:
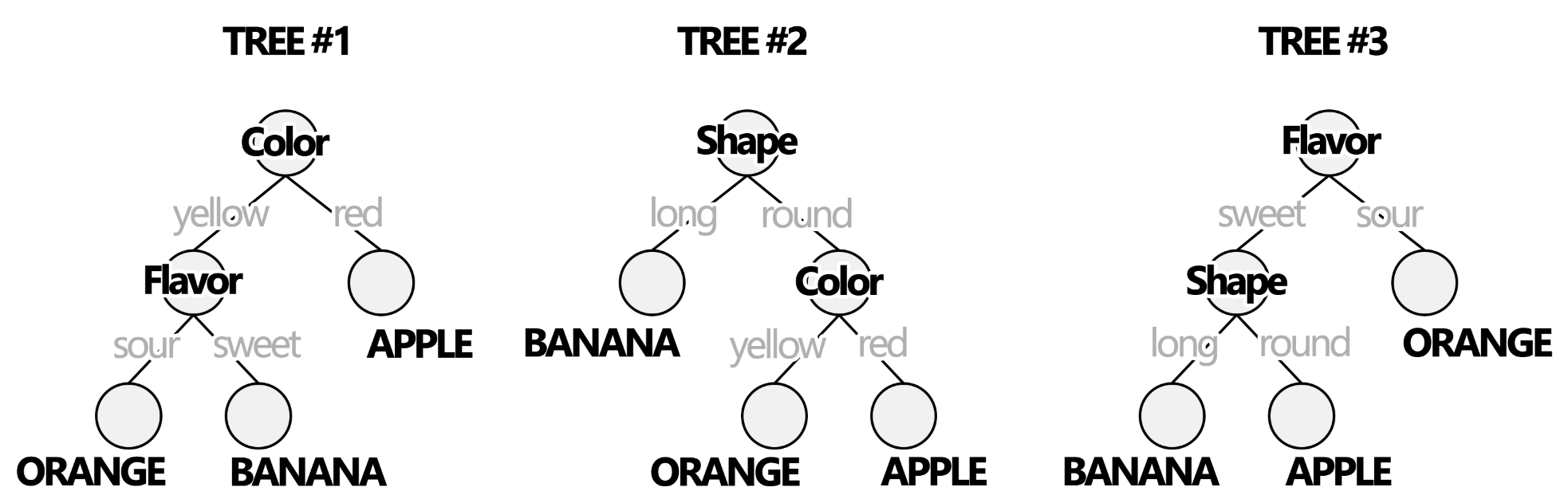
Figure 2.5: A Machine Learning Random Forest Classifier with 3 trees trained with the sample set above. Note that algorithm was able to ‘learn’ about each fruit separate them based on their attributes.
You can test this classifier and its accuracy using a testing sample set. A testing set is usually a subset of the training set that will be used only for testing the performance of the trained classifier. These testing samples will be run through each tree of the ensemble and the accuracy of the classifier will be based on whether or not it was able to classify that sample correctly:
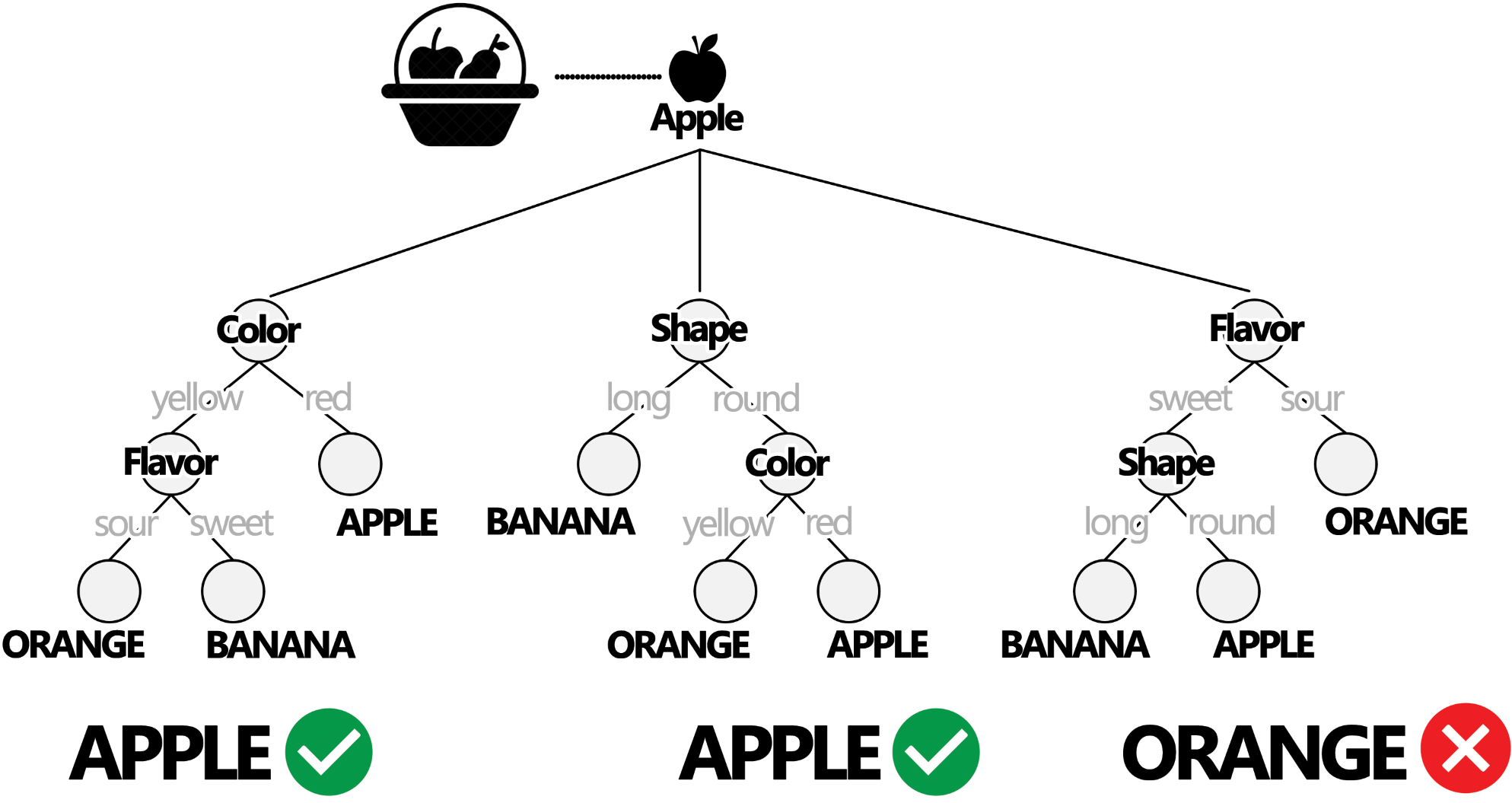
Figure 2.6: Testing a trained Random Forest Classifier. A testing sample is used to assess whether or not the classifier is able to correctly classify them. In this example, this sample was correctly classified by 2 out of the 3 trees in the ensenble.
You can then classify unlabeled samples using the trained classfier. The final classification of a sample is based upon the majority of the votes of the trees in the ensemble:
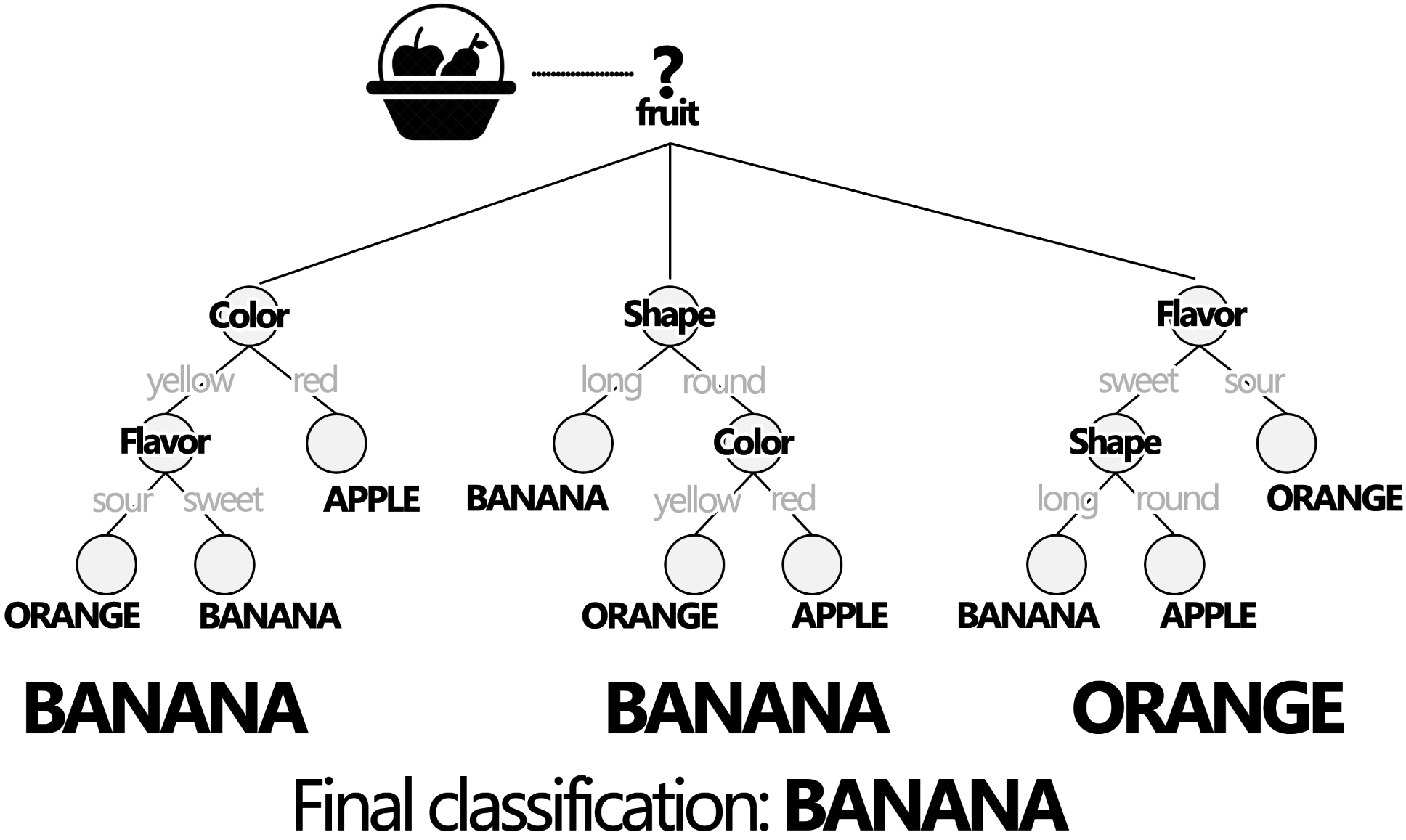
Figure 2.7: The trained RF classifier is used to classify an unlabeled fruit sample. In this case, the majority of the votes (2 out of 3) was BANANA. Therefore, the final classification of this sample is BANANA.
Even though this is a rather simplistic example, it can be easily translated into a land cover classification context: Instead of fruits, each unit being classified is a Landsat image pixel. Each pixel from each land cover class will contain different spectral attributes. These spectral attributes will be used at each branch of the trees in the ensemble. We can then used a trained Random Forest classifier to label hundreds of thousands Landsat pixels into different land cover classes. The RF is one of the most used and robust classifiers and it is fully implemented in GEE. In the following examples we will present the steps for land cover classification using the RF classifier and present some basic analysis that can be done using the classification output.
2.2.1 Example 1: Land cover classification of Greater Cairo and Giza area, Egypt - Year 2022
The general steps for an image classification process with Landsat image is:
To use a cloud-masking function to mask clouds on Landsat 8 Imagery;
To calculate spectral indices that will be used as predictors for the Random Forest;
To produce a cloud-free composite mosaic using the median reducer, and;
To select training samples;
To classify the cloud-free composite mosaic of Landsat 8 scenes using Random Forest.
In this example we will classify the median composite (compositeMedian
) created in the previous section. You can find the code for creating it here.
So far, we have covered the steps a, b and c.
- Training sample selection
For this example, let’s classify the ‘composite’ into four classes: water, agricultural land, sand and bare areas and urbanization. The first step is to create the training samples set to use into the Random Forest Model.
Step 1 - In the Geometry Imports, click +new layer and make four sets of geometries, each set will represent samples from the classes ‘water’, ‘cropland’, ‘sand’, and ‘urban’.
Figure 2.8: Geometry sets to hold samples for each of the four classes.
Step 2 - For each geometry in the list, click on the settings icon⚙: name them accordingly using the ‘Name’ box, choose a color for it using the color picker and import each geometry as FeatureCollection. Add a property called landcover by clicking on the + Property and set a consecutive integer starting from 0 or 1 for each of the classes. You should achieve something similar to this:
Figure 2.9: Geometries used to create the training sample sets.
Your Geometry Imports should look like this:
Figure 2.10: Geometry Imports after creating geometry sets to hold training samples
Start selecting samples by clicking on the ‘Water’ geometry in the Geometry Imports. Choose the point drawing tool and place some points along the River Nile:
Take advantage of the high resolution imagery to help you select samples for each class. You can toggle in between map and Google Earth imagery by using the buttons Map and Satellite in the upper right corner of the Map Editor. You can also toggle the Landsat composite ON and OFF by using the layer manager.
Instead of single points (i.e pixels), we can also use polygons containing a variable number of relatively homogenous pixels of a given land cover class. Switch to the polygon drawing tool and draw a few polygons over the River Nile:
Figure 2.11: Sample points and polygons for the Water class
Once you finish selecting samples, click the Exit button on the polygon editor dialogue box . Repeat the process for each of the other class. Make sure you select samples that are representative of each land cover class by selecting points and polygons of homogenous pixels.
Figure 2.12: Example of sample points and polygons for ‘Water’ (blue), ‘Cropland’ (yellow), ‘Urban’ (red) and ‘Sand’ (pink). Each pixel within the polygons will be used as training inputs for the RF classifier.
After selecting the samples, we will merge all the geometries together into an object var classes using the .merge
method of the ee.FeatureCollection() object
:
- Sample sets
In this section, we will create the training (and testing) sample sets to be used in the classification with Random Forest. First, we will select the predictors to assign to each sample point in the sample sets. For this example, we will create a list ‘bands’ with the names of three spectral bands ('SR_B4'
,'SR_B5'
,'SR_B6'
) and the spectral indices ('NDVI'
,'NBR'
,'MNDWI'
,'SR'
,'GCVI'
and 'MSAVI'
).
Next, we will sample the Landsat pixels by overlaying the geometries with the composite using .sampleRegions()
. The main arguments of this method is the image to sample (in this case, compositeMedian
), the regions to sample over (in this case, classes
) and the list of properties to copy from each geometry (in this case landcover):
var samples = compositeMedian.select(bands).sampleRegions({
collection: classes,
properties: ['landcover'],
scale: 30
}).randomColumn('random');
In the samples
object we have just created, each sample will include a column with the values from the list bands
inherited from the compositeMedian
and a column with their respective class label. Optionally, you can perform an accuracy assessment of the classifier by taking advantage of the identifiers assigned to the samples by the .randomColumn('random')
within samples
. This method adds a column to the feature collection populated with random numbers in the range of 0 to 1.
For this example, we will randomly partition the sample set into training
(80% of the samples) and testing
(20% of the samples) samples by filtering the samples by its random number column using the lower (.lt
) and greater than or equal (.gte
) filters:
var split = 0.8;
var training = samples.filter(ee.Filter.lt('random', split));
var testing = samples.filter(ee.Filter.gte('random', split));
For your information, you can inspect the size of a ee.FeatureCollection
using the .aggregate_count()
method. This method only takes a property of the feature collection being counted as its argument. In this case, we have a property called ‘landcover’ in our feature collection.
.aggregate_count()
It is a useful tool to extract the number of features on your sample set:
print('Samples n =', samples.aggregate_count('landcover'));
print('Training n =', training.aggregate_count('landcover'));
print('Testing n =', testing.aggregate_count('landcover'));
If a feature collection do not have a property defined, you can still
count its feature by using ‘.all’
as an argument for
.aggregate_count()
.
Using the split value above, roughtly 80% of the features in samples
will be training
and 20% will be in testing
:
Figure 2.13: Sample sets size for this example. Note that the number will vary based on the number of geometries (polygons and points) and the sample split value.
- Classification
Next, we will train a Random Forest classifier using the training sample set training
. The Random Forest classifier ee.Classifier.smileRandomForest
has several user-defined parameters. However, two of them are the most usually defined: the number of trees in the ensemble (a.k.a ‘forest’) and the number of predictors to randomly tested at each tree. Predictors, in this case, are the spectral bands and spectral indices associated to each training sample. If unspecified, it uses the square root of the number of predictors.
Higher number of trees and number of predictors tested at each node do not mean better performance and overall accuracy.
For this example, we will use 200
trees and 8
randomly selected predictors to use in each tree and train Random Forest model called ‘classifier’ using the .train()
method on the .ee.Classifier.smileRandomForest()
:
var classifier = ee.Classifier.smileRandomForest(100,5).train({
features: training,
classProperty: 'landcover',
inputProperties: bands
});
In the features
argument of the code above, make sure to select the predictors you want to use from the sample set (in this example, training
) to train the model. In this case we are providing all of them. However, if you want to select a particular group of variables, you can use .select(['variableName1','variableName2',..])
. Also, you must include the class property (classProperty
) in which the class label is stored (in this example 'landcover'
).
You can test the accuracy of classifier by classifying the testing
samples using .classify()
method. Then, you can compute a 2D error matrix for the classified testing samples using .errorMatrix()
. This method will output an ee.ConfusionMatrix()
object by comparing the two columns of the classified testing samples: one containing the actual labels (in our case, the property called ‘landcover’), and one containing predicted values by the classifier (which defaults to ‘classification’). Finally, you can use .accuracy()
method of the ee.ConfusionMatrix()
object to compute the overall accuracy of the classifier:
var validation = testing.classify(classifier);
var testAccuracy = validation.errorMatrix('landcover', 'classification');
print('Validation error matrix RF: ', testAccuracy);
print('Validation overall accuracy RF in %: ', testAccuracy.accuracy().multiply(100));
As explained earlier, the trained classifier can be used to classify the compositeMedian
. Google Earth Engine will run each pixel through each of the 200 trees in the classifier and assing it the label which had the majority of votes among all the tree:
For visualization of the resulting classification output, we will create a color palette object paletteMAP
with a list of colors for each class of the map. The order of the colors in the palette will follow the order of the classes:
var paletteMAP = [
'#0040ff', // Water (Class value 0)
'#00ab0c', // Croplands / Cultivated Areas (Class value 1)
'#fbf2ad', // Sand and bare areas (Class value 2)
'#878587' // Built-up and Urban Areas (Class value 3)
];
Finally, we will add classification
to the map using Map.addLayer()
: use min: 0
(first class), max: 3
(last class), add the color palette paletteMAP
and a name for the layer:
After several seconds, you should see something similar to the figure below:
Figure 2.14: Random Forest classification output for 2022.
As an optional step, you can export your classification output as a Google Earth Engine asset. You can save geospatial datasets and analysis outputs into your Google Earth Engine account through the Assets tab and the left side of the code Editor.
Figure 2.15: Assets manager. Your Google Earth Engine account will be able to host around 200GB worth of assets. Externally imported assets as well as datasets exported from Earth Engine scripts will be found here.
You can also upload external datasets into your Google Earth Engine Assets with the NEW button. (See Earth Engine’s Importing Raster Data for instructions on uploading an image to your assets or Importing Table/Shapefile Data for more details).
We will export classification
with the Export.image.toAsset()
method. This method takes a dictionary with several parameters such as:
image
: the image object you want to export;description
: a description to be showing on the Task tab. No spaces allowed;assetId
: A name for your asset. No spaces allowed;scale
: A scale to export;region
: the region or area of yourimage
you want to export. It takes a geometry/ feature collection;maxPixels
: This is somewhat important argument. In order to avoid memory errors, the Earth Engine detault is 1x10e8 pixels. If you do not define your max pixels and your exports exceeds the default, you will get an error.
We will create a geometry ‘region’ to encompass the entire classified scene in order to export the entire area. The code below will export the classification:
Export.image.toAsset({
image: classification,
description: 'ClassificationOutput',
assetId: 'Cairo2022',
scale: 30,
region: region,
maxPixels:1e12
});
Running the code above, will create the exporting task named ClassificationOutput in the Tasks tab.
Figure 2.16: Tasks manager.
Clicking the Run button will start exporting your classification. You can check some of the parameters of the Export.image.toAsset()
one more time before exporting the asset. You are allowed to change some of these parameters at this point. For our example, everything will follow the parameters we defined previously.
Figure 2.17: Tasks manager: Image Export.
The time elapsed of your exporting task will be shown in the Tasks tab.
Figure 2.18: Once a task has started the time elapsed will be shown in the Tasks tab.
I may take several minutes to export your classification image to your assets. Once your task is completed, it will be shown at the Task tab as:
Figure 2.19: Exported asset.
Finally, your export will be available as an Earth Engine asset:
Figure 2.20: Assets will be available through the Assets tab. If they are not showing, try refreshing the folder with the refresh button.
2.2.2 Example 2: 2015 - 2022 Map-to-Map change of greater Cairo and Giza area, Egypt
In this example, we will use the codes from Example 1 to create a land cover classification for the year 2015 period. Following are the changes to be made on the codes from Example 1:
- The
year
object will have a value of'2015'
; - Repeat the training sample selection to make sure the samples reflect the correct land cover class for the L8 composite in the year 2015.
You can access the edited script for two steps above here.
After following the 2 steps above, you should achieve a similar output as before:
Figure 2.21: Random Forest classification output for 2015.
Export this new classification to you assets. Next, open a new code editor page and add the classification exports to this script by clicking the Import into script button (blue arrow) for both classification assets in the Assets tab:
Figure 2.22: Assets tab with classification maps. Clicking in the Import into script button will add the assets to the script.
Finally, rename each import by clicking on their names at the Imports header:
Figure 2.23: Imports header. You can rename imported assets by clicking on its name.
Alternatively, you can import assets to your scripts by using ee.Image()
and their respective asset directory. For this example, you can use:
var Cairo2015 = ee.Image("users/capacityBuilding/Cairo2015");
var Cairo2022 = ee.Image("users/capacityBuilding/Cairo2022");
Add Cairo2015
and Cairo2022
to the map using the code below:
var paletteMAP = [
'#0040ff', // Water - pixel value = 0
'#00ab0c', // Croplands / Cultivated Areas - pixel value = 1
'#fbf2ad', // Sand and bare areas - pixel value = 2
'#878587', // Built-up and Urban Areas - pixel value = 3
];
Map.addLayer(Cairo2015, {min: 0, max: 3, palette:paletteMAP}, 'Cairo2015');
Map.addLayer(Cairo2022, {min: 0, max: 3, palette:paletteMAP}, 'Cairo2022');
You can examine both layers on the map and try to identify areas where classes have changed in the 7 years period. However, we can use a transition error matrix to quickly quantify these changes. This matrix can be used to assess how much each class has changed and to what they have changed based on sampling design of your choosing. To do that, we will start by stacking both maps Cairo2015
and Cairo20222
into a single object stackedClassifications
and rename Cairo2015 and Cairo2022 as 'before'
and 'later'
, respectively:
Next, we will create a testing sample set with 1000 points for each class using a stratified random sampling design.
In this sampling design each class has the same weight. Therefore, the same number of points will be placed in each class, regardless of its relative extent. This is important to consider as some classes may have very small area extent to be captured by a random sampling design. In this example, water bodies is relatively small compared to the other three land cover classes. Thus, a stratified sampling design will ensure that this class will have the same number of points. We can used .stratifiedSample()
method. This method takes a dictionary with several parameters such as:
numPoints
: Number of points you want to select by each class;classBand
: The classification you want the sample points to inherit the class labels from;scale
: Always remember to set the scale to match Landsat nomimal spatial resolution (30 m)
Always remember to check the Docs tab for more information on the parameters each method takes!
Using the information above, a stratified sample set can be created as following:
var samples = stackedClassifications.stratifiedSample({
numPoints: 1000,
classBand: "before",
scale: 30,
geometries: true
});
Once the points are placed, they will inherit the class label (pixel value) from Cairo2015
('before'
):
We can add the samples to the map using Map.addLayer()
. To colorize geometries, you use the color
paramenter instead of palette
:
Figure 2.24: Stratified samples. 1000 samples were randomly selected within each strata (class).
Next, we will create a transition matrix using the samples above. This matrix will show how many of these 1000 remained the same land cover class 7 years later (Cairo2022
) and how many of them changed to a given class. You can use .accuracy()
to calculate the % of these samples remained unchanged between the two periods:
var transitionMatrix = samples.errorMatrix({
actual: 'before',
predicted: 'later'
});
print('Transition Matrix', transitionMatrix);
print('% of Unchanged Samples: ', transitionMatrix.accuracy().multiply(100));
The matrix will be printed to the Console tab. The code above should produce something similar to figure below:
Figure 2.25: Transition matrix based on the 4000 (1000 for each class) samples.
In the transition matrix above you can draw information about how many samples transitioned to each class from 2015 to 2022. For example, from the 1000 samples of agriculture class (pixel value 1), 4 was classified (or ‘transitioned’) to water, 8 to sand and 53 to urban areas in 2022. 935 of them remained unchanged. Overall, 87% of these samples from all classes remained unchanged.
- Cropland area expansion and conversion in the Nile Delta from 2015-2022
Another quick analysis that can be done with land cover class maps in two points in time is highlighting areas in which a particular class has changed. In this example, we will assess the cultivated/cropland change between the 2015 and 2022. First, we will isolate the agricultural land class (pixel value 1
) from both years into separate objects class2015
and class2022
using the .select()
and .eq()
methods. Secondly, we will calculate the change by simply subtracting both objects:
var class2015 = Cairo2015.select(['classification']).eq(1);
var class2022 = Cairo2022.select(['classification']).eq(1);
var change = class2022.subtract(class2015);
By default, the new objects class2015
and class2022
will have values of 1 where the 'classification'
has the label equals (.eq
) to 1 (agriculture) in the land cover map. This will be the case regardless of the label value. By using the .select()
and .eq()
, the new image object will default to value of 1 where that condition was met. Thus, the object change
will have values of -1
, 0
and 1
, that represents loss/conversion, no change and gains, respectively:
-1
= No crops in 2022 - crops in 2015 (0 - 1 = -1);0
= crops in 2022 - crops in 2015 (1 - 1 = 0);1
= crops in 2022 - no crops in 2015 (1 - 0 = 1)
We will add change
to the map.
var paletteCHANGE = [
'red', // Loss/conversion
'white', // No Change
'green', // Gain/Expansion
];
Map.addLayer(change, {palette: paletteCHANGE}, 'Change 2015-2022');
The resulting change map will look similar to figure below. Green pixels represent ‘gains’ while red pixels represent ‘conversions’:
Figure 2.26: Subset of the 2015-2022 Change Map. Conversion/loss of classifiedagricultural areas.
This code can easily be used to investigate the other classes as well. Simply change the class value within .select(['classification']).eq(classValueHere)
.
In the example below, we changed the value from 1
to 3
to highlight the expansion of urban/built up areas.
Figure 2.27: Subset of the 2015-2022 Change Map. Expansion of the future capital of Egypt, New Cairo City.
You can calculate the area of expansion/conversion by isolating the pixels of gain/loss from the change
object into gain
and loss
. Then, calculate the area of each pixel using ee.Image.pixelArea()
and multiplying by the count of pixels in gain
and loss
using multiply
. The default unit is square meters (m²). You can use .divide()
to transform into square kilometers (.divide(1000000)
) or hectares (.divide(10000)
):
var gainArea = gain.multiply(ee.Image.pixelArea().divide(1000000));
var lossArea = loss.multiply(ee.Image.pixelArea().divide(1000000));
These objects will hold the area calculation for a single pixel. Then, we can use .reduceRegion()
method, from the ee.Image()
container. This will apply a reducer to all the pixels in a specific region. This method will take the reducer
parameter, which will contain the type of mathematical operation you wish to compute for all these pixels. In this case, we will use ee.Reducer.sum()
, to sum up all the single area values for all the pixels within gainArea
and lossArea
:
Make sure to create a geometry around an area of interest in order to calculate the area (extent) for the pixels of a given class within that geometry! In this example, we used a geometry around New Cairo City called ‘AOI’.
var statsgain = gainArea.reduceRegion({
reducer: ee.Reducer.sum(), // Sum of all the area values.
scale: 30, // Landsat scale.
geometry: AOI,
maxPixels: 1e14
});
var statsloss = lossArea.reduceRegion({
reducer: ee.Reducer.sum(),
scale: 30,
geometry: AOI,
maxPixels: 1e14
});
Finally, you can print these values using the code below:
Figure 2.28: Subset of the 2015-2022 Change Map. Expansion of the future capital of Egypt, New Cairo City. The .reduceRegion()
method was used to calculate the extent of gains for the urban/built up class. Finally print()
was used to print the result to the Console tab.
2.3 Post-classification processing
So far you have learned the basics of creating a classification output using a machine learning algorithm such as Random Forest. The objective of this section is to provide useful post-classification steps for corrections and general improvement of a random forest classification output.
2.3.1 Re-classification
Classification outputs often times will need some degree of correction or adjustment. Some of these corrections and adjustments include, for example, the Correction for missclassification erros in specific areas and changing class labels (pixel values) or class order. In this section we will explore the function remap()
. This function maps from input values to output values, represented by two parallel lists: one includes the original number of classes and their value; the other represents which class (or classes) is being remapped and what it is being remapped to.
To ilustrate this concept, consider the following example:
- The previously produced land cover maps include four classes: Water (pixel value = 0), Cropland / Cultivated Areas (pixel value = 1), Sand and bare areas (pixel value = 2) and Built-up and Urban Areas (pixel value = 3) (List 1). If any of these class values needs to be changed, the new value for that class is placed in the List 2, in the position of the class that needs changing (Figure 1).

Figure 2.29: The remap
function for the land cover classification of the Greater Cairo.
To test this function, start by opening a new code editor page and importing one of the classification assets you used in the previous section. In this example, we will importe the latest land cover map from the year 2022 as a variable called ‘Cairo2022’ and we will add it to the map editor using the same color scheme from paletteMAP
used in the previous section:
var Cairo2022 = ee.Image("users/capacityBuilding/Cairo2022");
var paletteMAP = [
'#0040ff', // Water
'#00ab0c', // Croplands / Cultivated Areas
'#fbf2ad', // Sand and bare areas
'#878587', // Built-up and Urban Areas
];
Map.centerObject(Cairo2022);
Map.addLayer(Cairo2022, {min: 0, max: 3, palette:paletteMAP}, 'Cairo2022');
Using Map.centerObject()
will center the map view on a
given object you click the Run button. It is always a
good practice to set the center on your main object so you can always
come back to it! In this example you can center the map view to your
classification object Cairo2022
.
Now, to illustrate the .remap()
method, let’s consider the following scenarios:
*Scenario 1
A new version of the Cairo2022
map where Sand and bare areas (pixel value = 2) and Built-up and Urban Areas (pixel value = 3) switch orders in the final map. In this case, we can use the .remap()
method to change the pixel value of Built-up and Urban Areas to 2 and Sand and bare areas to 3 in their respective position on list 2:

Figure 2.30: Sand (2) and Cities (3) changing orders in the image output using .remap
As a guide, the figure above can be used to create a new variable CairoV1
with the new order for the classes using .remap()
:
Add the CairoV1
to the the map using the original color scheme paletteMAP
:
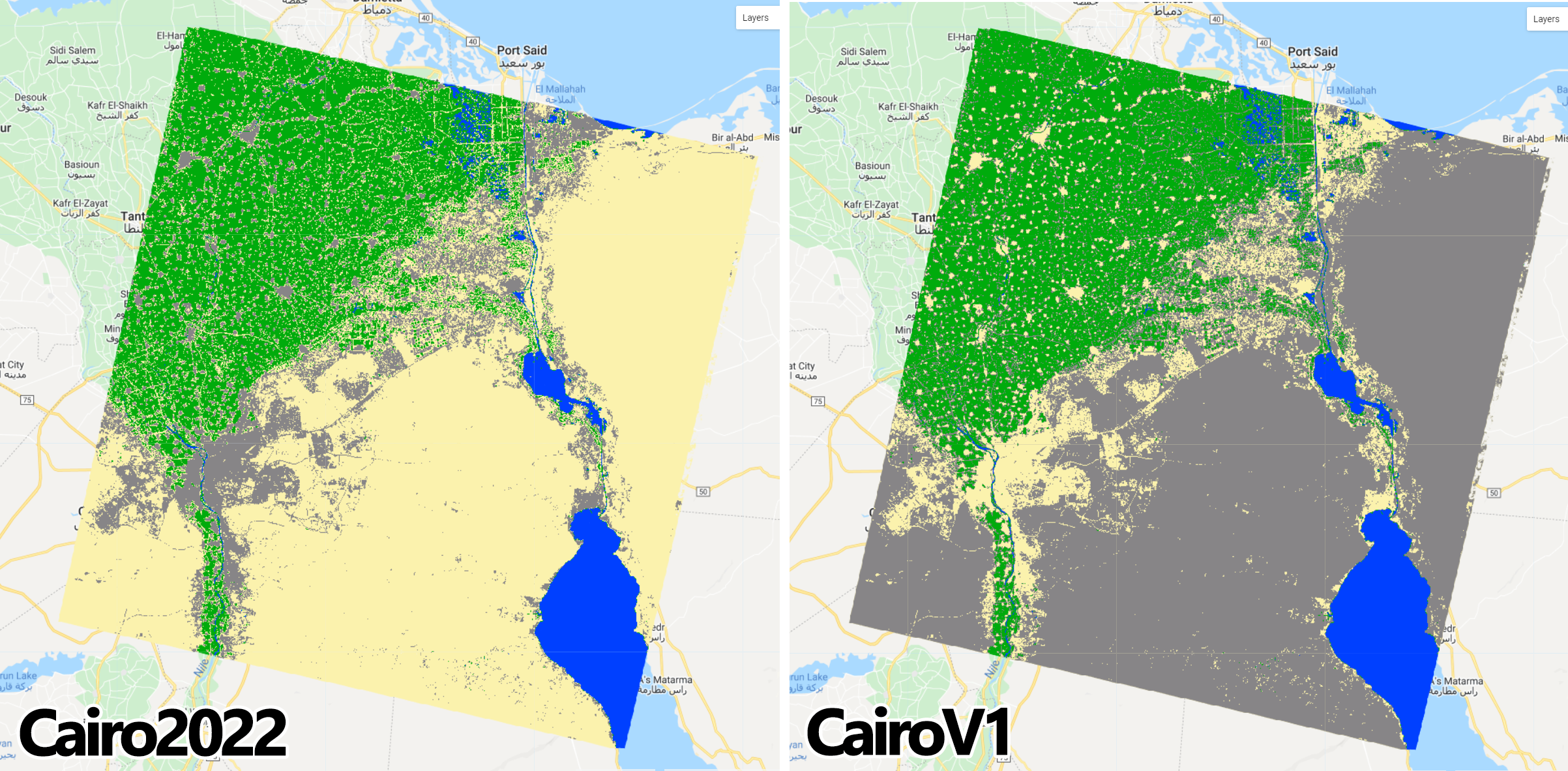
Figure 2.31: Cairo2022
and CairoV1
maps using the original paletteMap
. Note that Sand and bare areas and Built-up and Urban areas switched colors as we switched their order.
*Scenario 2
A new version of the Cairo2022
map where Sand and bare areas (pixel value = 2) and Built-up and Urban Areas (pixel value = 3) are merged into a new class called Barren land and Articicial Surfaces (pixel value = 2). Note that this new class can assume any value when merging, as long as both classes have the same value:

Figure 2.32: Sand and bare areas merging with Built-up and Urban area into a new class value (2). New classes can assume any value when merging, as long as these values are the same for both classes.
For this example, we will keep the value 2 for this new class in a new variable CairoV2
for the new map:
We will add the CairoV2
to the the map using the original color scheme paletteMAP
.
Note that the min
and max
range of values
changed from 0-3 (4 classes) to 0-2 (three classes) in
CairoV2
. You can still maintain the original
min
and max
range of values in this particular
case because Map.addLayer()
would only map the color scheme
to the first three values ofCairov2
(0, 1 and 2). However,
it is always good practice to set the min
and
max
range of values to match the actual number of classes
along with editing the color palette to have the same number of colors
as the number of classes in your map.
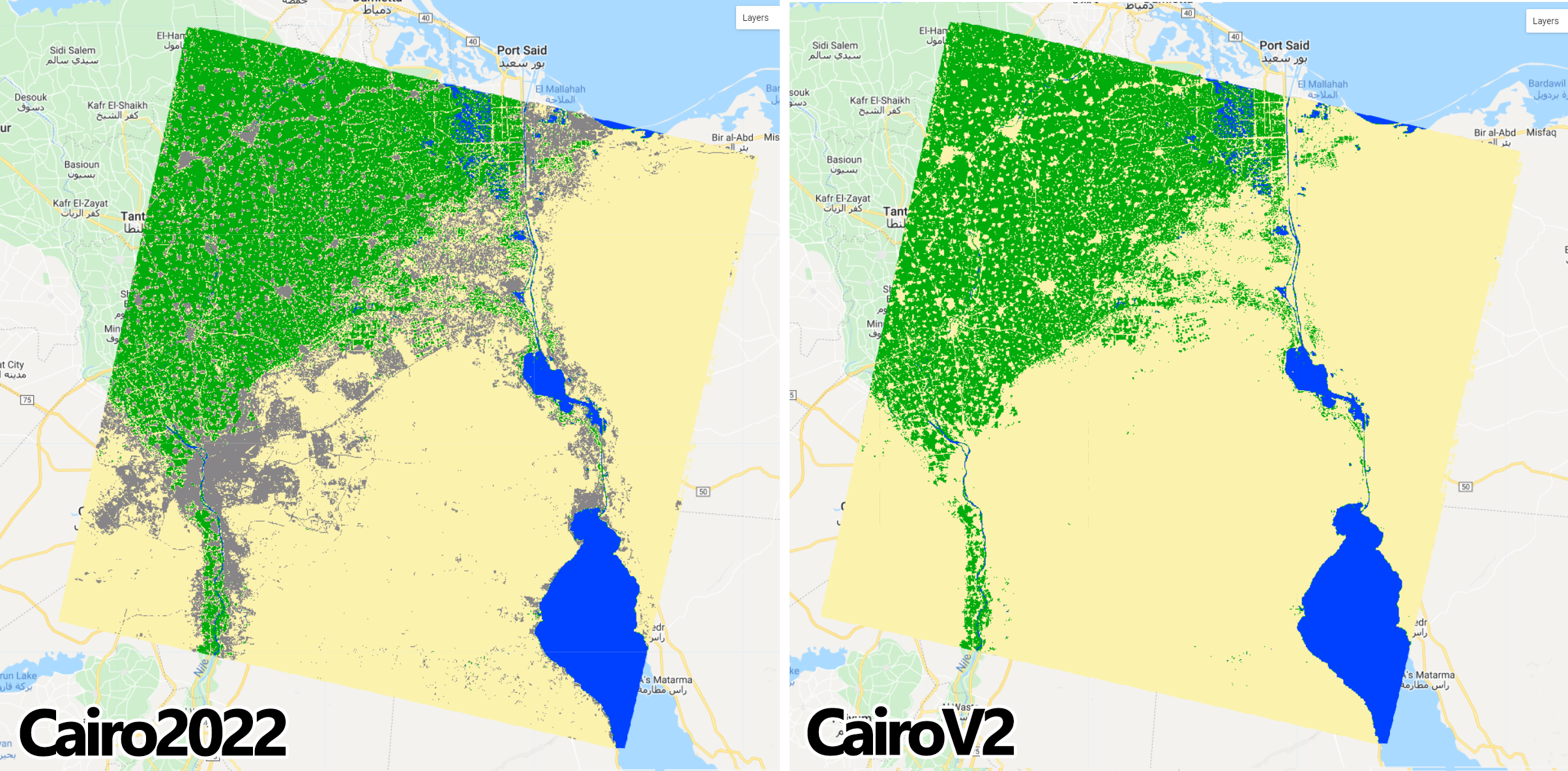
Figure 2.33: Cairo2022
and CairoV2
maps using the original paletteMap
. Note that Sand and bare areas and Built-up and Urban areas have the same color as they were merged under the same pixel value (2).
*Scenario 2
A new version of the Cairo2022
map where only a portion of it is remapped to a given class.
This scenario is one of the most commonly used post-classification procedure where the goal is to remap specific areas to fix for classification errors. To ilustrate, we will consider the following example:
Upon close inspection, the Cairo2022
map showed some cropland/cultivated areas within zones of dense urbanization of New Cairo City. A team on the ground went to the area and confirmed that is indeed dense urbanization and no agricultural land was found in that area. Therefore, that portion of the map needs to be rectified in order to reflect the actual land cover. In this case, the .where()
method of an ee.Image()
object is used. This function performs conditional replacement of values, following the formula input.where(test,value)
. For each pixel in each band of input
, if the corresponding pixel in test
is nonzero, Google Earth Engine will output the corresponding pixel in value
, otherwise it will output the input
pixel. Translating this function to this example, input
is the land cover map in which we aim to perform the reclassification - in this case Cairo2020
; test
is the area or region of the map value
will take place, and; value
is the correct classification that will be included in the final map:

Figure 2.34: The .where
function applied to this example.
In your own version of Cairo2022
map, create an new geometry over an area of your interest and name it aoi
:
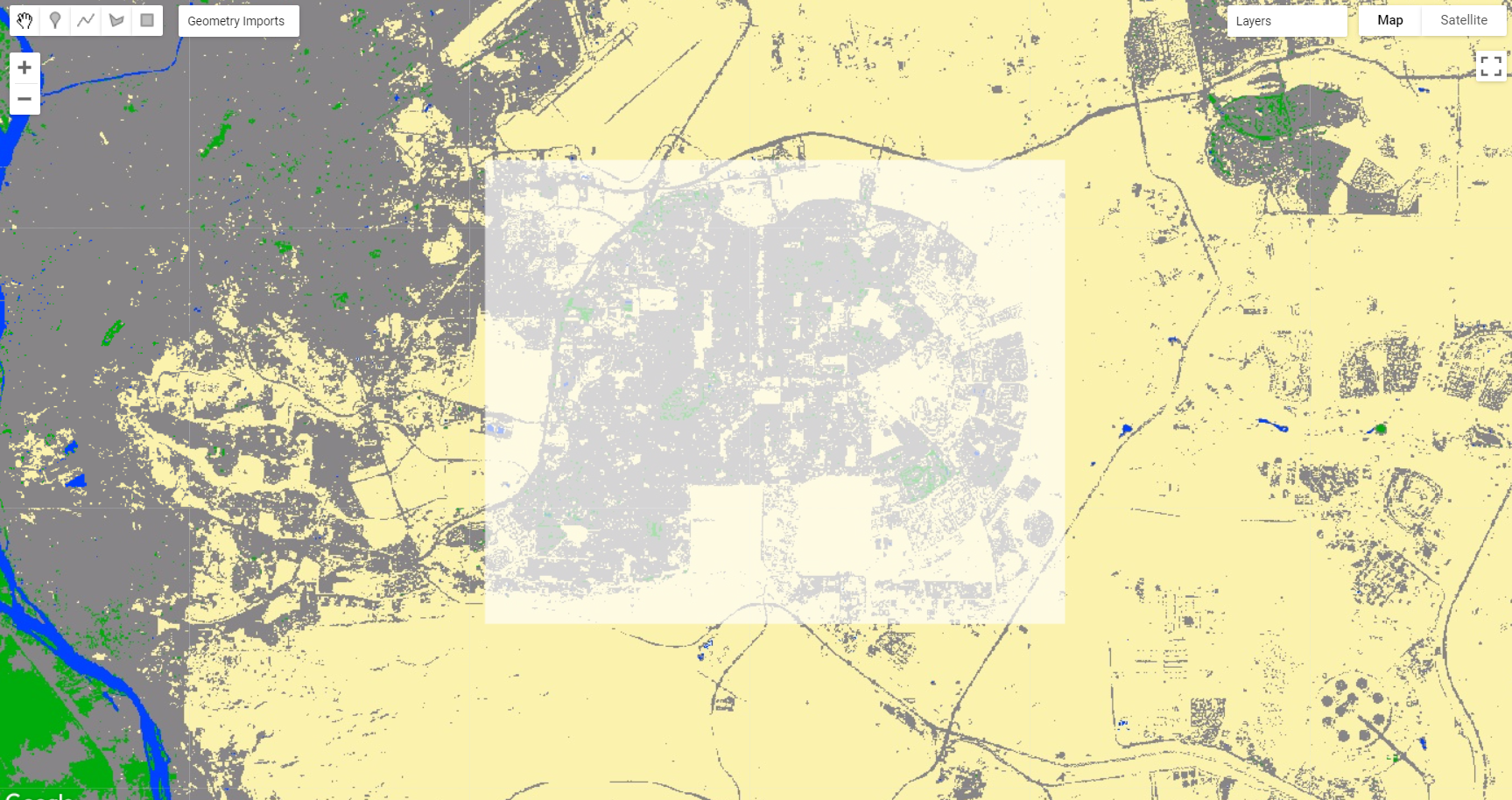
Figure 2.35: A geometry over an area of Cairo2020
highlighting cultivated areas next to urbanization.
The method .where()
only uses ee.Image()
objects as test
. Therefore, using the geometry/feature aoi
is not allowed.
An easy and effective way to go around this rule is to create an image with ee.Image()
and clip it for the region of interest:
The function above will create an image with value of 1 and will clip it for the area of interest aoi
. Next, using the same approach from Scenario 1 and 2, we will create a new version of Cairo2022
called ‘’subistitute’, where the Cropland/Cultivated Areas class is remapped to Built-up and Urban Areas: the position 2 on list 2 (belonging to agricultural/cultivated areas) receives the value 3 from Built-up and Urban Areas on list 1:
Now, using .where()
we will create a new map CairoV3
following the formula (2.34) and add it to the map editor:
var CairoV3 = Cairo2022.where(region,substitute);
Map.addLayer(CairoV3, {min: 0, max: 3, palette:paletteMAP}, 'Cairo V3');
Compare the two classification objects: you will notice that every pixel of Cropland/Cultivated Areas (green, pixel value = 2) within the aoi
in Cairo2022
is now remapped to Built-up and Urban Areas (grey, pixel value = 3) in CairoV3
:
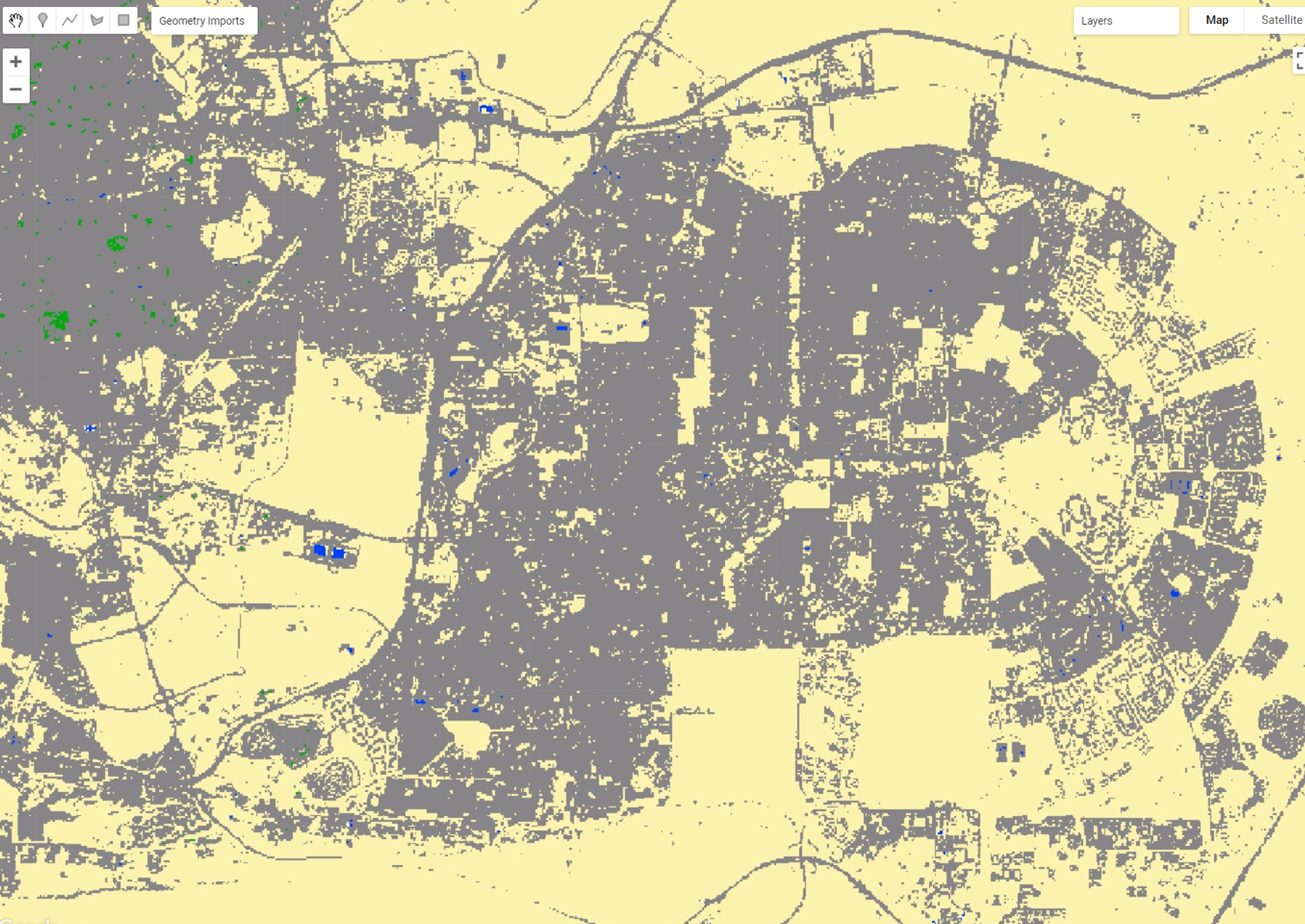
Figure 2.36: CairoV3
showing the pixels of Cropland/Cultivated Areas remapped as Built-up and Urban Areas within aoi
.
2.3.2 Map spatial smoothing
Spatial smoothing is another post-classification procedure to enhance the quality of a land cover classification output. A common issue that arises from the pixel-based classification of fine/medium spatial resolution imagery is the “salt-and-pepper” effect. This happens when individual pixels are classified differently from their neighbors, creating speckles of isolated pixels of different classes. There are several ways to minimize this issue, such as:
image pre-processing, such as low-pass filter and texture analysis;
contextual classification, and;
post-classification processing, such as median and mode filtering.
In this section, we will focus on a post-classification technique to reduce the salt and pepper effect and edge roughness of land cover maps using focal median filtering (ee.Image.focal_median()
).
We will apply the .focal_median()
method to Cairo2022
. This method is a simple sliding-window spatial filter that replaces the center value (or class) in the window with the median of all the pixel values in the window. The window, or kernel, is usually square but can be any shape. The .focal_median()
method usually is expressed as:

Figure 2.37: .focal_median
spatial filter.
In the function above, the radius
parameter specifies the number of pixels from the center that the kernel will cover. This radius value can be expressed as number of pixels or meters. The kernelType
specifies the type of kernel to use.
Either .focal_median(1, ‘square’, ‘pixels’)
or
.focal_median(30, ‘square’, ‘meters’)
will produce a 3x3
pixel sliding-window, as you are specifing 1 pixel (or 30 meters which
is equal to one Landsat pixel) in each direction from the center pixel.
Similarly, a radius
of 2 pixels or 60 meters will produce a
5x5 sliding-window: a center pixel plus 2 pixels in each direction.
Following Figure 2.37 and the example above, we will apply the .focal_median()
filtering with two radius
sizes (30 and 60 meters) to Cairo2022
, add Cairo30
and Cairo60
to the map editor and compare the results:
var Cairo30 = Cairo2022.focal_median(30,'square', 'meters');
var Cairo60 = Cairo2022.focal_median(60,'square', 'meters');
Map.addLayer(Cairo30, {min: 0, max: 3, palette:paletteMAP}, 'Cairo 3x3');
Map.addLayer(Cairo60, {min: 0, max: 3, palette:paletteMAP}, 'Cairo 5x5');
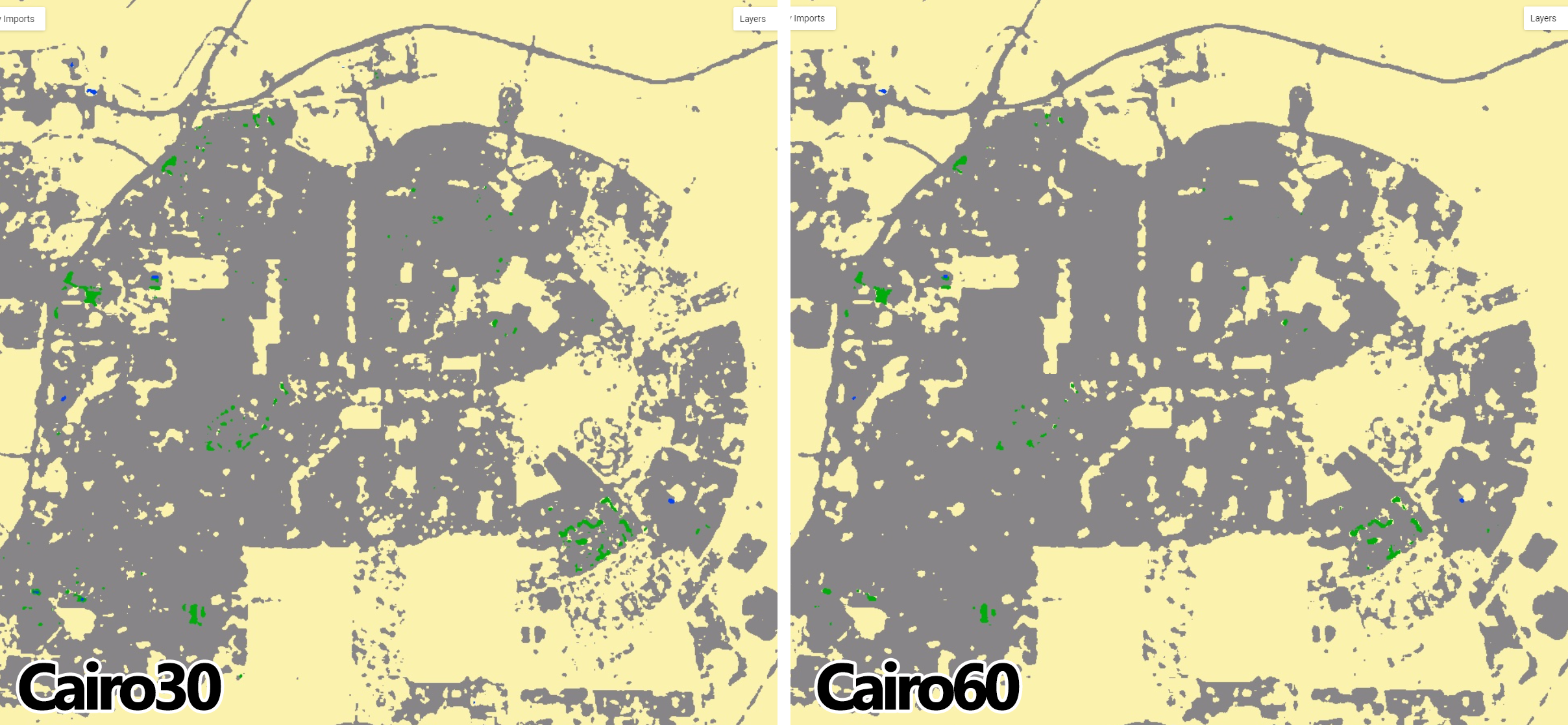
Figure 2.38: Cairo2022
processed with .focal_median
spatial filter two radius sizes.
IMPORTANT: The
.focal_median(1, ‘square’, ‘pixels’)
* and
.focal_median(30, ‘square’, ‘meters’)
will only achieve the
intended final result if you reproject it back to the original scale and
projection from the original map. Note that this technique while
effective in removing the “salt-and-pepper” effect from the original
map, it creates edges that are not in the same resolution and projection
as the original map. Therefore, creating fairly rounded boundaries for
the classes. This is not ideal as it is usually intended for these maps
to keep the same resolution as the original map, as well as to preserve
the boundaries between classes. To account for this issue, you can
reproject this output back the original scale so it is formed by 30 x 30
meter pixels. To do that, simply use
.reproject(projection.atScale(scale))
within the
.focal_median
filter. The .reproject
function
will take two arguments: projection and scale. You can extract this
information from the original map with .projection()
and
.nominalScale()
:
Then, we re-aply the .focal_median()
filter with .reproject(projection.atScale(scale))
:
var newCairo30 = Cairo2022.focal_median(1,'square', 'pixels')
.reproject(projection.atScale(scale));
var newCairo60 = Cairo2022.focal_median(60,'square', 'meters')
.reproject(prj.atScale(scale));
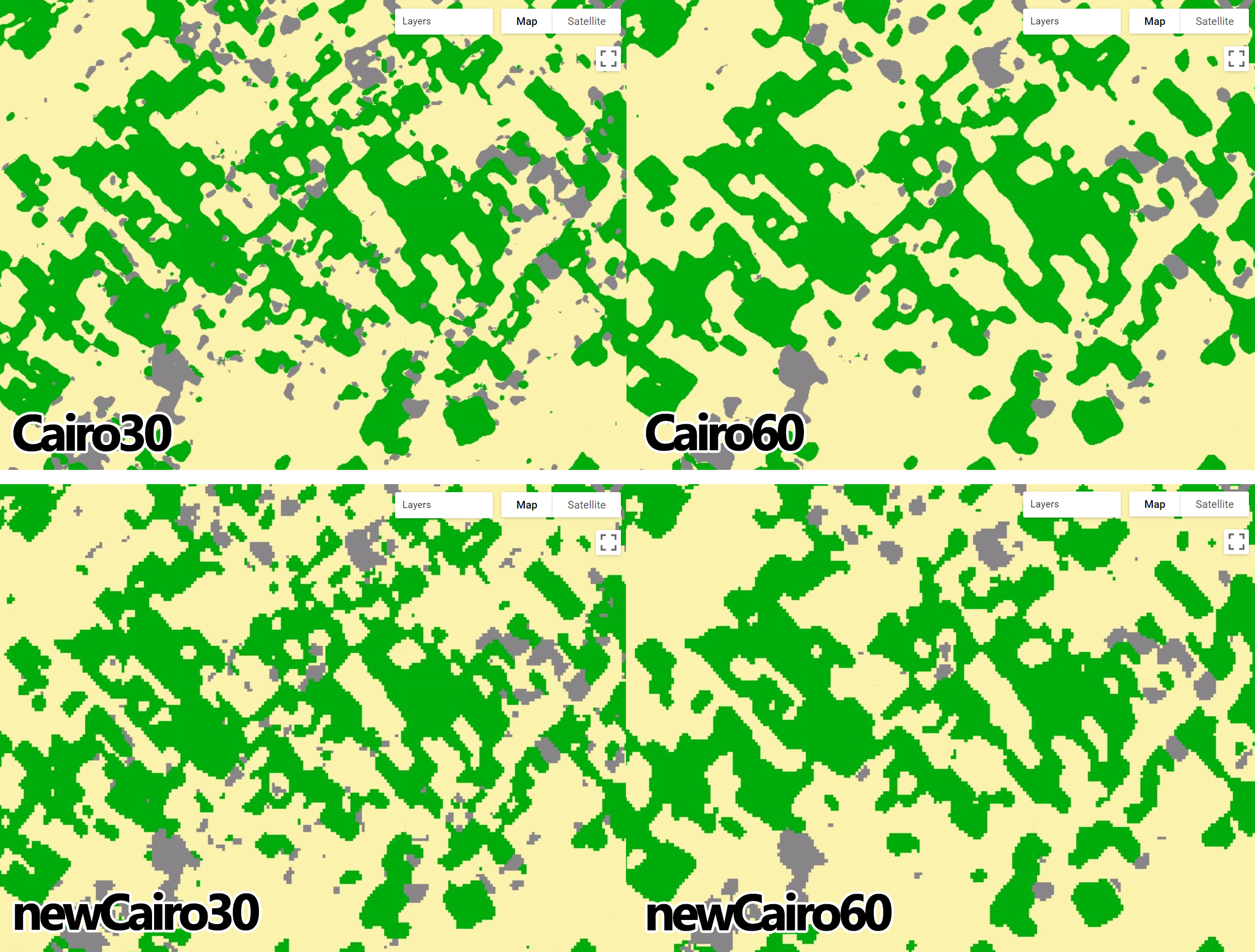
Figure 2.39: Cairo2022
processed with .focal_median
spatial filter with 3x3 and 5x5 pixel windows. Note that the function was reprojected to match Cairo2022
’s projection and scale. Now the discrete boundaries of classes are pixelated back to 30 meters.
Note that even though the boundaries of the classes are smoother, they are formed by the 30 x 30 meter pixels.
Usually, a radius
of 1 (3x3 square window) removes most of the salt-and-pepper effect, smoothens the boundaries in between classes while preserving the overall shape of the classes.
Access the full scrip for this section here.
You have the option to classify the entire scene or clip it to an area of interest. You can create a geometry
AreaOfInterest
and clip your composite using.clip(AreaOfInterest)
. You can also customize the composite visualization parameters by clicking on the settings icon (gear ⚙) next to the layer name (in this example: ‘Composite Median’)